Improving Learned Video Compression by Exploring Spatial Redundancy
ICASSP 2024 - 2024 IEEE International Conference on Acoustics, Speech and Signal Processing (ICASSP)(2024)
摘要
Learned video compression has developed rapidly and shown promising rate-distortion performance recently. Existing works have made great progress on removing temporal redundancy between inter-frames, while neglecting spatial redundancy within a frame. In this paper, we propose to explore spatial redundancy to further improve compression efficiency and reduce computation complexity by extending inter-frame prediction from temporal prediction to spatial-temporal prediction. Specifically, the current frame is first down-sampled to reduce spatial redundancy, which is then temporally predicted to compensate motions and spatially predicted to restore resolutions. The spatial prediction module is designed in a reference-based manner, which improves prediction performance without additional bit rate cost by mining high-frequency details from a neighboring high-resolution decoded frame. Experimental results demonstrate that our proposed module can improve compression efficiency and reduce encoding/decoding time. Our framework achieves competitive compression efficiency compared with recent learned methods and traditional codecs.
更多查看译文
关键词
Learned video compression,spatial prediction
AI 理解论文
溯源树
样例
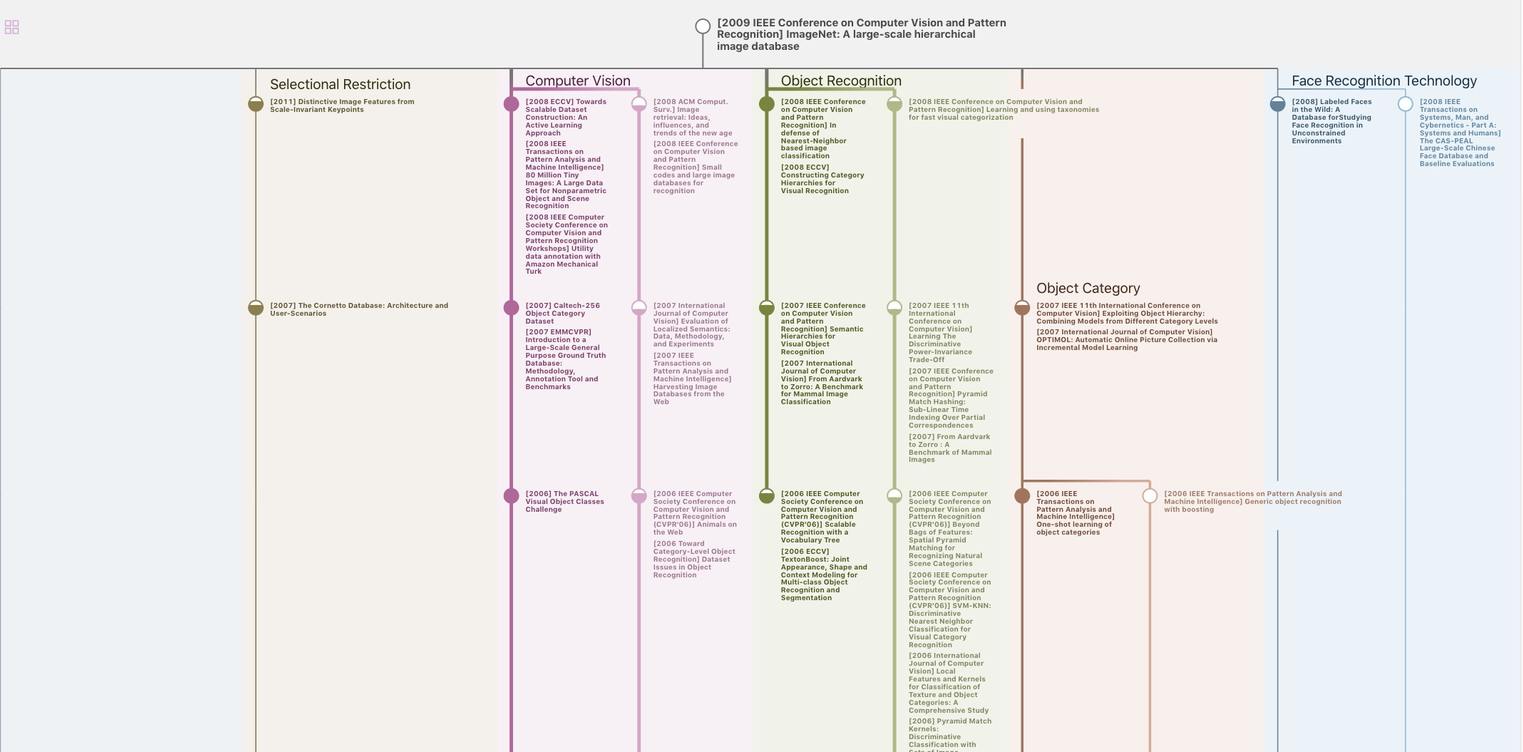
生成溯源树,研究论文发展脉络
Chat Paper
正在生成论文摘要