Large-scale plasma proteomics in the UK Biobank modestly improves prediction of major cardiovascular events in a population without previous cardiovascular disease.
European journal of preventive cardiology(2024)
摘要
AIMS:Improved identification of individuals at high risk of developing cardiovascular disease would enable targeted interventions and potentially lead to reductions in mortality and morbidity. Our aim was to determine whether use of large-scale proteomics improves prediction of cardiovascular events beyond traditional risk factors (TRFs).
METHODS:Using proximity extension assays, 2919 plasma proteins were measured in 38 380 participants of the UK Biobank. Both data- and literature-based feature selection and trained models using extreme gradient boosting machine learning were used to predict risk of major cardiovascular events (MACE: fatal and non-fatal myocardial infarction, stroke and coronary artery revascularisation) during a 10-year follow-up. Area under the curve (AUC) and net reclassification index (NRI) were used to evaluate the additive value of selected protein panels to MACE prediction by Systematic COronary Risk Evaluation 2 (SCORE2) or the 10 TRFs used in SCORE2.
RESULTS:SCORE2 and SCORE2 refitted to UK Biobank data predicted MACE with AUCs of 0.740 and 0.749, respectively. data-driven selection identified 114 proteins of greatest relevance for prediction. Prediction of MACE was not improved by using these proteins alone (AUC of 0.758) but was significantly improved by combining these proteins with SCORE2 or the 10 TRFs (AUC=0.771, p<001, NRI=0.140, and AUC=0.767, p=0.03, NRI 0.053, respectively). Literature-based protein selection (113 proteins from five previous studies) also improved risk prediction beyond TRFs while a random selection of 114 proteins did not.
CONCLUSIONS:Large-scale plasma proteomics with data-driven and literature-based protein selection modestly improves prediction of future MACE beyond TRFs.
更多查看译文
AI 理解论文
溯源树
样例
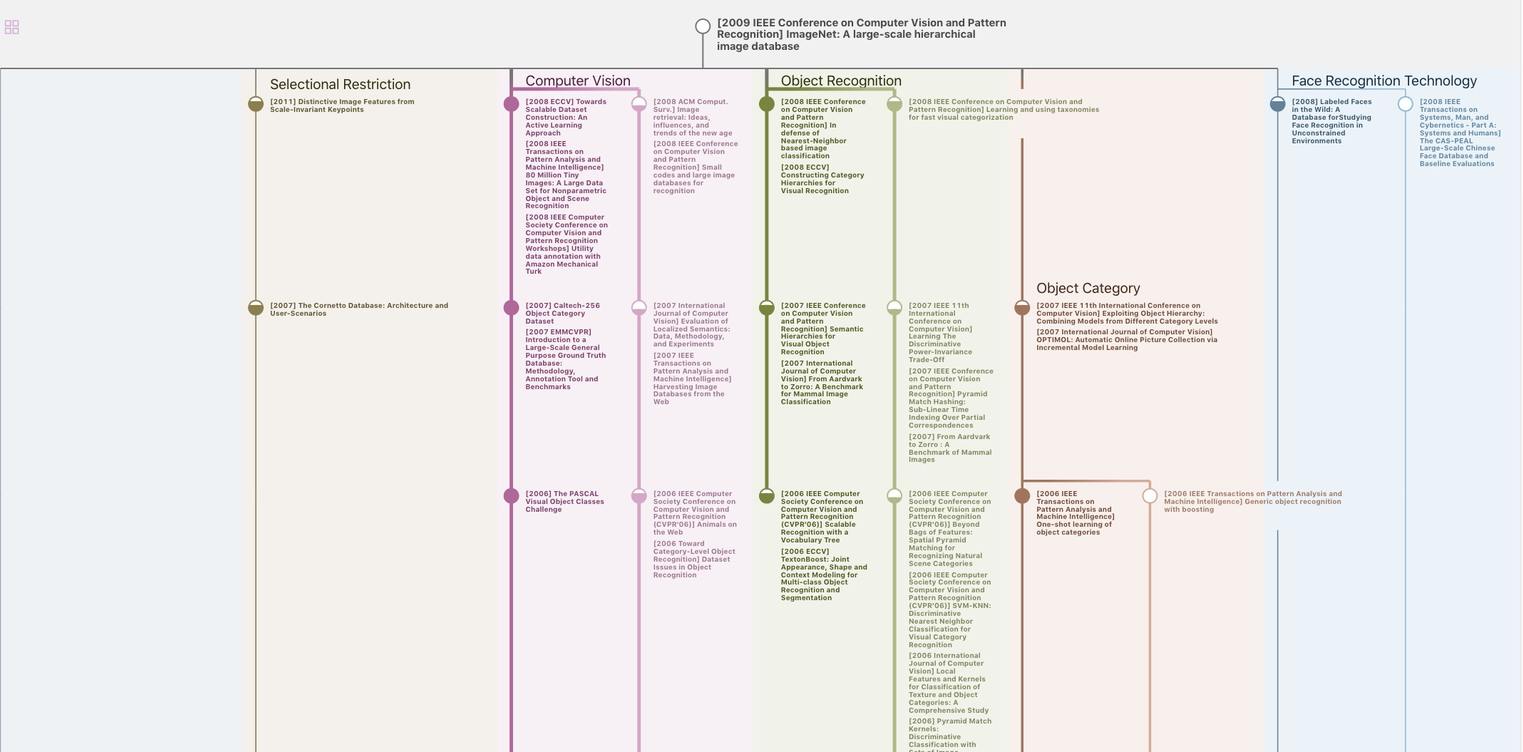
生成溯源树,研究论文发展脉络
Chat Paper
正在生成论文摘要