Span-Based Optimal Sample Complexity for Weakly Communicating and General Average Reward MDPs
arxiv(2024)
摘要
We study the sample complexity of learning an ϵ-optimal policy in an
average-reward Markov decision process (MDP) under a generative model. For
weakly communicating MDPs, we establish the complexity bound
Õ(SAH/ϵ^2), where H is the span of the bias function
of the optimal policy and SA is the cardinality of the state-action space.
Our result is the first that is minimax optimal (up to log factors) in all
parameters S,A,H and ϵ, improving on existing work that either
assumes uniformly bounded mixing times for all policies or has suboptimal
dependence on the parameters. We further investigate sample complexity in
general (non-weakly-communicating) average-reward MDPs. We argue a new
transient time parameter B is necessary, establish an
Õ(SAB+H/ϵ^2) complexity bound, and prove a matching
(up to log factors) minimax lower bound. Both results are based on reducing the
average-reward MDP to a discounted MDP, which requires new ideas in the general
setting. To establish the optimality of this reduction, we develop improved
bounds for γ-discounted MDPs, showing that
Ω̃(SAH/(1-γ)^2ϵ^2) samples suffice
to learn an ϵ-optimal policy in weakly communicating MDPs under the
regime that γ≥ 1-1/H, and
Ω̃(SAB+H/(1-γ)^2ϵ^2) samples
suffice in general MDPs when γ≥ 1-1/B+H. Both these results
circumvent the well-known lower bound of
Ω̃(SA1/(1-γ)^3ϵ^2) for arbitrary
γ-discounted MDPs. Our analysis develops upper bounds on certain
instance-dependent variance parameters in terms of the span and transient time
parameters. The weakly communicating bounds are tighter than those based on the
mixing time or diameter of the MDP and may be of broader use.
更多查看译文
AI 理解论文
溯源树
样例
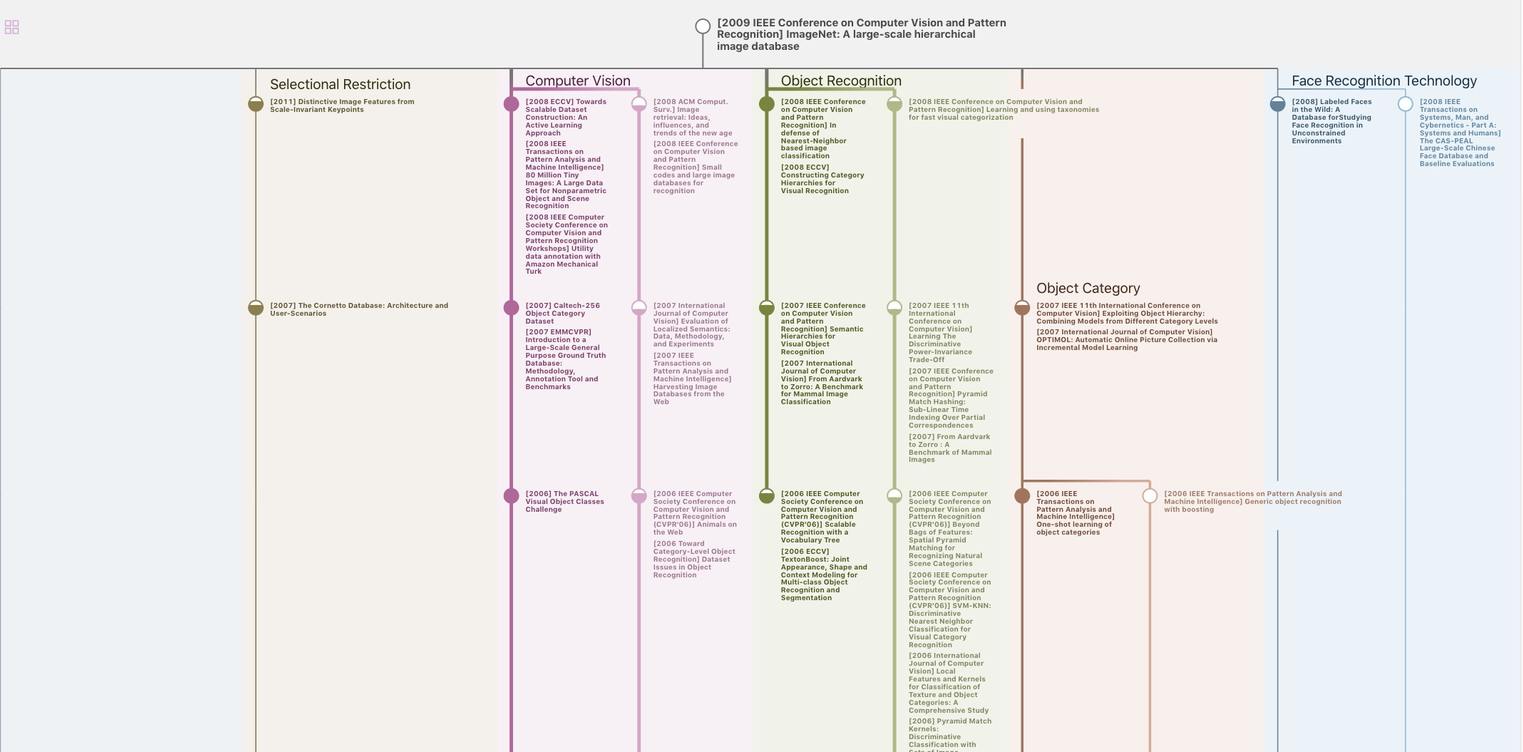
生成溯源树,研究论文发展脉络
Chat Paper
正在生成论文摘要