Phase Continuity-Aware Self-Attentive Recurrent Network with Adaptive Feature Selection for Robust VAD
ICASSP 2024 - 2024 IEEE International Conference on Acoustics, Speech and Signal Processing (ICASSP)(2024)
摘要
Deep neural network (DNN) applications have significantly progressed in voice activity detection (VAD). Most current DNN-based VAD methods ignore the rich audio information in the phase domain. Therefore, applying this auxiliary information rationally and coping with low signal-to-noise ratio (SNR) background noise environments remains one of the challenges for VAD. To address this problem, we propose a VAD model robust to noise called phase continuity-aware self-attentive recurrent network (PC-ARN). For the input of PC-ARN, we draw inspiration from recent speech enhancement research by introducing phase-related features and further employing an adaptive feature selection module (AFSM) to combine magnitude features with it efficiently. The backbone network is an ARN module combining the attention mechanism and recurrent neural network (RNN), which can consider the relationship between local and global information to improve VAD performance competently. Experimental results show that our method has remarkable generalization ability and robustness compared to the traditional VAD techniques.
更多查看译文
关键词
Voice activity detection,phase continuity,self-attention,recurrent neural network,adaptive feature selection module
AI 理解论文
溯源树
样例
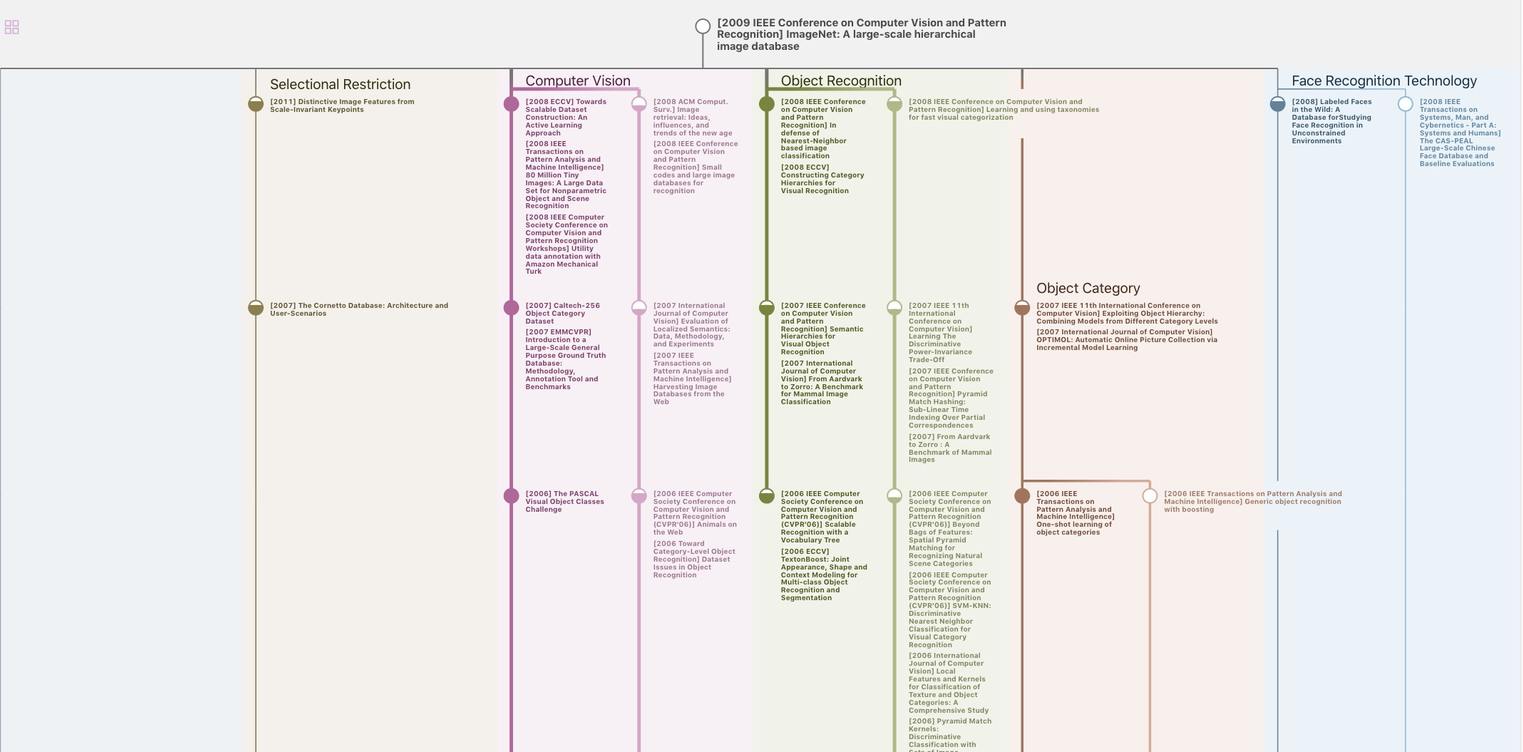
生成溯源树,研究论文发展脉络
Chat Paper
正在生成论文摘要