Hyperspectral Image Reconstruction Using Hierarchical Neural Architecture Search from A Snapshot Image
ICASSP 2024 - 2024 IEEE International Conference on Acoustics, Speech and Signal Processing (ICASSP)(2024)
摘要
Hyperspectral imaging is a promising imaging modality, and has attracted increasing research attention by compressive sensing such as coded aperture snapshot spectral imaging (CASSI), for simultaneously capturing abundant information in spatial, spectral and temporal domains. Hyperspectral image (HSI) reconstruction in the CASSI aims to retrieve the original 3D signal upon the 2D compressed snapshot. Recently, deep learning has extensively been employed for HSI reconstruction via manually designing network architectures, and usually causes complicated and massive-computational models, which are difficult for being embedding in the real imaging systems. This study aims to leverage network architecture search to automatically design effective and efficient network architectures for HSI reconstruction. Specifically, we exploit gradient-based search strategies and prepare optional operations (cells) with adaptive receptive field such as dilate and deformable convolutional layers to construct a flexible hierarchical search space. Through sharing cells within different levels of features and utilizing an early stopping technique, we achieve a computational and memory efficient NAS strategy to automatically design an effective lightweight model for HSI reconstruction. Extensive experimental results have demonstrated that the network architecture achieved by our proposed NAS has much smaller model size and a lower computational cost while produce better or comparable HSI reconstruction performance compared with the state-of-the-art methods.
更多查看译文
关键词
Hyperspectral image reconstruction,Net-work architecture search,Gradient-based search,Hierarchical search space,Lightweight model
AI 理解论文
溯源树
样例
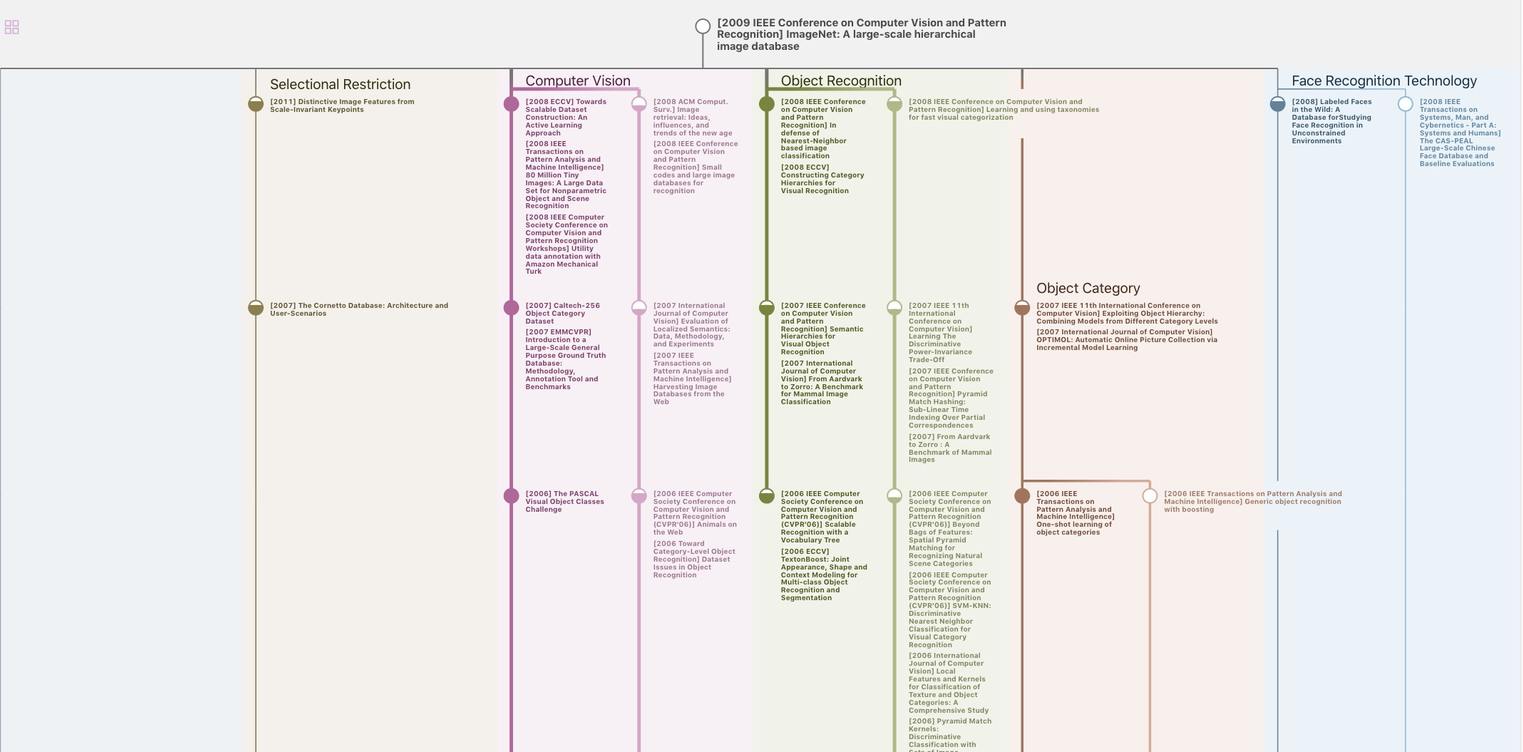
生成溯源树,研究论文发展脉络
Chat Paper
正在生成论文摘要