ALDM-Grasping: Diffusion-aided Zero-Shot Sim-to-Real Transfer for Robot Grasping
CoRR(2024)
摘要
To tackle the "reality gap" encountered in Sim-to-Real transfer, this study
proposes a diffusion-based framework that minimizes inconsistencies in grasping
actions between the simulation settings and realistic environments. The process
begins by training an adversarial supervision layout-to-image diffusion
model(ALDM). Then, leverage the ALDM approach to enhance the simulation
environment, rendering it with photorealistic fidelity, thereby optimizing
robotic grasp task training. Experimental results indicate this framework
outperforms existing models in both success rates and adaptability to new
environments through improvements in the accuracy and reliability of visual
grasping actions under a variety of conditions. Specifically, it achieves a
75% success rate in grasping tasks under plain backgrounds and maintains a
65% success rate in more complex scenarios. This performance demonstrates this
framework excels at generating controlled image content based on text
descriptions, identifying object grasp points, and demonstrating zero-shot
learning in complex, unseen scenarios.
更多查看译文
AI 理解论文
溯源树
样例
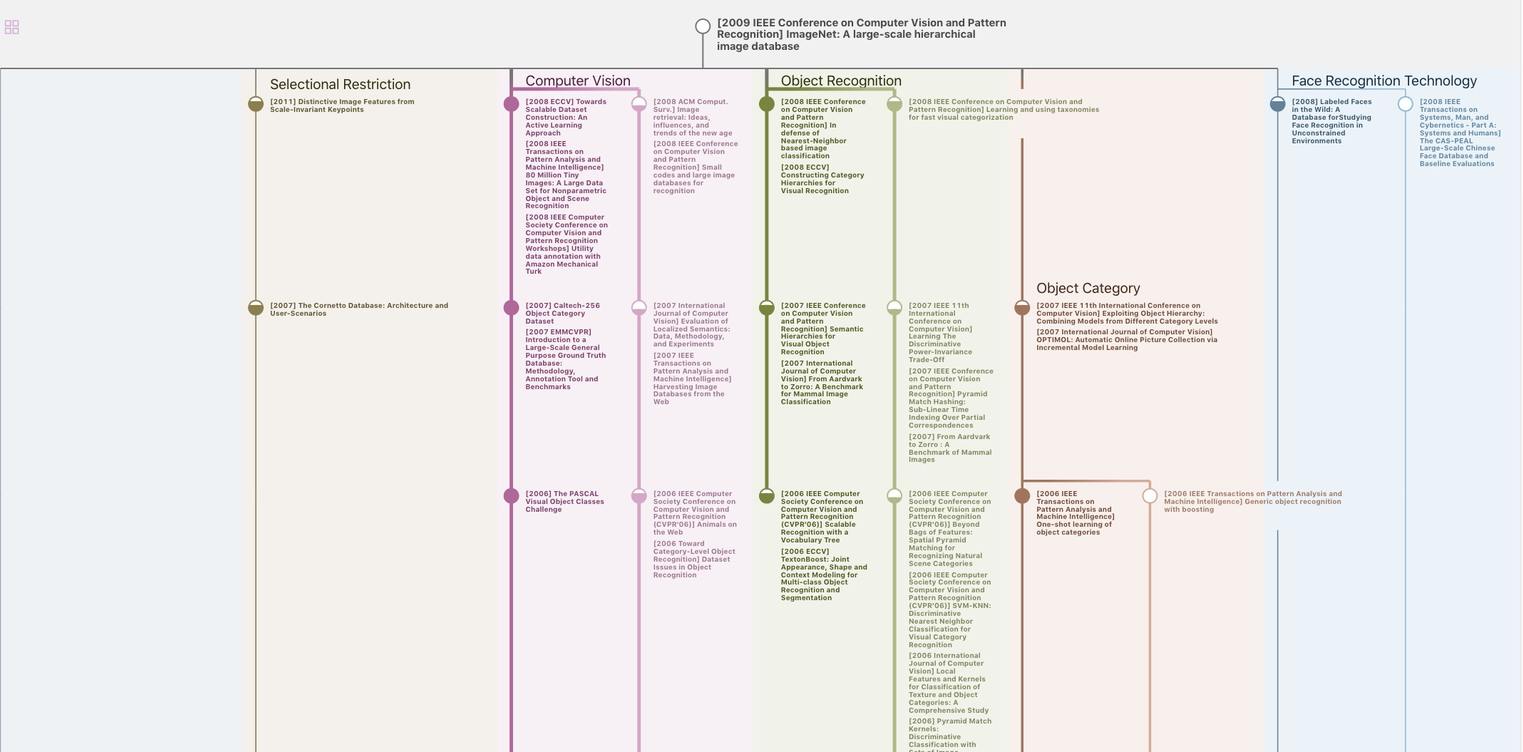
生成溯源树,研究论文发展脉络
Chat Paper
正在生成论文摘要