Adaptive Multiview Community-Preserved Graph Convolutional Network for Multiatlas-Based Functional Connectivity Analysis
ICASSP 2024 - 2024 IEEE International Conference on Acoustics, Speech and Signal Processing (ICASSP)(2024)
摘要
Recently, functional connectivity network (FCN) analysis via graph convolutional networks (GCNs) has greatly boosted diagnostic performance of brain diseases on a population graph for subject classification. However, most existing methods only focus on FCNs based on a single brain atlas (ignoring complementary information among multiatlas-based FCNs), and the population graph structure is preconstructed and fixed during the GCN training (not truly reflecting the relation between subjects). In this paper, we propose an adaptive multiview community-preserved graph convolutional network (CP-GCN) method to accommodate multiatlas-based FCNs. Specifically, we first introduce a multiview FCN fusion module to obtain multiatlas FCN embeddings via concatenating both intra- and inter-atlas embeddings that are extracted separately from fully connected layers. We then develop a multihead similarity learning module to adaptively learn the population graph structure, best serving GCN for node classification. Finally, under the learned graph structure, a CP-GCN based node classification module is applied for subject classification through designing a community-preserved constraint on the GCN. Experimental results on the ABIDE validate the effectiveness of our method for autism identification, and our findings related to autism can be easily traced back with biological interpretability.
更多查看译文
关键词
Functional connectivity,graph convolutional network,subject classification
AI 理解论文
溯源树
样例
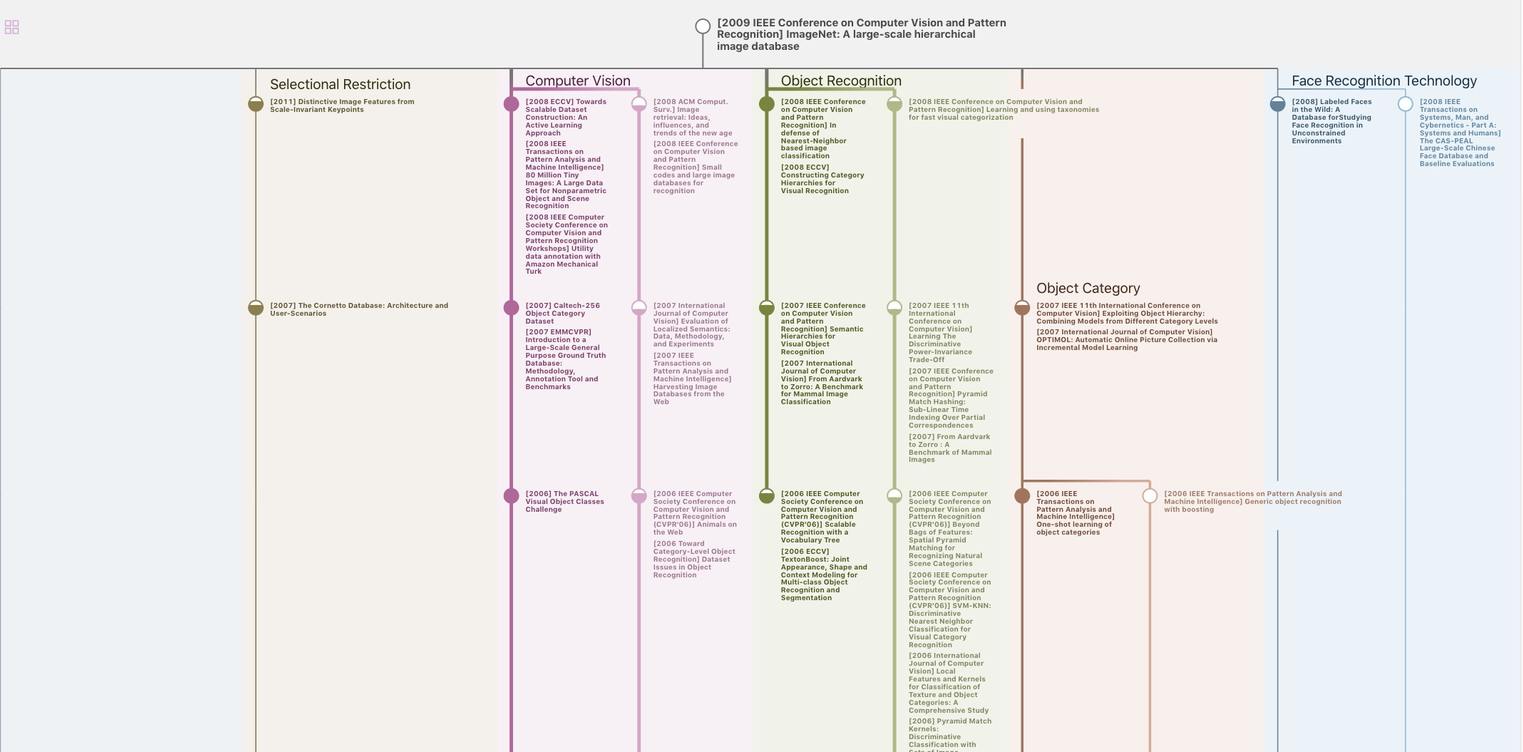
生成溯源树,研究论文发展脉络
Chat Paper
正在生成论文摘要