Multi-Linear Kernel Regression and Imputation VIA Manifold Learning: the Dynamic MRI Case
ICASSP 2024 - 2024 IEEE International Conference on Acoustics, Speech and Signal Processing (ICASSP)(2024)
摘要
This paper introduces an efficient multi-linear nonparametric (kernel-based) approximation framework for data regression and imputation. Data features are assumed to reside in or close to a smooth and userunknown manifold embedded in a reproducing kernel Hilbert space. Landmark points are identified to describe concisely the point cloud of features by linear approximating patches which mimic the concept of tangent spaces to smooth manifolds. The multi-linear model effects dimensionality reduction, enables efficient computations, and extracts data patterns and their geometry without any training data or additional information. Numerical tests on highly accelerated dynamic magnetic-resonance imaging (dMRI) data demonstrate remarkable improvements in efficiency and accuracy of the proposed approach over its predecessors and popular "shallow" data modeling methods, while offering substantial computational savings with regards to a deep-image-prior scheme.
更多查看译文
关键词
Regression,imputation,kernel,manifold,MRI
AI 理解论文
溯源树
样例
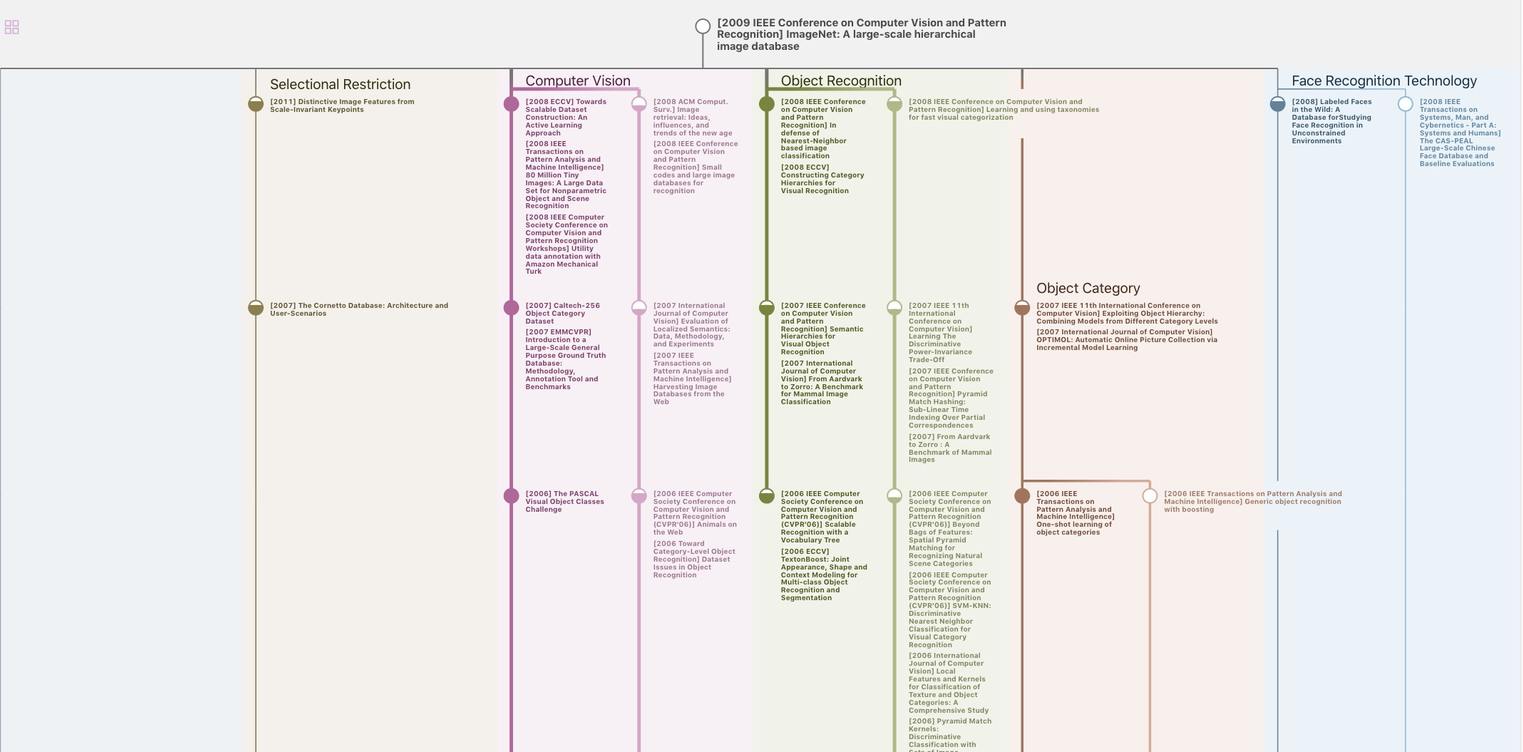
生成溯源树,研究论文发展脉络
Chat Paper
正在生成论文摘要