Gradient Inversion Attacks on Acoustic Signals: Revealing Security Risks in Audio Recognition Systems
ICASSP 2024 - 2024 IEEE International Conference on Acoustics, Speech and Signal Processing (ICASSP)(2024)
摘要
With a greater emphasis on data confidentiality and legislation, distributed training and collaborative machine learning algorithms are being developed to protect sensitive private data. Gradient exchange has become a widely used practice in those multi-node machine learning systems. But with the advent of gradient inversion attacks, it is already established that private training data can be revealed from the gradients. Gradient inversion attacks covertly spy on gradient updates and backtrack from the gradients to obtain information about the raw data. Although this attack has been widely studied in computer vision and natural language processing tasks, understanding the impact of this attack on acoustic signals still requires a comprehensive investigation. To the best of our knowledge, we are the first to explore gradient inversion attacks on acoustic signals by extracting the speakers’ voices from an audio recognition system. Here, we design a new application of gradient inversion attack to retrieve the audio signal used for training the deep learning model, irrespective of whether the audio has undergone conversion into mel-spectrogram or MFCC representations prior to feed to neural network. Experimental results demonstrate the capability of our attack method to extract the input vectors of the audio data from the gradients, which highlight the security risks in revealing the sensitive audio data from highly secured systems. We also discuss several possible strategies as countermeasures and their effectiveness to prevent the attack.
更多查看译文
关键词
Data privacy,Adversarial attacks,Audio
AI 理解论文
溯源树
样例
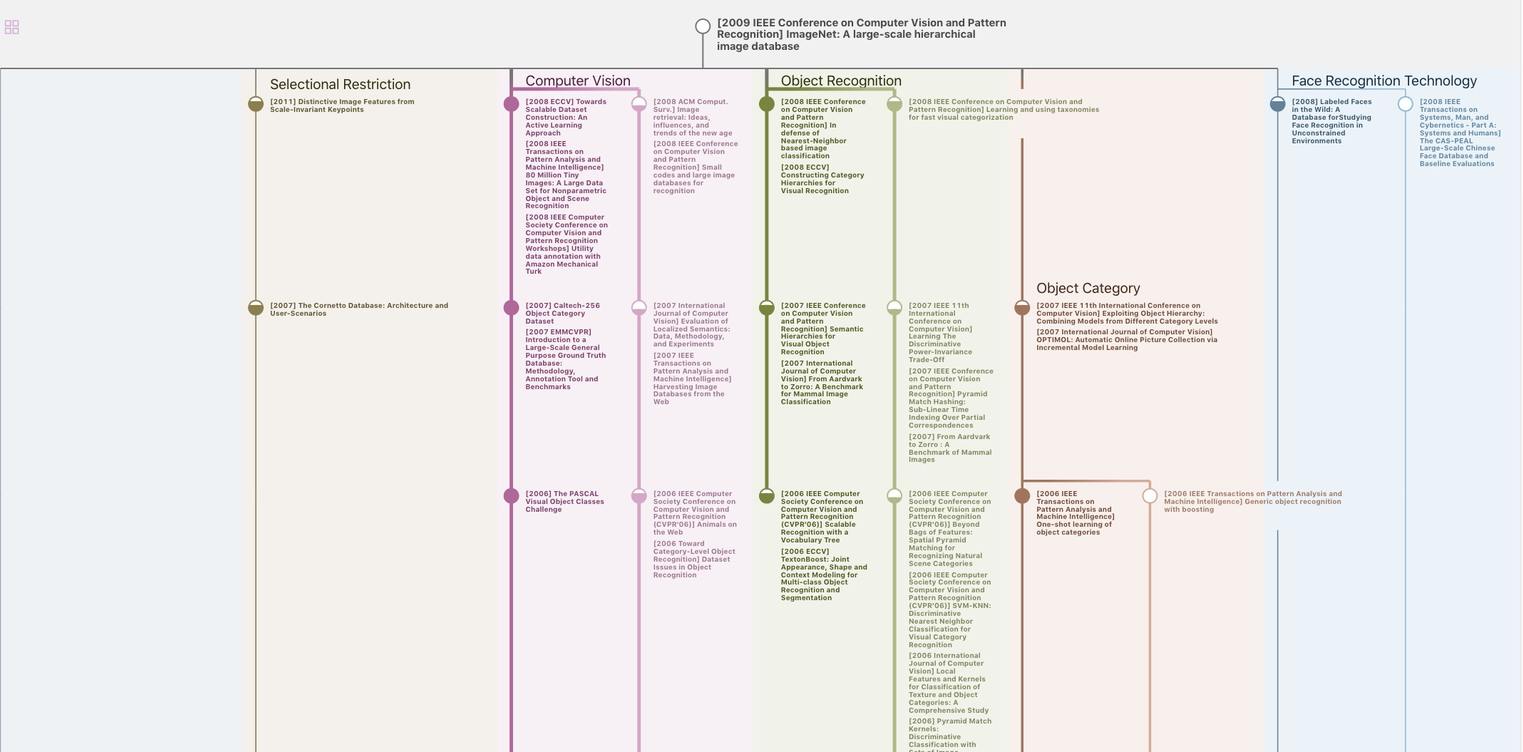
生成溯源树,研究论文发展脉络
Chat Paper
正在生成论文摘要