Data-unbalanced traffic accident prediction via adaptive graph and self-supervised learning
Applied Soft Computing(2024)
摘要
Traffic accident prediction is an important research problem, which can help to identify dangerous situations on the road in advance and take appropriate measures. Nonetheless, real-world traffic accident data suffers from a significant data unbalance problem, as accident occurrences vary unevenly in both spatial and data domains. This unbalance can easily lead to the prediction methods biased towards the side with more data. Recently, researchers have proposed a series of effective prediction methods based on deep learning and graph theory. Existing graph-based methods always adopt the predefined distance graph. However, these methods cannot fully capture the spatial correlations among regions that are far away from each other but share similar accident patterns. To address these challenges, we propose a traffic accident prediction method that combines Adaptive Graphs with Self-Supervised Learning (AGSSL). In the proposed method, we can adaptively construct graph structures to learn global spatial correlations among urban regions. Meanwhile, two self-supervised learning modules called Graph Infomax and Focal Contrastive Regularization are used to learn a robust representation of traffic accidents data under an unbalanced distribution. Experiment results show that AGSSL outperforms SOTA methods in traffic accident prediction.
更多查看译文
关键词
Traffic accident prediction,Unbalanced data classification,Self-supervised learning
AI 理解论文
溯源树
样例
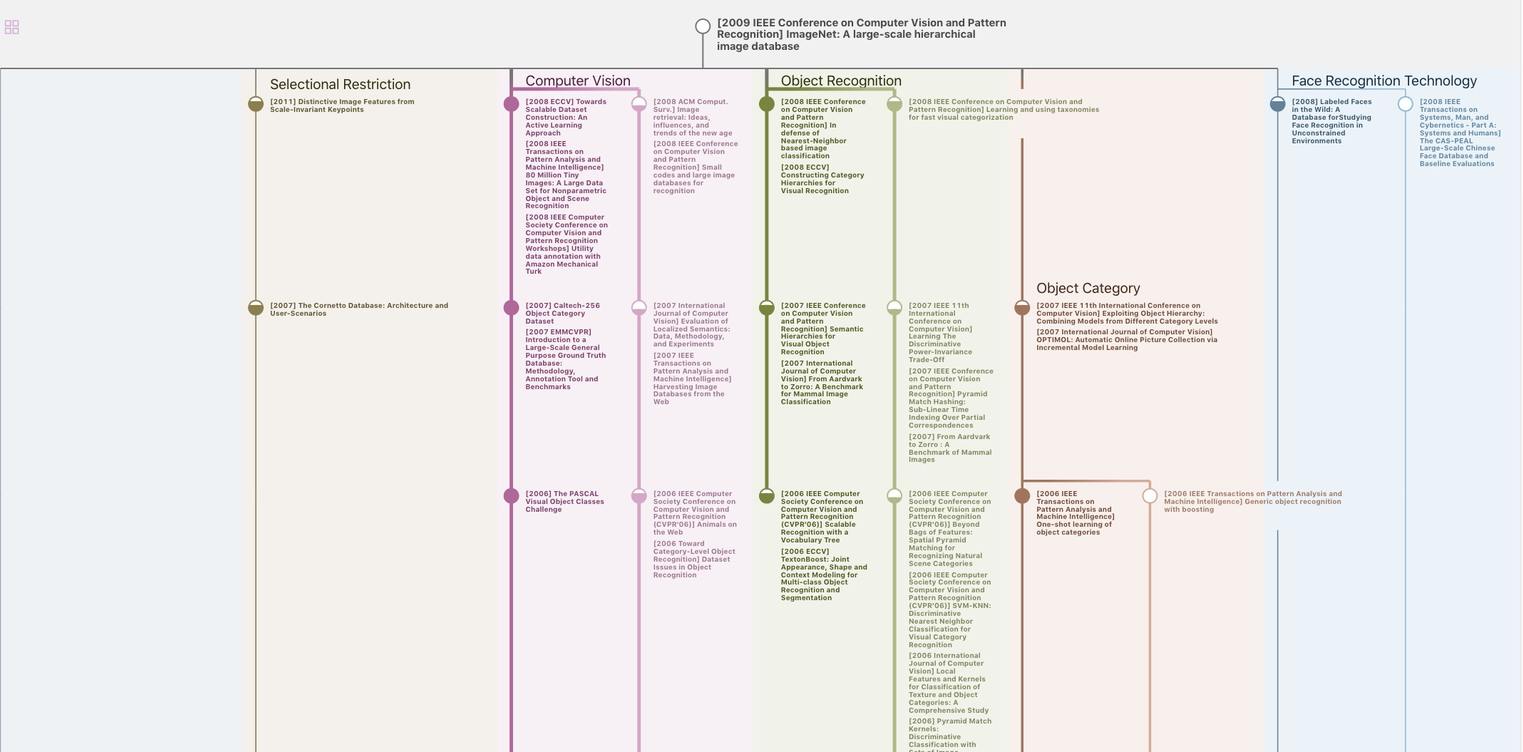
生成溯源树,研究论文发展脉络
Chat Paper
正在生成论文摘要