Quality-Aware Dynamic Resolution Adaptation Framework for Adaptive Video Streaming
Proceedings of the ACM Multimedia Systems Conference 2024 on ZZZ(2024)
摘要
Traditional per-title encoding schemes aim to optimize encoding resolutions
to deliver the highest perceptual quality for each representation. XPSNR is
observed to correlate better with the subjective quality of VVC-coded
bitstreams. Towards this realization, we predict the average XPSNR of VVC-coded
bitstreams using spatiotemporal complexity features of the video and the target
encoding configuration using an XGBoost-based model. Based on the predicted
XPSNR scores, we introduce a Quality-A ware Dynamic Resolution Adaptation
(QADRA) framework for adaptive video streaming applications, where we determine
the convex-hull online. Furthermore, keeping the encoding and decoding times
within an acceptable threshold is mandatory for smooth and energy-efficient
streaming. Hence, QADRA determines the encoding resolution and quantization
parameter (QP) for each target bitrate by maximizing XPSNR while constraining
the maximum encoding and/ or decoding time below a threshold. QADRA implements
a JND-based representation elimination algorithm to remove perceptually
redundant representations from the bitrate ladder. QADRA is an open-source
Python-based framework published under the GNU GPLv3 license. Github:
https://github.com/PhoenixVideo/QADRA Online documentation:
https://phoenixvideo.github.io/QADRA/
更多查看译文
AI 理解论文
溯源树
样例
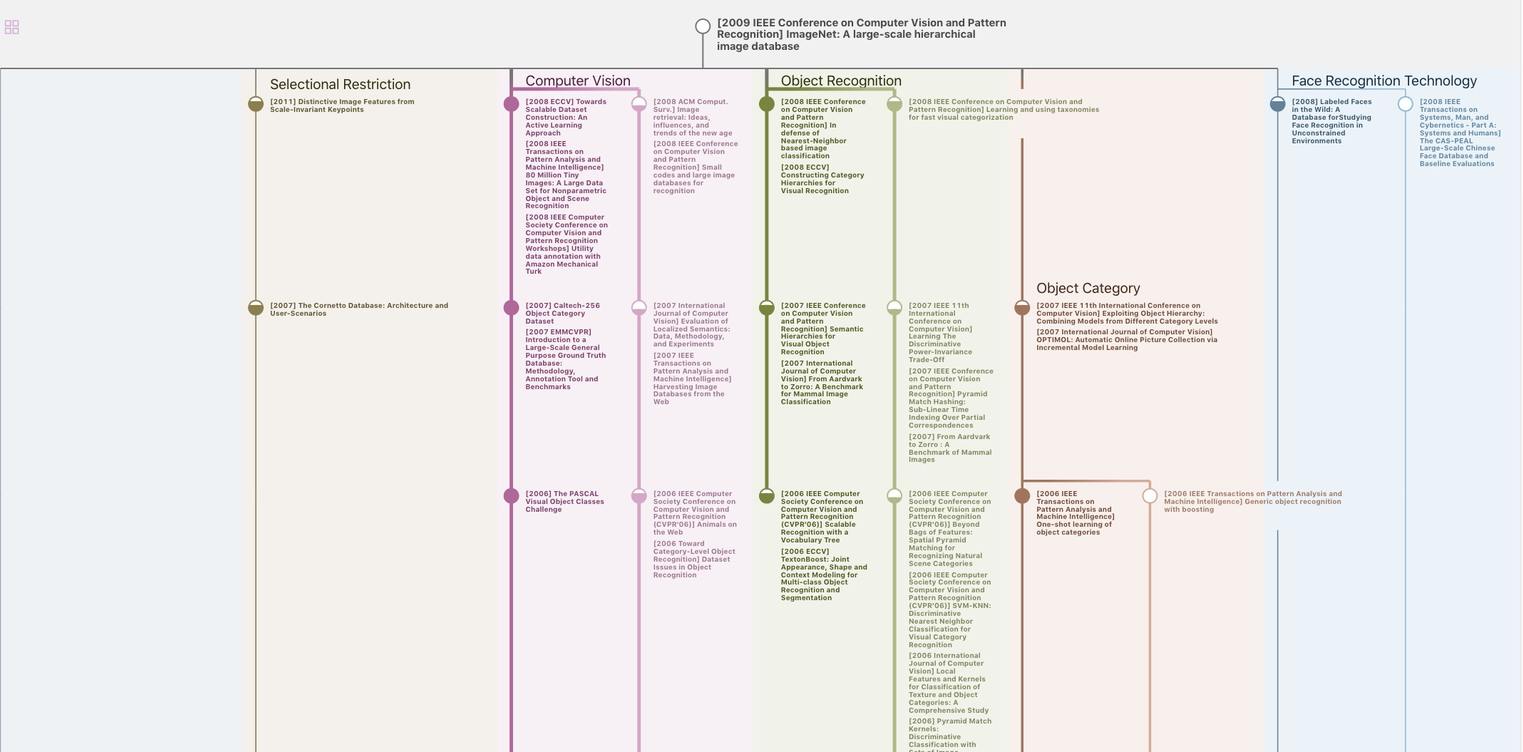
生成溯源树,研究论文发展脉络
Chat Paper
正在生成论文摘要