Gradient-Based Dimensionality Reduction for Speech Emotion Recognition Using Deep Networks
ICASSP 2024 - 2024 IEEE International Conference on Acoustics, Speech and Signal Processing (ICASSP)(2024)
摘要
This paper introduces a gradient-based approach for reducing the dimensionality of acoustic features, tailored for supervised deep learning models used in speech emotion recognition (SER). This method allows us to pinpoint the crucial acoustic features that the network heavily relies on, enabling us to simplify and retrain the network accordingly. It significantly boosts testing speed, making real-time SER systems suitable for embedded systems with resource constraints in speech processing units. The proposed method is evaluated on four convolutional neural network (CNN)-based deep learning models, and one of the best results demonstrates a 56.96% reduction in test time, albeit with a minor 3.81% drop in test accuracy. The method is compared with three mainstream dimensionality reduction techniques across various dimensions, consistently outperforming them in most scenarios. A Python implementation of the method is available at https://github.com/hxwangnus/Grad-based-Dim-Red-for-SER.git.
更多查看译文
关键词
speech emotion recognition,dimensionality reduction,deep neural networks
AI 理解论文
溯源树
样例
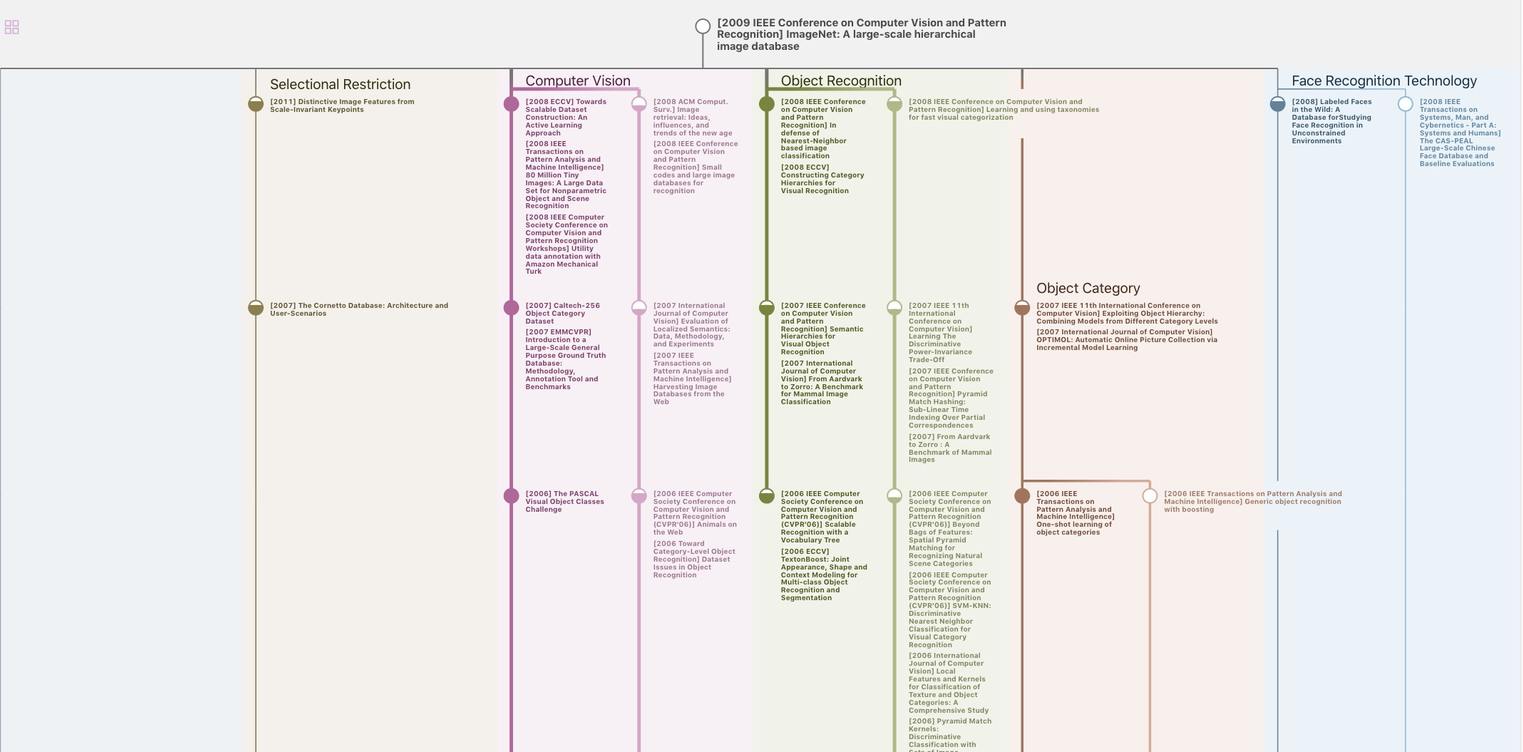
生成溯源树,研究论文发展脉络
Chat Paper
正在生成论文摘要