Trades++: Enhancing Multi-Object Tracking of Real Low Confidence Targets Using a Pyramid-Like Self-Attention Model
ICASSP 2024 - 2024 IEEE International Conference on Acoustics, Speech and Signal Processing (ICASSP)(2024)
摘要
In reality, multi-object tracking (MOT) is used in a wide range of scenarios. Maintaining the motion trajectory of the target, especially in high-density pedestrian scenarios, is often difficult. The tracking quality of most multi-object trackers correlates strongly with the detector quality and they often ignore the low-scoring detection boxes obtained by the detector. In this paper, we propose a TraDeS-based method called TraDeS++ that enhances the detection features using a pyramid-like self-attention model, significantly reducing the model training time and achieving a reduction of half the training epochs. The second motivation is to focus on the association method. We use a two-stage matching strategy with GIoU constraints, effectively improving HOTA. Experimental results show that our component effectively improves the metrics of MOT, especially MOTA, HOTA, and IDF1. Competitive results are achieved on the popular MOT16 and MOT17 datasets.
更多查看译文
关键词
Multi-object tracking,TraDeS,pyramid-like self-attention model,GIoU constraints
AI 理解论文
溯源树
样例
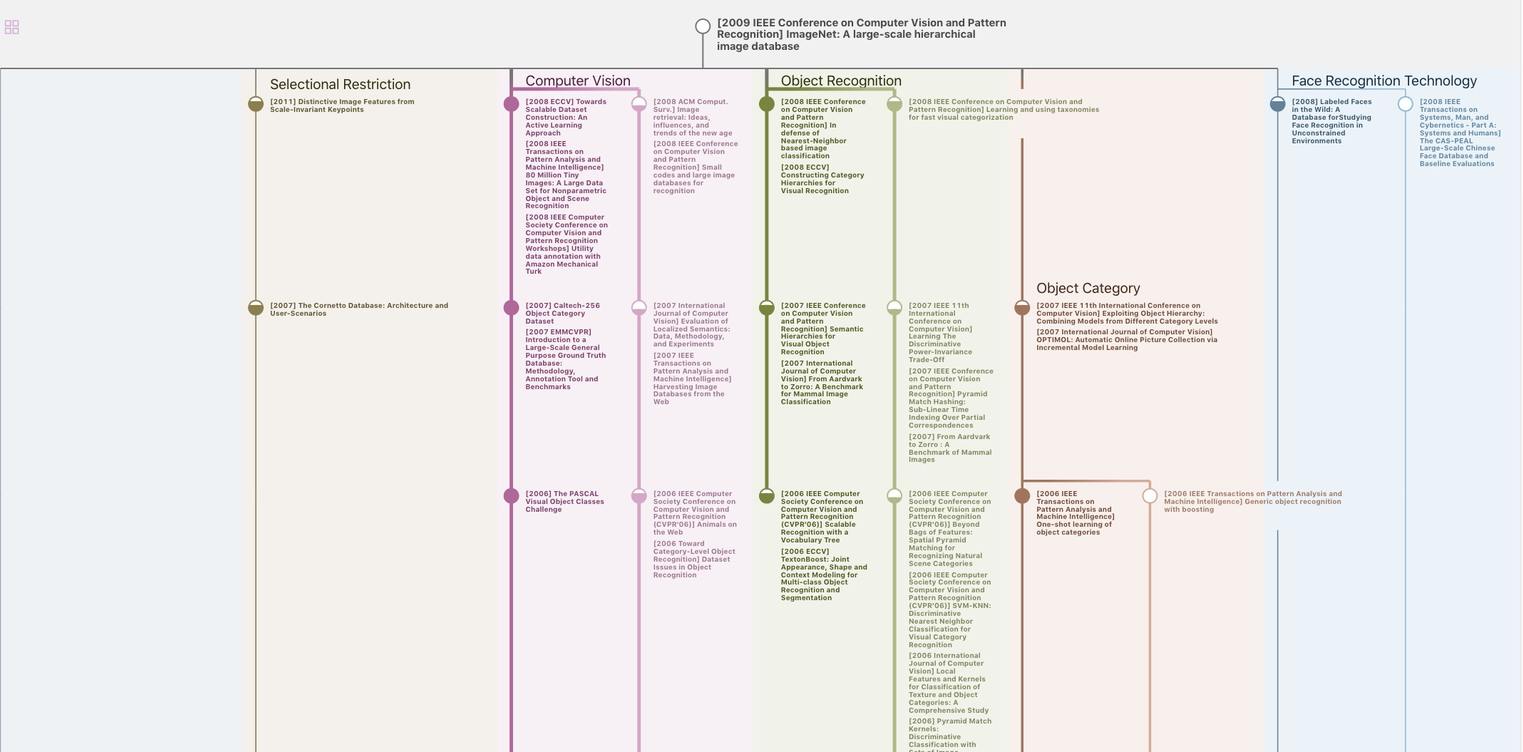
生成溯源树,研究论文发展脉络
Chat Paper
正在生成论文摘要