Encoding Seasonal Climate Predictions with Modular Neural Network
ICASSP 2024 - 2024 IEEE International Conference on Acoustics, Speech and Signal Processing (ICASSP)(2024)
摘要
We propose a novel modeling framework that efficiently encodes seasonal climate predictions to provide robust and reliable time-series forecasting for supply chain functions. The encoding framework enables effective learning of latent representations—be it uncertain seasonal climate prediction or other time-series data (e.g., buyer patterns)—via a modular neural network architecture. Our extensive experiments indicate that learning such representations to model seasonal climate forecasts results in an error reduction of approximately 13% to 17% across multiple real-world data sets compared to existing demand forecasting methods.
更多查看译文
关键词
Neural Network,Climate Prediction,Seasonal Climate,Network Modularity,Seasonal Prediction,Seasonal Climate Prediction,Modular Neural Network,Time Series,Supply Chain,Time Series Data,Latent Representation,Time Series Prediction,Demand Forecasting,Seasonal Forecasts,Uncertain Predictions,Root Mean Square Error,Climate Variability,Attention Mechanism,Climate Data,Spatial Domain,Mean Absolute Percentage Error,Temporal Coding,Error Metrics,Demand Prediction,Historical Observations,Forecasting Model,Historical Time Series,Temporal Attention
AI 理解论文
溯源树
样例
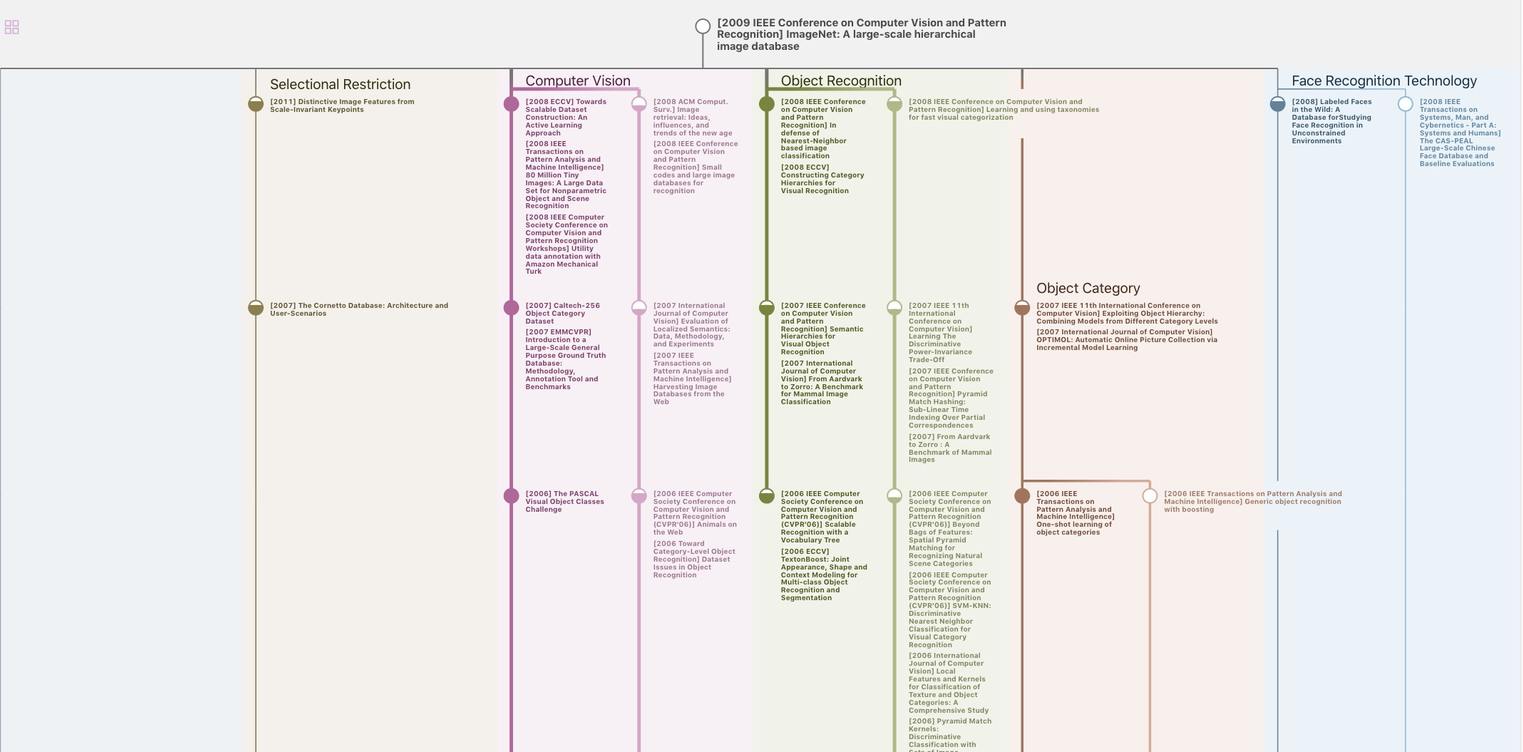
生成溯源树,研究论文发展脉络
Chat Paper
正在生成论文摘要