Improving Motion Deblur By Multi-Output Learning
ICASSP 2024 - 2024 IEEE International Conference on Acoustics, Speech and Signal Processing (ICASSP)(2024)
摘要
Image deblurring is an ill-posed task, where exists infinite feasible solutions for blurry images. Modem deep learning approaches usually discard the learning of blur kernels and directly employ end-to-end supervised learning. However, supervised learning can’t handle ill-posed tasks appropriately. It regresses the average thus losing sharp details. Therefore, we propose an extension to the network to learn from stochastic supervisions, where a novel multi-output architecture and Min-Out loss function are designed. Our approach enables the model to output multiple feasible solutions to fit various non-uniform motions. We then propose a novel parameter multiplexing method that reduces computations while improving performance with fewer parameters. After training, the best-performed head is fine-tuned to be used for inference where the sharp label is absent. The proposed approach is evaluated with multiple image deblur models on the GoPro motion deblur dataset. On average, the multi-output extension improves the PSNR by 0.08 dB. When applied to the popular attention-based model Restormer, the multi-output helps it achieve 33.05 dB (+0.13 dB) PSNR without modification on network architecture.
更多查看译文
关键词
low-level vision,image deblur,motion deblur
AI 理解论文
溯源树
样例
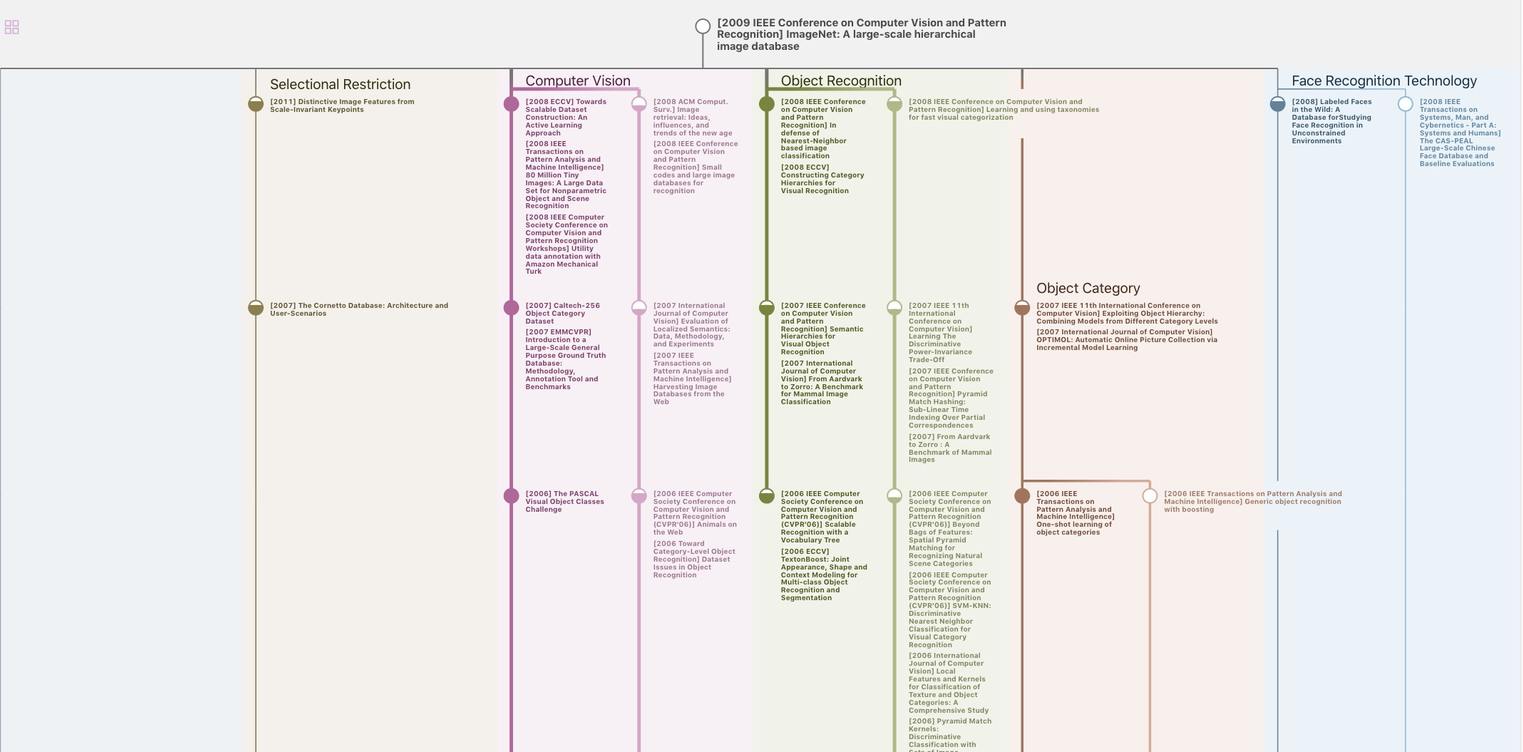
生成溯源树,研究论文发展脉络
Chat Paper
正在生成论文摘要