SR-HuBERT : An Efficient Pre-Trained Model for Speaker Verification
ICASSP 2024 - 2024 IEEE International Conference on Acoustics, Speech and Signal Processing (ICASSP)(2024)
摘要
Recently, pre-trained models (PTMs) have been extensively applied in speaker verification (SV) and greatly boosted system performance. However, mainstream PTMs currently concentrate on using frame-level universal representations. In this paper, we propose a novel pre-training framework that jointly models speaker information — Speaker Related HuBERT, abbreviated as SR-HuBERT. This framework aims to further explore speaker-related information inherent in speech universal representations. The proposed SR-HuBERT utilizes an unsupervised clustering algorithm based on graph structures to generate speaker pseudo-labels and promotes the learning of segment-level speaker-related representations through a multi-task pre-training framework. Experimental results on VoxCeleb1 test set demonstrate the effectiveness of the proposed SR-HuBERT. Even in the scenarios of limited fine-tuning data, SR-HuBERT outperforms the other existing PTMs on SV tasks. Additionally, SR-HuBERT also performs well on speaker-related tasks of SUPERB benchmark.
更多查看译文
关键词
speaker verification,pre-trained model,clustering,fine-tuning
AI 理解论文
溯源树
样例
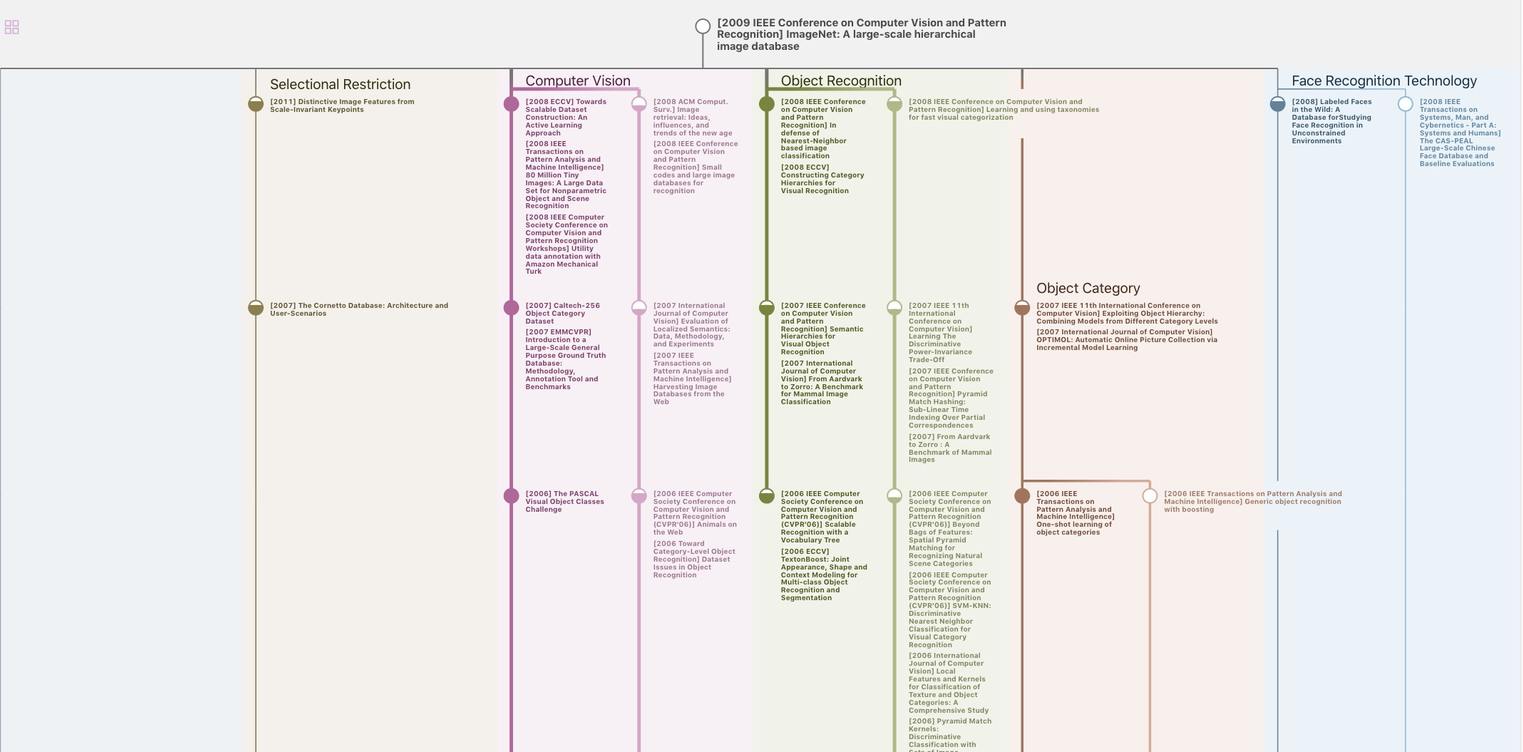
生成溯源树,研究论文发展脉络
Chat Paper
正在生成论文摘要