Phonon predictions with E(3)-equivariant graph neural networks
arxiv(2024)
摘要
We present an equivariant neural network for predicting vibrational and
phonon modes of molecules and periodic crystals, respectively. These
predictions are made by evaluating the second derivative Hessian matrices of
the learned energy model that is trained with the energy and force data. Using
this method, we are able to efficiently predict phonon dispersion and the
density of states for inorganic crystal materials. For molecules, we also
derive the symmetry constraints for IR/Raman active modes by analyzing the
phonon mode irreducible representations. Additionally, we demonstrate that
using Hessian as a new type of higher-order training data improves energy
models beyond models that only use lower-order energy and force data. With this
second derivative approach, one can directly relate the energy models to the
experimental observations for the vibrational properties. This approach further
connects to a broader class of physical observables with a generalized energy
model that includes external fields.
更多查看译文
AI 理解论文
溯源树
样例
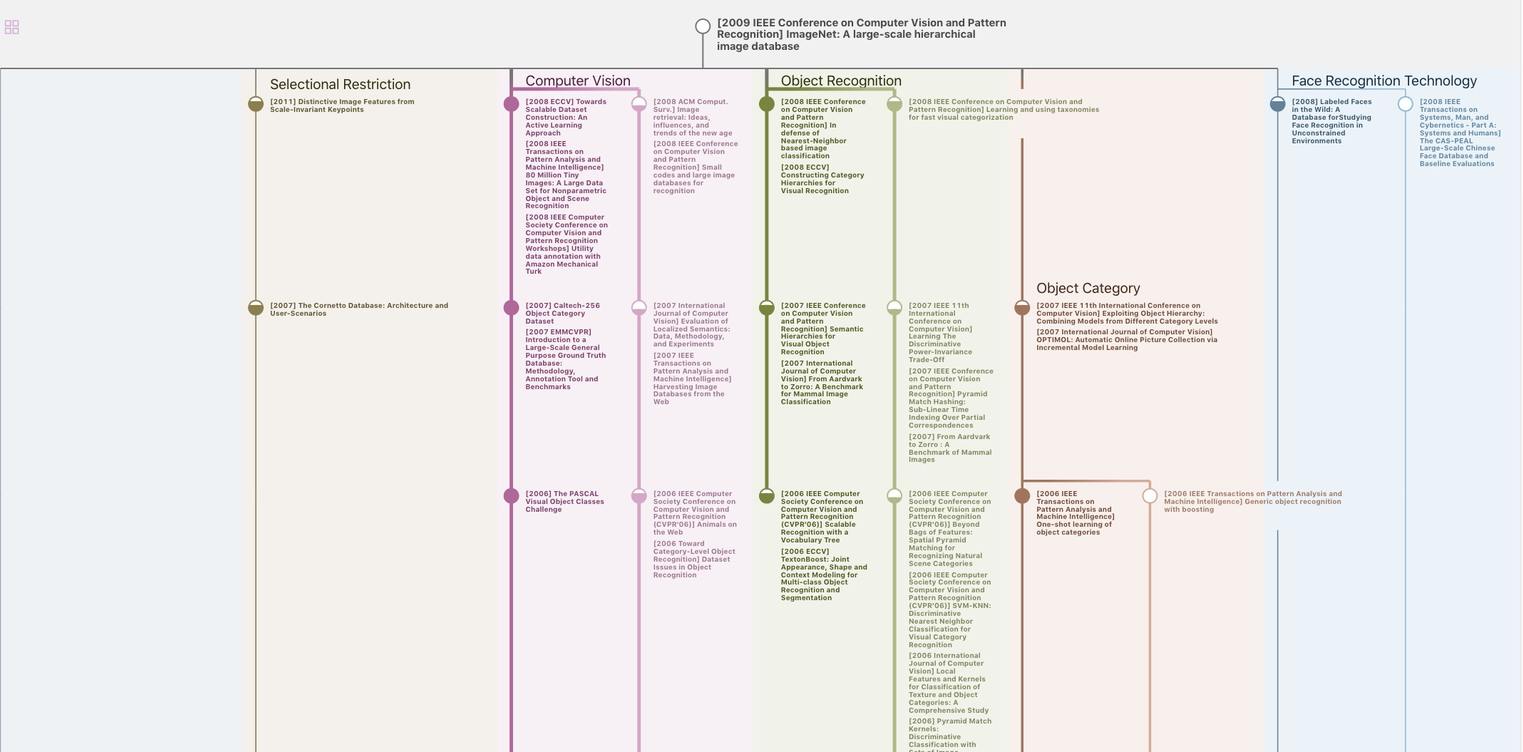
生成溯源树,研究论文发展脉络
Chat Paper
正在生成论文摘要