Domain-Slot Aware Contrastive Learning for Improved Dialogue State Tracking
ICASSP 2024 - 2024 IEEE International Conference on Acoustics, Speech and Signal Processing (ICASSP)(2024)
摘要
Large-scale pre-trained neural language model has facilitated to achieve the state-of-the-art performance on Dialogue State Tracking (DST) tasks. One of the existing works models the semantic correlation between the dialogue context and (domain, slot) pair encoded by BERT and make the prediction. Despite the effectiveness, they ignore the fact that there is no perfect semantic correspondence between (domain, slot) pair and the dialogue context. In this paper, we propose a domain-slot aware contrastive learning framework to solve this problem, which proposes three methods to bridge the semantic gap between the dialogue context and the (domain, slot) by constructing training sample pairs to fine-tune the BERT model and use it for base DST model. The experiments demonstrate that our proposed method has improved the performance of the baseline model on the MultiWOZ2.1 and MultiWOZ2.4 datasets, yielding competitive results.
更多查看译文
关键词
Contrastive learning,Task-oriented dialogue system,Dialogue state tracking
AI 理解论文
溯源树
样例
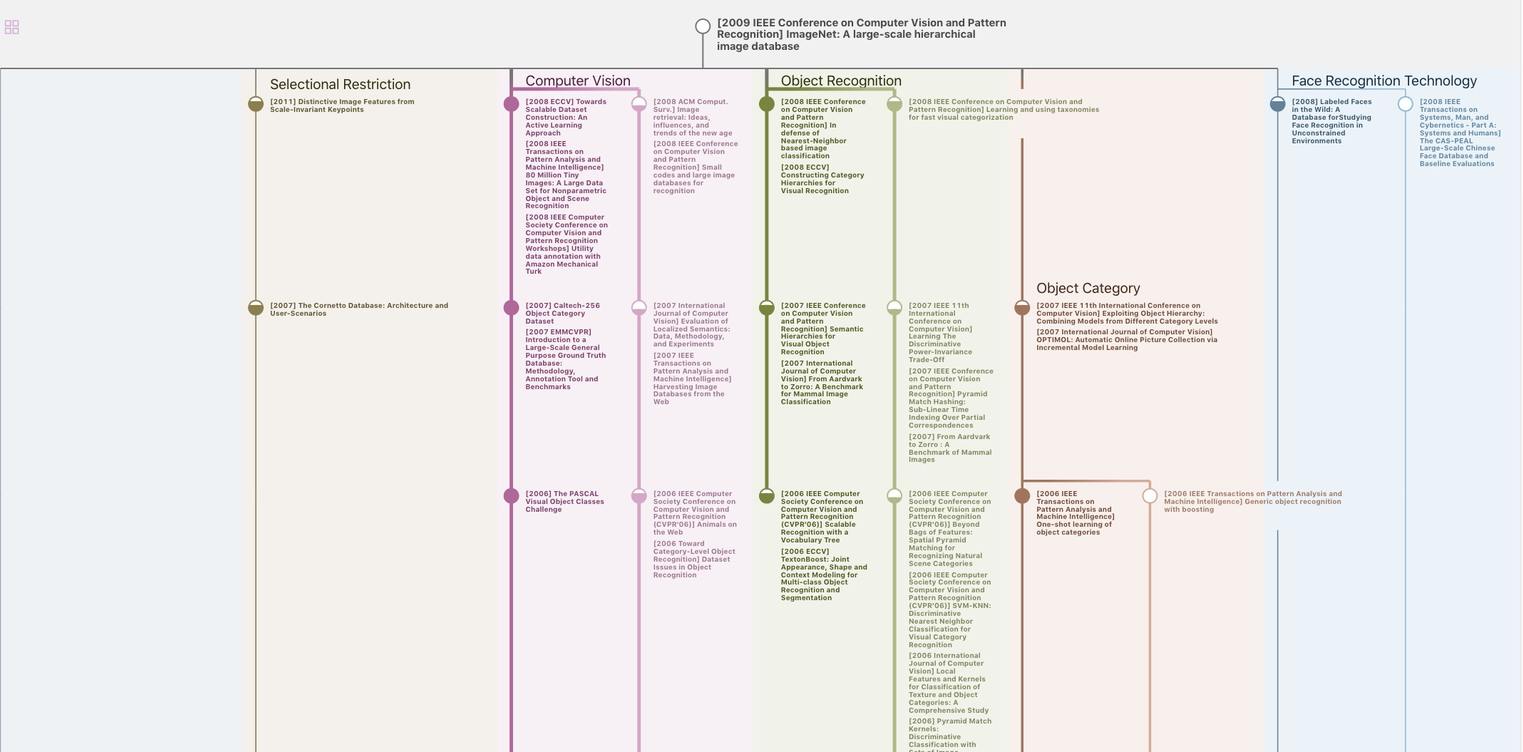
生成溯源树,研究论文发展脉络
Chat Paper
正在生成论文摘要