UCF-PKS: Unforeseen Consumer Fraud Detection With Prior Knowledge and Semantic Features
IEEE TRANSACTIONS ON COMPUTATIONAL SOCIAL SYSTEMS(2024)
摘要
The utilization of text classification techniques has demonstrated great promise in the field of detecting consumer fraud based on consumer reviews. However, persistent challenges remain in handling large samples at the borders and identifying unforeseen fraud behaviors. To address these challenges, we propose a novel approach that combines a channel biattention convolutional neural network (CNN) with a pretrained language model. Specifically, we propose a similarity computation module for implicitly learning a metric matrix to characterize the similarity between prior knowledge and consumer reviews in vector space. Through this process, the model is able to learn and understand the relationship between prior knowledge and corresponding samples during training, thereby improving its ability to identify unforeseen fraudulent behaviors. Additionally, we propose a channel biattention CNN module to adaptively emphasize the importance of relevant prior knowledge to enhance the model's ability to accurately classify boundary samples. To ensure effective model training, we expand and organize a real-world dataset, reducing noise and increasing the number of fraud samples available for analysis. Experimental results demonstrate that our approach achieves state-of-the-art performance in fraud detection. Notably, our model is capable of detecting unforeseen fraud cases without the need for retraining or fine-tuning, making it highly adaptable and efficient in practical applications.
更多查看译文
关键词
Consumer fraud,fraud detection,priori knowledge,semantic features,unforeseen fraud behaviour
AI 理解论文
溯源树
样例
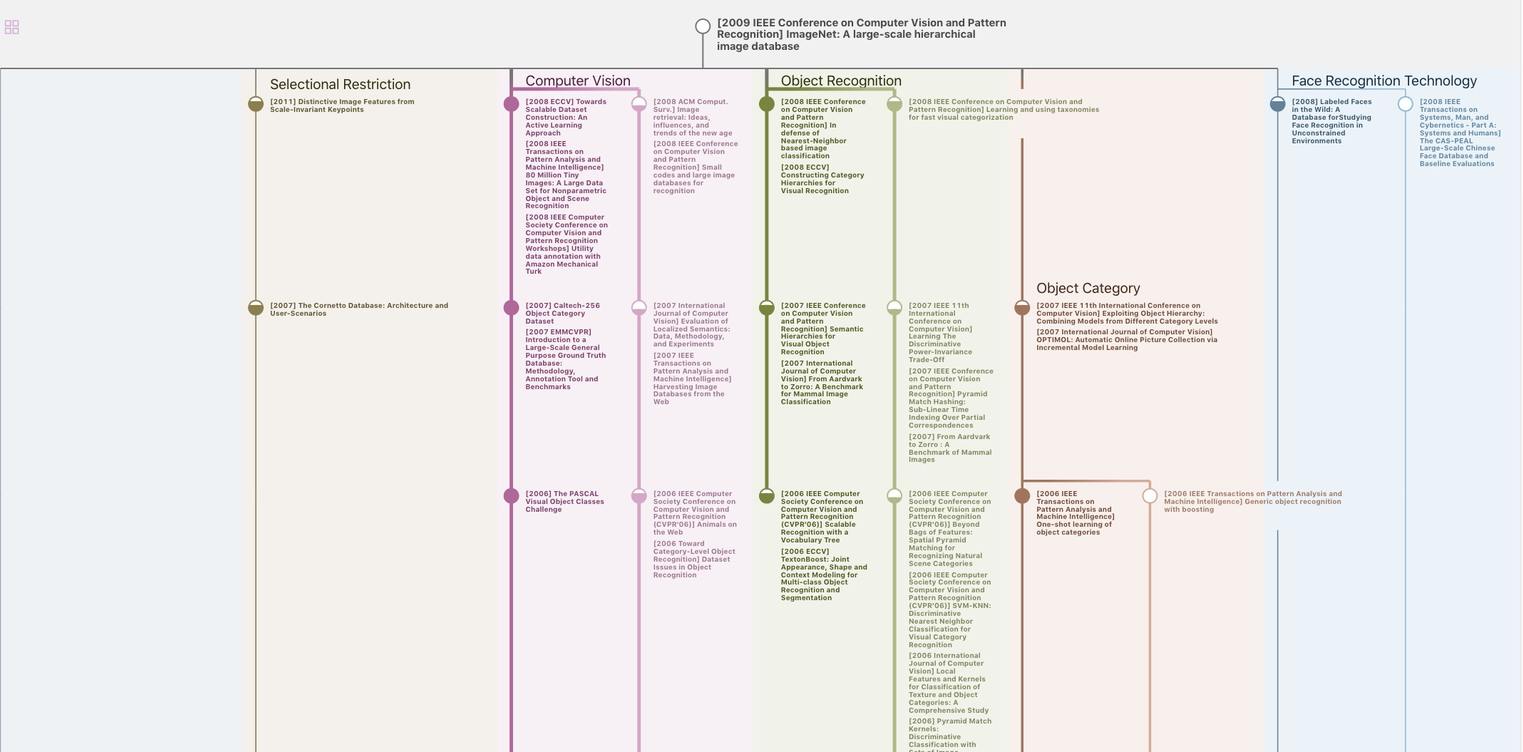
生成溯源树,研究论文发展脉络
Chat Paper
正在生成论文摘要