Machine learning modeling of fluorescence spectral data for prediction of trace organic contaminant removal during UV/H2O2 treatment of wastewater
Water Research(2024)
摘要
Dynamic feedback of the removal performance of trace organic contaminants (TrOCs) is essential towards economical advanced oxidation processes (AOPs), whereas the corresponding quick-response feedback methods have long been desired. Herein, machine learning (ML) multi-target regression random forest (MORF) models were developed based on the fluorescence spectra to predict the removal of TrOCs during UV/H2O2 treatment of municipal secondary effluent as a typical AOP. The predictive performance of the developed MORF model (R2 = 0.83-0.95) exhibited higher accuracy over the traditional linear regression models with R2 increased by ∼0.15. Furthermore, through feature importance analysis, the spectral regions of high importance were identified for different groups of TrOCs, thus enabling faster data acquisition due to remarkably reduced size of required fluorescence spectral scanning region. Specifically, the fluorescence regions Ex(235-275 nm)/Em(325-400 nm) and Ex(240-360 nm)/Em(325-450 nm) were found highly correlated with the removal of the TrOCs susceptible to both photodegradation and •OH degradation and those primarily subject to •OH degradation, respectively. In addition, the spectral regions of high importance were also individually identified for the investigated TrOCs during the AOP. Through providing an efficient ML-based feedback method to monitor TrOC removal during AOP, this study sheds light on the development of dynamic feedback-based strategies for precise and economical advanced treatment of wastewater.
更多查看译文
关键词
advanced oxidation,micropollutant removal,machine learning,multi-target regression random forest model,excitation-emission matrix
AI 理解论文
溯源树
样例
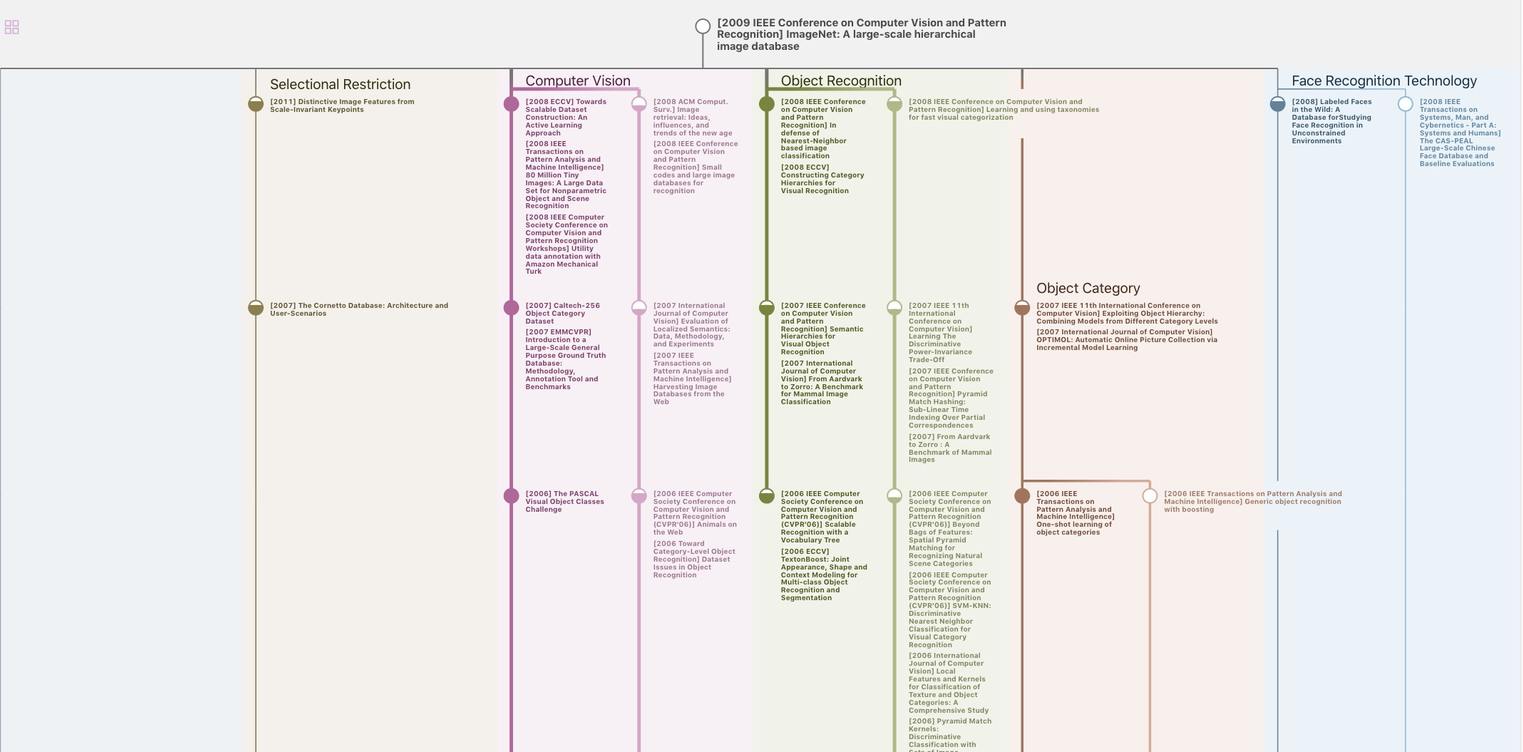
生成溯源树,研究论文发展脉络
Chat Paper
正在生成论文摘要