SPDG-Net: Semantics Preserving Domain Augmentation through Style Interpolation for Multi-Source Domain Generalization
ICASSP 2024 - 2024 IEEE International Conference on Acoustics, Speech and Signal Processing (ICASSP)(2024)
摘要
This paper focuses on domain generalization (DG), addressing the challenge of robust classifier learning from multiple source domains for generalizing to unseen ones. DG suffers from limited source domain diversity, which may hinder model generalization. Recent studies explore domain-augmentation strategies but struggle to maintain semantics while altering image styles and generate only a few pseudo domains. To tackle this, we introduce Semantics Preserving DG Network (SPDG-Net). SPDG-Net is a triplet-conditioned U-Net-based GAN that synthesizes various pseudo domains, preserving image semantics through a cycle-consistency constraint. Moreover, our style interpolation-based domain generation produces fine-grained synthetic domains, unlike existing models. We also propose recognizing image styles alongside object classes to reduce model bias. Our experiments across benchmark datasets consistently outperform recent literature in DG.
更多查看译文
关键词
Domain Generalization,Cross Domain Learning,Machine Learning,Deep Learning,Image Analysis
AI 理解论文
溯源树
样例
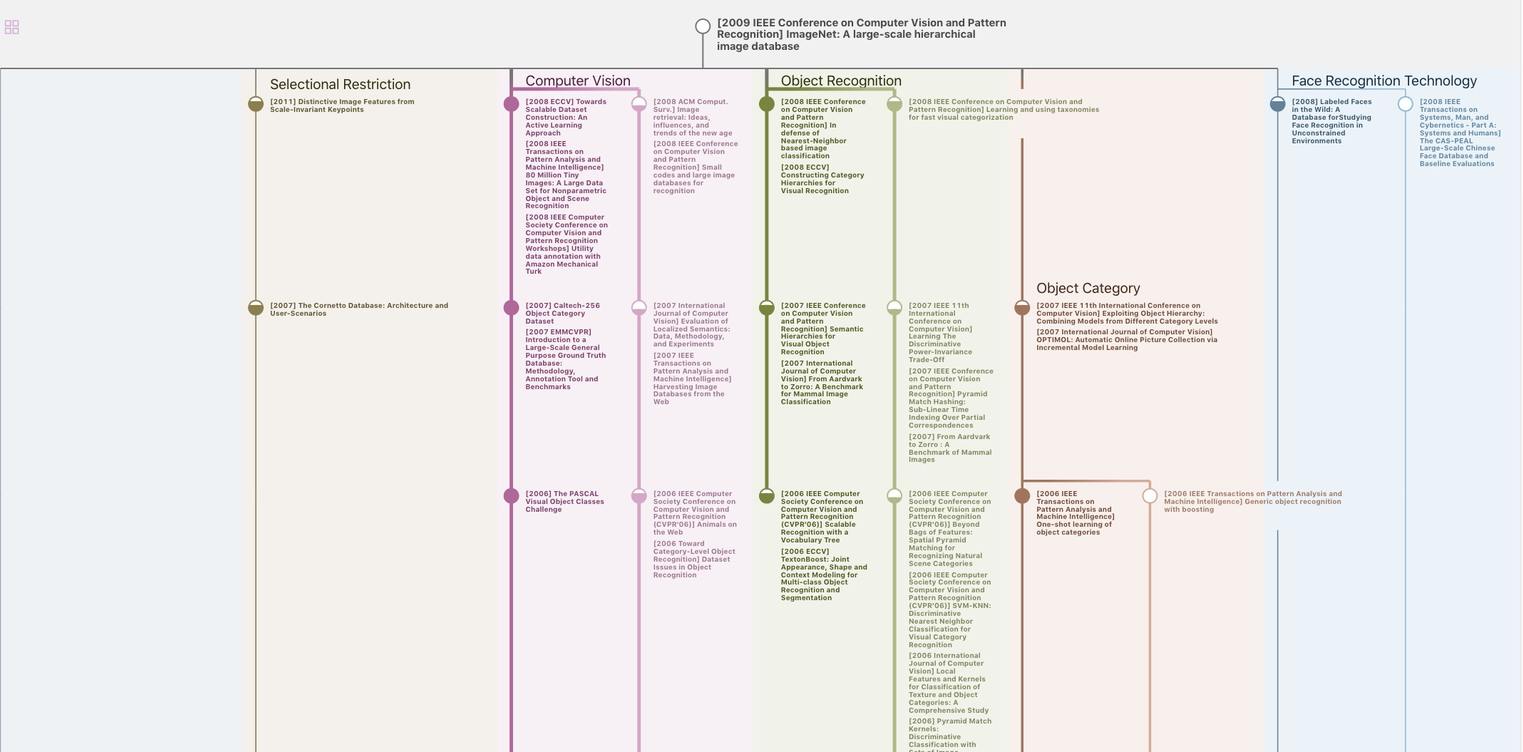
生成溯源树,研究论文发展脉络
Chat Paper
正在生成论文摘要