A novel QCT-based deep transfer learning approach for predicting stiffness tensor of trabecular bone cubes
IRBM(2024)
摘要
Objectives
This study was performed to prove the concept that transfer learning techniques, assisted with a generative model, could be used to alleviate the ‘big data’ requirement for training high-fidelity deep learning (DL) models in prediction of stiffness tensor of trabecular bone cubes.
Material and methods
Transfer learning approaches of domain adaptation were used, in which a source domain included 1,641 digital trabecular bone cubes synthesized from a generative model, and a target domain included 868 real trabecular bone cubes from human cadaver femurs. Simulated quantitative computed tomography (QCT) images of both the synthesized and real bone cubes were used as input, whereas the stiffness tensor of these cubes determined using finite element simulations were used as output. Three transfer learning algorithms, including instance-based (TrAdaBoostR2 and WANN) and parameter-based (RNN) methods, were used. Two case studies, one with varying sizes of training dataset and the other with a gender-biased training dataset, were performed to evaluate these deep transfer learning models in comparison with a base deep learning (DL) model trained using the dataset from the target domain.
Results
The results indicated that these deep transfer learning models were robust both to sample size and to the gender-biased training dataset, whereas the base DL model was very sensitive to such changes. Among the three transfer learning algorithms, the prediction accuracy of the RNN-based deep transfer learning model was the best (0.92-0.96%) and comparable to that of the base DL model trained using the dataset from the target domain.
Conclusion
This study proved the proposed concept and confirmed that high fidelity QCT-based deep learning models could be obtained for prediction of stiffness tensor of trabecular bone cubes.
更多查看译文
关键词
Trabecular Bone,Deep transfer learning,QCT image,Stiffness tensor,Synthesized digital bone samples,FEM
AI 理解论文
溯源树
样例
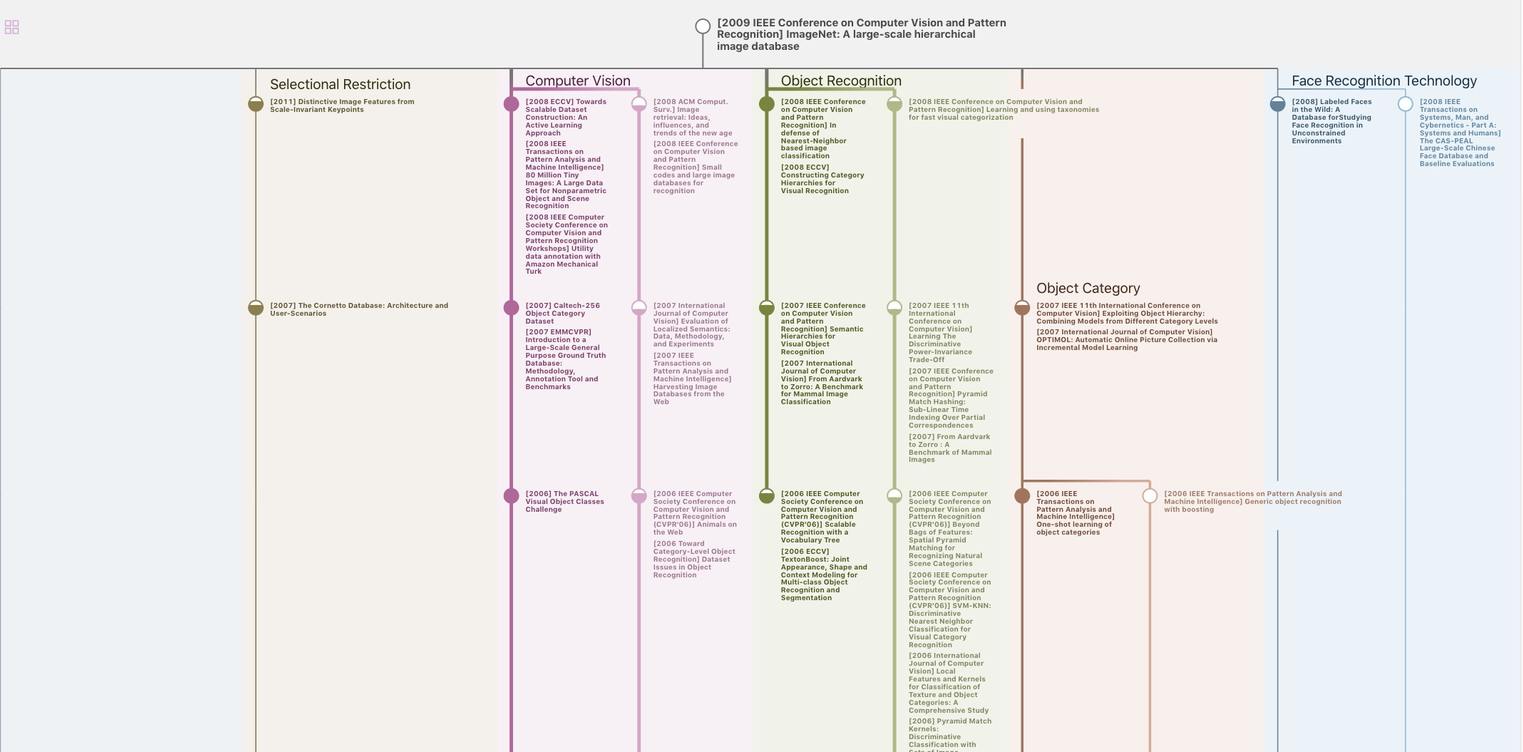
生成溯源树,研究论文发展脉络
Chat Paper
正在生成论文摘要