Retaining Informative Latent Variables in Probabilistic Segmentation
ICASSP 2024 - 2024 IEEE International Conference on Acoustics, Speech and Signal Processing (ICASSP)(2024)
摘要
Conditional latent-variable models can successfully quantify annotation variability in segmentation. Training such models involves tuning the dimensionality of the latent space to optimally capture the inherent data ambiguity. Nevertheless, we discover after careful tuning, that the latent space does not always reflect this. In fact, some latent dimensions are completely neglected. For such segmentation models the latent dimensionality is often poorly motivated or based on computational constraints. In this paper, we offer an information-theoretic approach to optimally leverage all latent dimensions. We adapt and improve the Probabilistic U-Net to maximize the mutual information between the latent and output variables, leading to improved latent space properties and higher segmentation performance.
更多查看译文
关键词
segmentation,aleatoric uncertainty,generative modeling
AI 理解论文
溯源树
样例
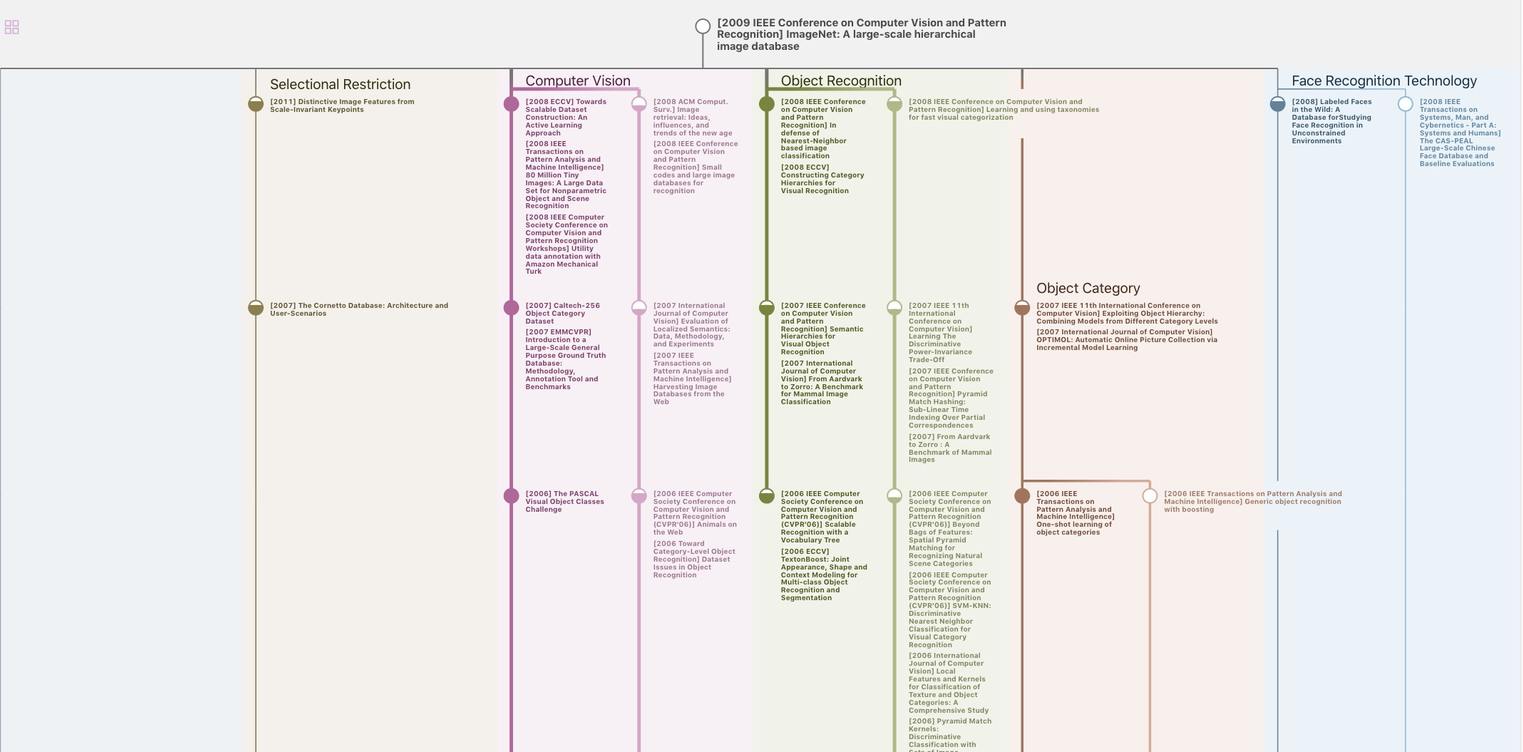
生成溯源树,研究论文发展脉络
Chat Paper
正在生成论文摘要