Generative Extension Positive Pairs and Improving Sample Selection Based on Contrastive Learning for Unsupervised Person Re-Identification
ICASSP 2024 - 2024 IEEE International Conference on Acoustics, Speech and Signal Processing (ICASSP)(2024)
摘要
In this paper, we present Generative Extension Positive Pairs (GEPP), a novel approach to enhance unsupervised person re-identification (re-id) through contrastive learning. Data generation and pair selection methods significantly impact model performance in contrastive learning. To improve positive pair generation, we incorporate a Generative Adversarial Network (GAN) to create novel views as augmentation samples. We also introduce a sample selection scheme in the contrastive learning process to effectively choose GAN-augmented positive samples. Leveraging our sample selection results, we construct the GEPP framework and propose a unique loss function for contrastive learning. Experimental results showcase that our generative extension of positive pairs and sample selection method offer a versatile, automated, and diverse approach, achieving higher mean average precision (mAP) in re-id tasks than conventional data augmentation techniques. Additionally, our framework outperforms existing state-of-the-art methods on the Market-1501 and MSMT17 datasets. The source code is available at https://github.com/andy412510/Contrastive-sample.
更多查看译文
关键词
Generative adversarial network,contrastive learning,person re-identification
AI 理解论文
溯源树
样例
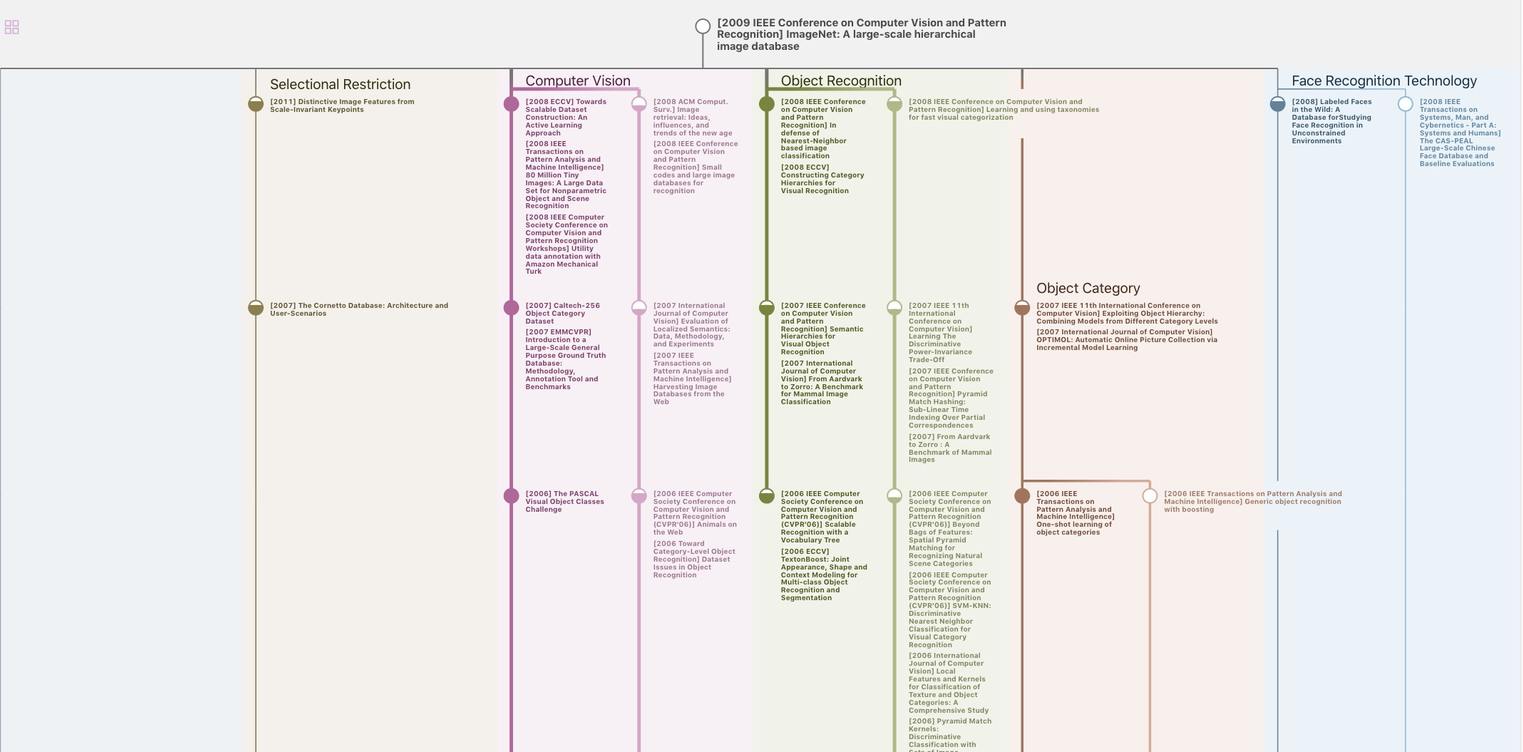
生成溯源树,研究论文发展脉络
Chat Paper
正在生成论文摘要