Dynamic Mutual-Activated Transformer for Human Motion Prediction
ICASSP 2024 - 2024 IEEE International Conference on Acoustics, Speech and Signal Processing (ICASSP)(2024)
摘要
Accurate human motion prediction is vital for diverse artificial intelligence applications, and recent research has yielded substantial advancements. Despite this, the prediction process often encounters abrupt discontinuities and accumulates errors over the long term due to insufficient modeling of spatial and temporal correlations, which significantly impacts predictive accuracy. To tackle these challenges, we introduce the Dynamic Mutual-Activated Transformer (DyMAT). This innovative approach learns spatial correlation among joints in pose and temporal correlation of each joint. It is achieved through separate yet concurrent Pose-wise Spatial Attention (PSA) and Joint-specific Temporal Attention (JTA). The dynamic mutual-activation block (DMA) adeptly combines spatio-temporal features, significantly enhancing DyMAT’s representational capacity. Moreover, we integrate a Temporal Self-Enhancement (TSE) block with JTA, serving as a supplement for refining temporal correlation learning. Our experiments conducted on Human3.6M and CMU Mocap datasets underscore that DyMAT consistently outperforms state-of-the-art methods in terms of prediction accuracy. Code is available at https://github.com/alanzhangv123/DyMAT.
更多查看译文
关键词
Human Motion Prediction,Dynamic Mutual-Activated Transformer,Temporal Self-Enhancement
AI 理解论文
溯源树
样例
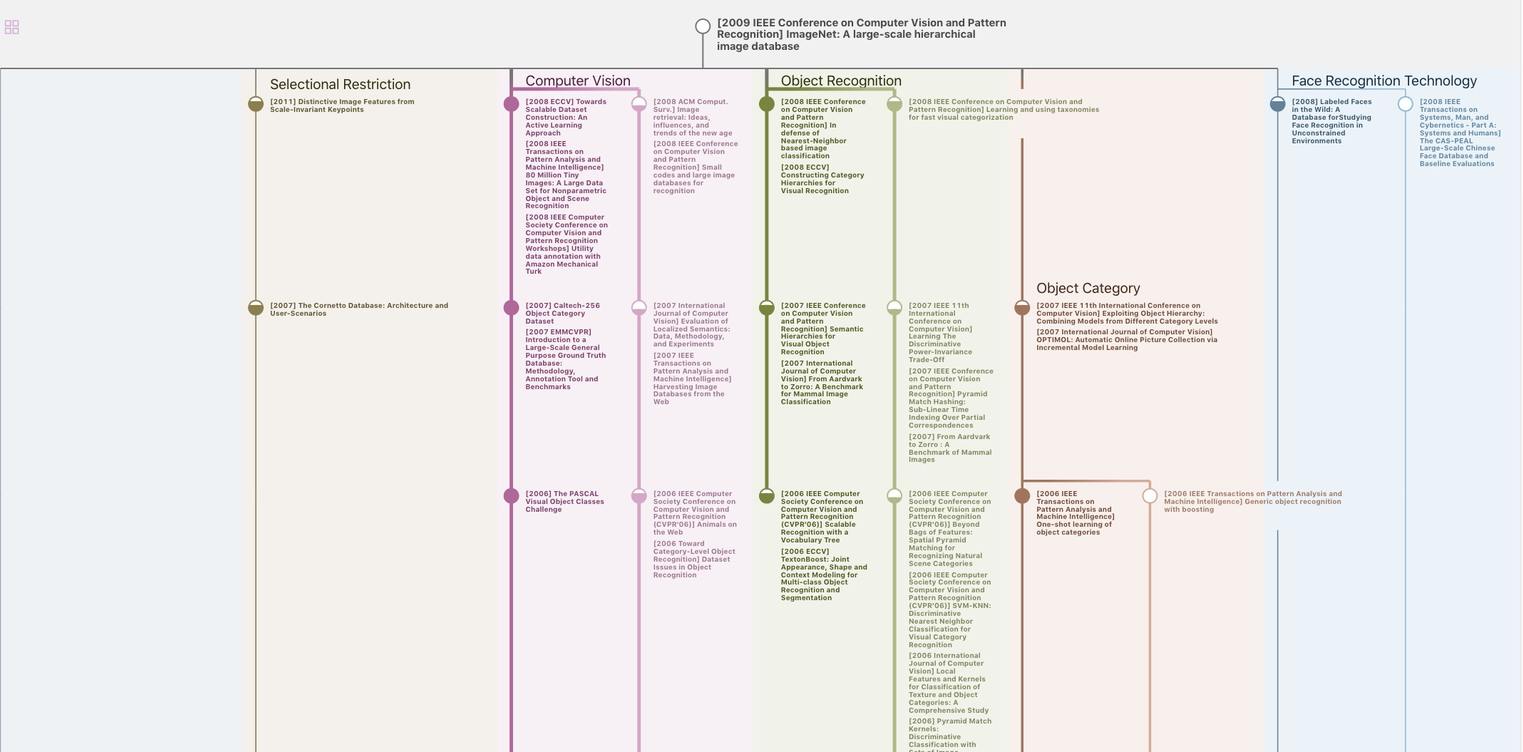
生成溯源树,研究论文发展脉络
Chat Paper
正在生成论文摘要