Direct Position Determination by Covariance-Fitting on the Riemannian Manifold of Hermitian Positive Definite Matrices
ICASSP 2024 - 2024 IEEE International Conference on Acoustics, Speech and Signal Processing (ICASSP)(2024)
摘要
Direct Position Determination (DPD) is the state-of-the-art solution for emitter localization using multiple phased arrays. This paper shows that DPD can be recast as a covariance-fitting (CF) problem that minimizes the Euclidean distance between a sample covariance matrix ${\mathbf{\hat R}}$ and its location-dependent model R. By showing equivalence to existing DPD methods, this CF viewpoint highlights that the geometry of the Hermitian Positive Definite (HPD) covariance matrices R and ${\mathbf{\hat R}}$ is simply overlooked. Based on this critical observation, we propose a new CF approach for DPD that specifically exploits the Riemannian geometry of HPD matrices for measuring the distance between R and ${\mathbf{\hat R}}$. Experimental results showcase that the proposed Riemannian CF approach for DPD leads to a significant improvement in localization accuracy.
更多查看译文
关键词
Emitter localization,covariance-fitting,Riemannian geometry,Hermitian positive definite manifold,affine-invariant metric
AI 理解论文
溯源树
样例
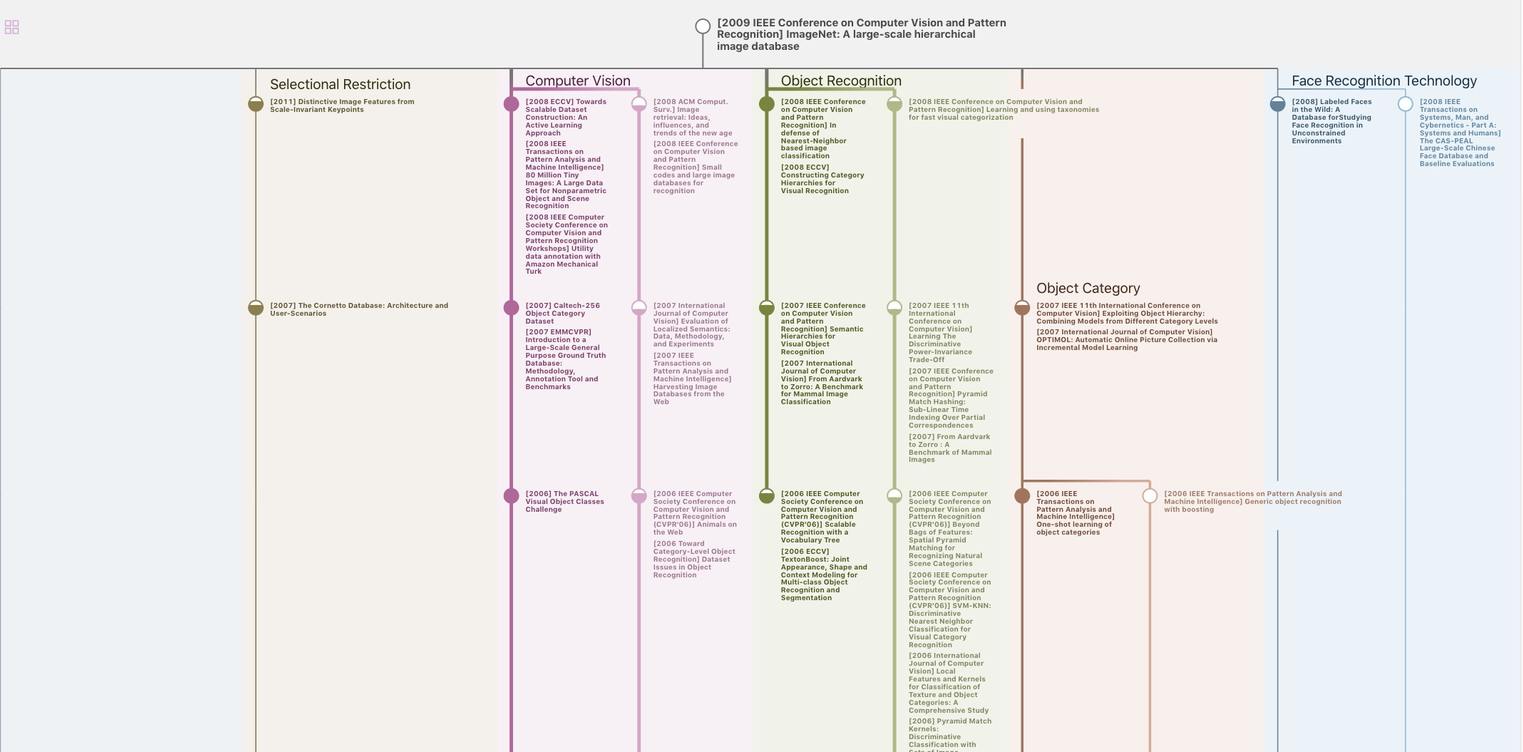
生成溯源树,研究论文发展脉络
Chat Paper
正在生成论文摘要