The study of hydraulic machinery condition monitoring based on anomaly detection and fault diagnosis
Measurement(2024)
摘要
The existing intelligent monitoring methods are hard to apply directly to hydraulic machinery due to fault data collection difficulties and the uncertainty of fault occurrence. To monitor the hydraulic machinery effectively, we design a novel feature extraction method called TFF_VMD and propose a comprehensive framework based on support vector data description (SVDD) and support vector machine (SVM), dividing the monitoring task into anomaly detection and fault identification. A pump test bench is built for data collection and method validation. Meanwhile, one online bearing dataset is used to pre-train SVM. The proposed method achieves satisfying results on three datasets, getting the accuracy of anomaly detection at 0.891, 0.999, and 0.995, respectively, getting mean and standard deviation of fault diagnosis accuracy at 0.954 ± 0.05, 0.96 ± 0.04, and 0.98 ± 0.03. Results show that TFF_VMD is valuable for SVDD and SVM, and the proposed framework can preliminarily achieve early warning and diagnosis of mechanical and hydraulic faults.
更多查看译文
关键词
Hydraulic machinery,Feature extraction,Condition monitoring,Support vector data description,Support vector machine
AI 理解论文
溯源树
样例
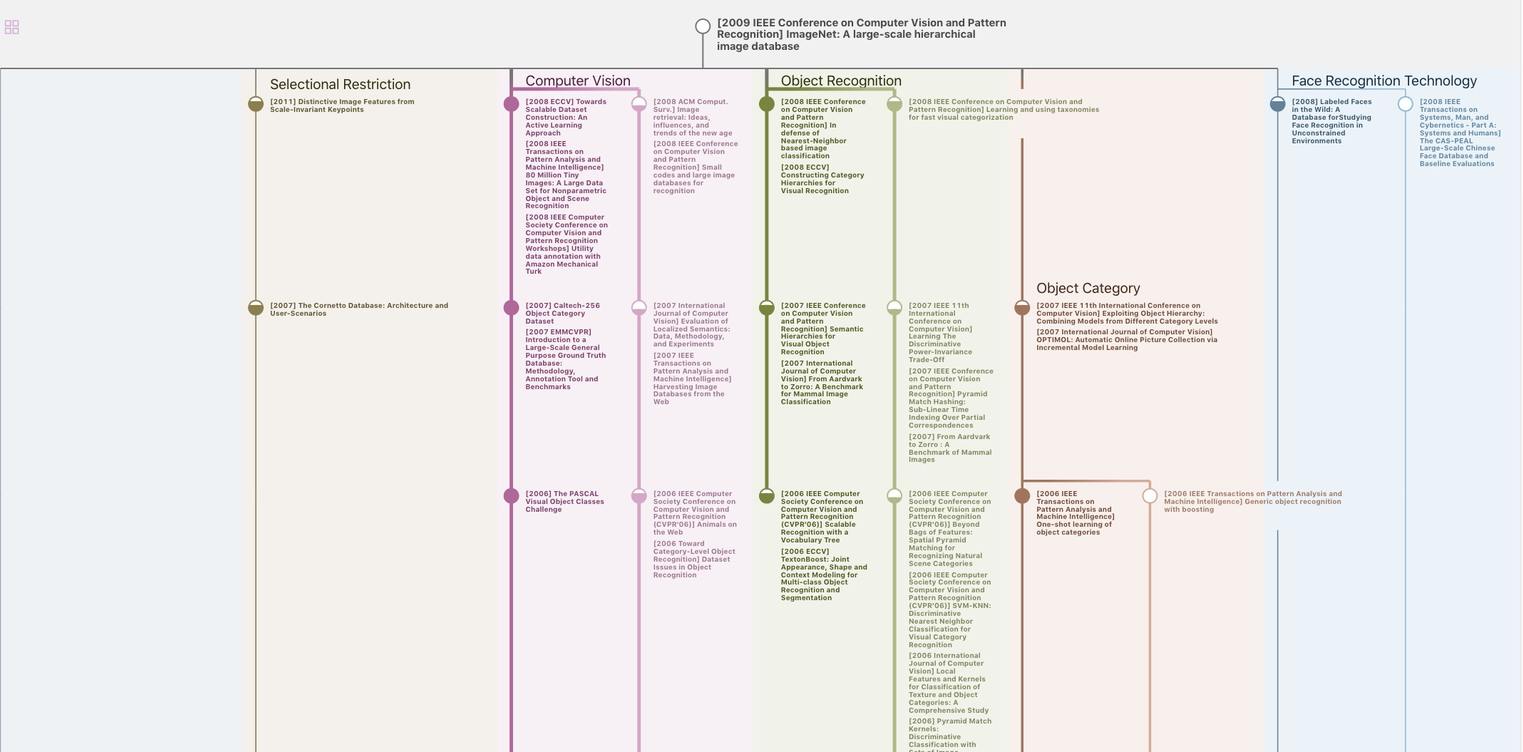
生成溯源树,研究论文发展脉络
Chat Paper
正在生成论文摘要