Adaptive Gaussian Regularization Constrained Sparse Subspace Clustering for Image Segmentation
ICASSP 2024 - 2024 IEEE International Conference on Acoustics, Speech and Signal Processing (ICASSP)(2024)
摘要
Sparse Subspace Clustering (SSC) is integral to image processing, drawing from spectral clustering foundations. However, prevalent methods, relying on an l
1
-norm constraint, fail to capture nuanced inter-region correlations, affecting segmentation efficacy. To remedy this, we introduce an Adaptive Gaussian Regularization Constrained SSC for enhanced image segmentation. This method begins with superpixel preprocessing to enrich local information. Given the Gaussian nature of the SSC’s sparse coefficient matrix, a Gaussian probability density function is infused as a regularization term, reinforcing regional image ties and facilitating similarity matrix creation. Using spectral clustering, we then define superpixel clusters leading to the final segmentation. When tested against the BSDS500 and SBD datasets and other leading algorithms, our model showcases marked improvements in natural image segmentation.
更多查看译文
关键词
SSC,Adaptive,l1-norm,Gaussian Regularization,Image Segmentation
AI 理解论文
溯源树
样例
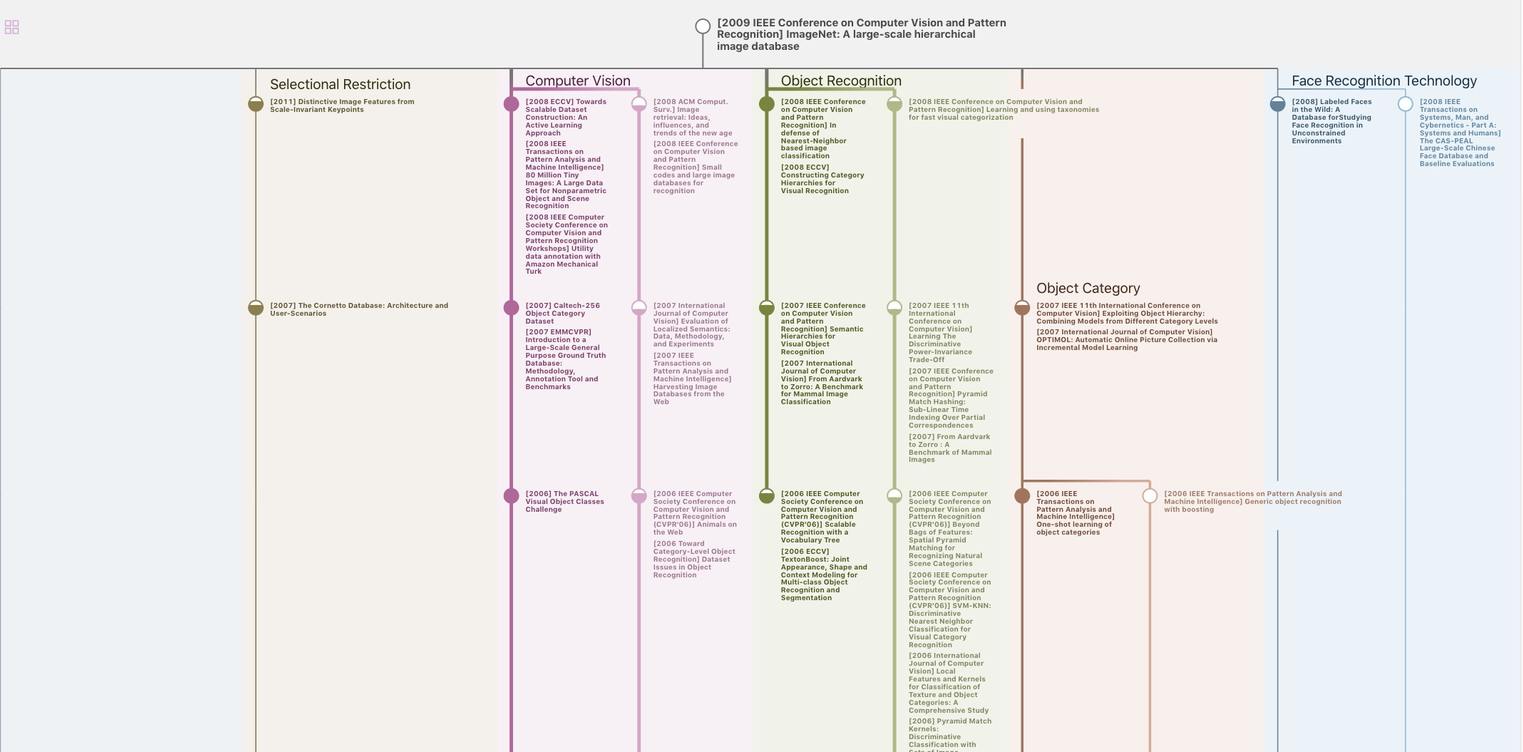
生成溯源树,研究论文发展脉络
Chat Paper
正在生成论文摘要