Hidden in Time, Revealed in Frequency: Spectral Features and Multiresolution Analysis for Encrypted Internet Traffic Classification.
Consumer Communications and Networking Conference(2024)
摘要
In recent years, privacy and security concerns have led to the wide adoption of encrypted protocols, making encrypted traffic a major portion of overall communications online. The transition into more secure protocols poses significant challenges for internet service providers to utilize traditional traffic classification techniques in order to guarantee the Quality of Service (QoS), Quality of Experience (QoE), and cyber-security of their customers. In this work, we introduce two methods, namely STFT-TC and DWT-TC, leveraging compact time-series representation coupled with well-known techniques from the field of Digital Signal Processing (DSP): the short-time Fourier transform (STFT) and the discrete wavelet transform (DWT). The STFT-TC method extracts a suite of statistical and spectral features from the magnitude spectrogram, offering a fresh perspective on interpreting and classifying encrypted traffic. The DWT-TC method extracts statistical features from the wavelet coefficients and incorporates unique characteristics that describe the signal's shape and energy distribution. Evaluating our methods on two public QUIC datasets demonstrated improvements in accuracy of up to 5.7%. Similarly, the F1-scores also showed enhancements, with increments of up to 5.9% for the same datasets.
更多查看译文
关键词
Spectral Features,Internet Traffic,Traffic Classification,Fourier Transform,Service Quality,Wavelet Transform,Statistical Features,Quality Of Experience,Signal Energy,Digital Signal Processing,Compact Representation,Short-time Fourier Transform,Internet Service Providers,Representative Time Series,Deep Neural Network,Low-pass,Time Series Data,Window Size,High-pass Filter,Random Forest Model,Random Forest Classifier,Approximation Coefficients,Time-frequency Analysis,Spectral Centroid,Mel-frequency Cepstral Coefficients,Benchmark Model,Conditions Hold,Key Metrics,Signal Waveform,Decomposition Level
AI 理解论文
溯源树
样例
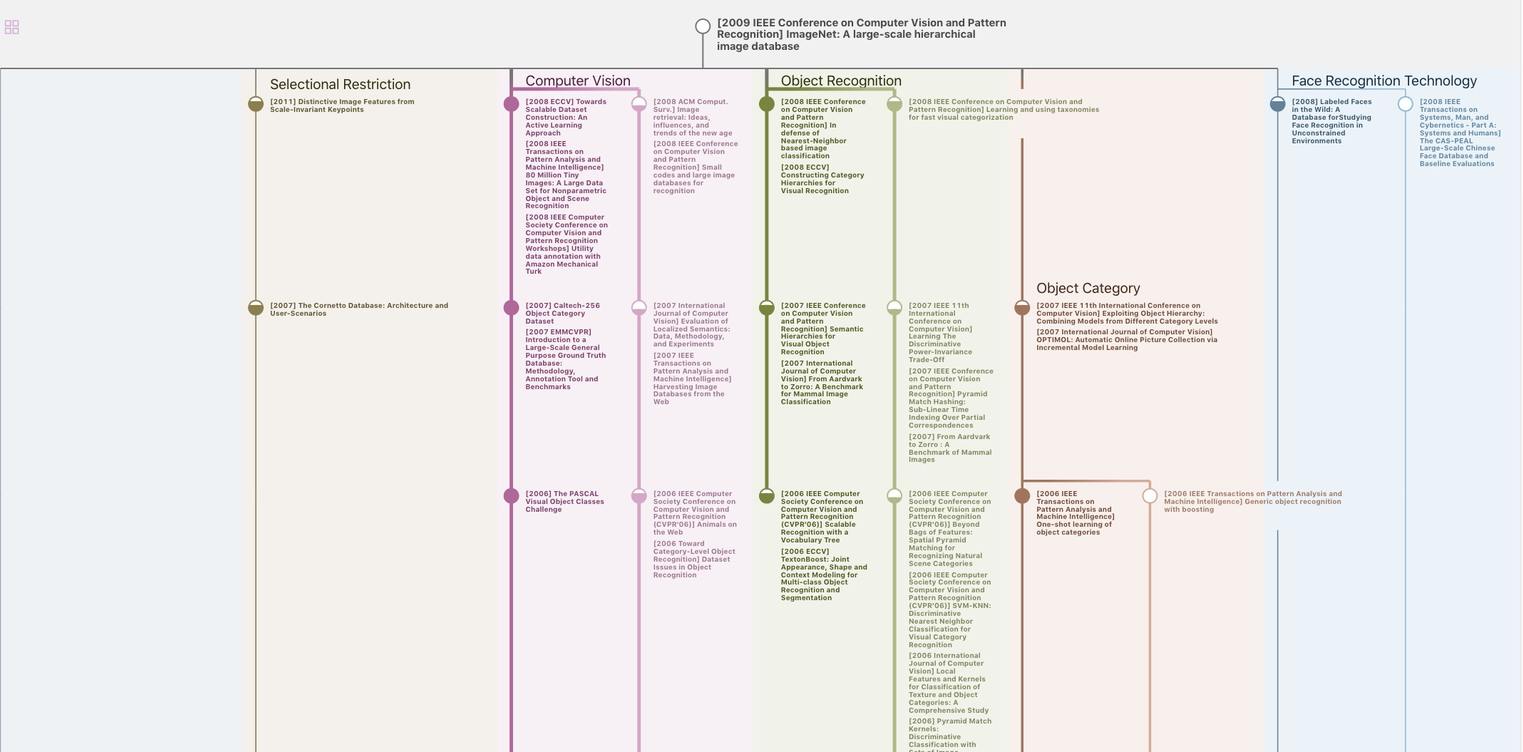
生成溯源树,研究论文发展脉络
Chat Paper
正在生成论文摘要