Artifact Feature Purification for Cross-domain Detection of AI-generated Images
arxiv(2024)
摘要
In the era of AIGC, the fast development of visual content generation
technologies, such as diffusion models, bring potential security risks to our
society. Existing generated image detection methods suffer from performance
drop when faced with out-of-domain generators and image scenes. To relieve this
problem, we propose Artifact Purification Network (APN) to facilitate the
artifact extraction from generated images through the explicit and implicit
purification processes. For the explicit one, a suspicious frequency-band
proposal method and a spatial feature decomposition method are proposed to
extract artifact-related features. For the implicit one, a training strategy
based on mutual information estimation is proposed to further purify the
artifact-related features. Experiments show that for cross-generator detection,
the average accuracy of APN is 5.6
on GenImage dataset and 1.7
cross-scene detection, APN maintains its high performance. Via visualization
analysis, we find that the proposed method extracts flexible forgery patterns
and condenses the forgery information diluted in irrelevant features. We also
find that the artifact features APN focuses on across generators and scenes are
global and diverse. The code will be available on GitHub.
更多查看译文
AI 理解论文
溯源树
样例
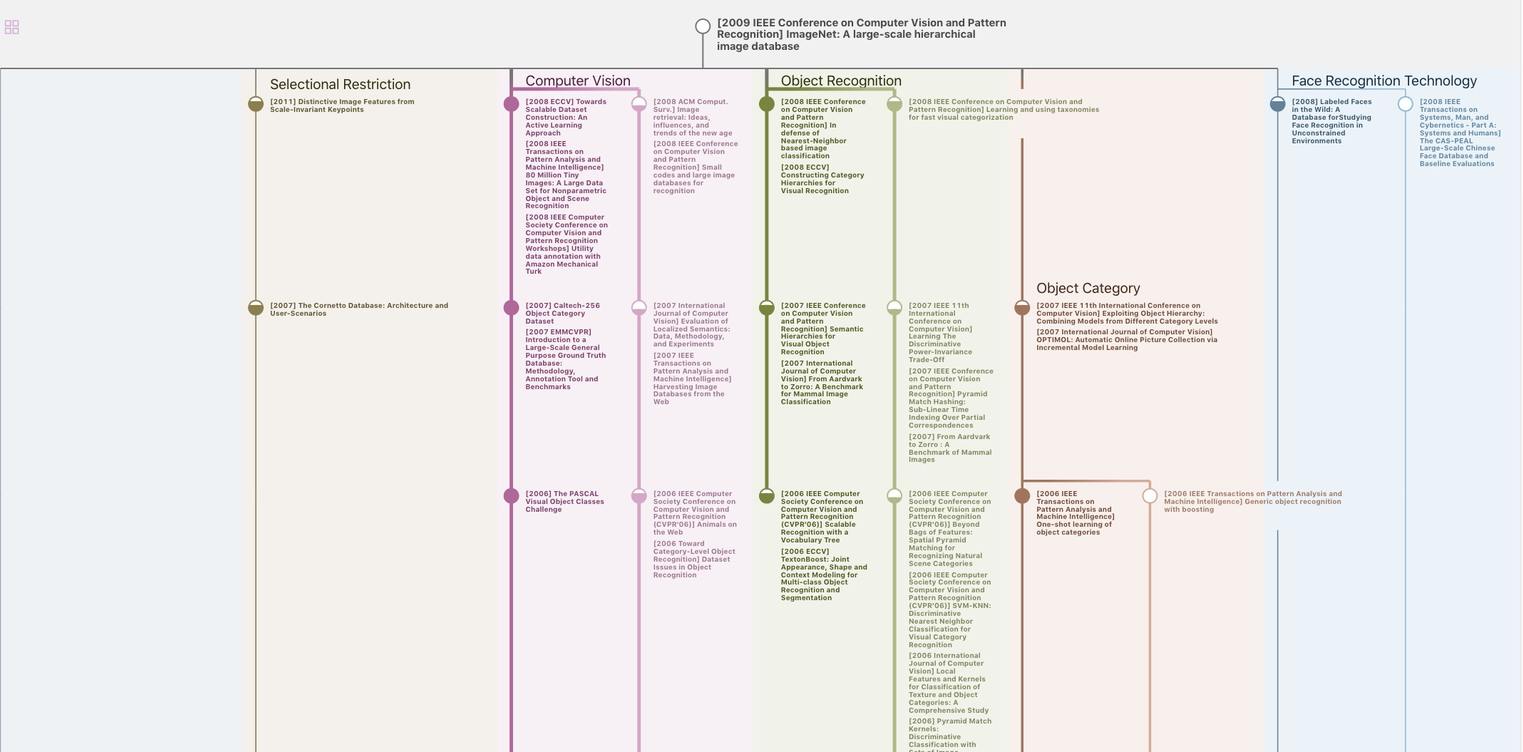
生成溯源树,研究论文发展脉络
Chat Paper
正在生成论文摘要