Multimodal prediction of student performance: A fusion of signed Graph Neural Networks and Large Language Models
Pattern Recognition Letters(2024)
摘要
In online learning platforms, accurately predicting student performance is essential for timely dropout prevention and interventions for at-risk students. Despite the widespread use of Multiple-Choice Questions (MCQs) in learnersourcing platforms for engagement, the accurate prediction of student performance remains challenging due to noise in student-generated content and existing graph-based representations’ inability to encode the semantic difference between a correct and wrong response. Addressing these limitations, we introduce the Large Language Model enhanced Signed Bipartite graph Contrastive Learning (LLM-SBCL) model—a novel Multimodal Model utilizing Signed Graph Neural Networks (SGNNs) and a Large Language Model (LLM). Our model uses a signed bipartite graph to represent students’ answers, with positive and negative edges denoting correct and incorrect responses, respectively. To mitigate noise impact, we apply contrastive learning to the signed graphs and combine this with knowledge point embeddings from the LLM. Upon evaluating our model on five real-world datasets, it demonstrated superior accuracy and stability, exhibiting an average F1 improvement of 3.7% over the best baseline models.
更多查看译文
关键词
Signed network,Graph representations learning,Natural language processing,Multimodal
AI 理解论文
溯源树
样例
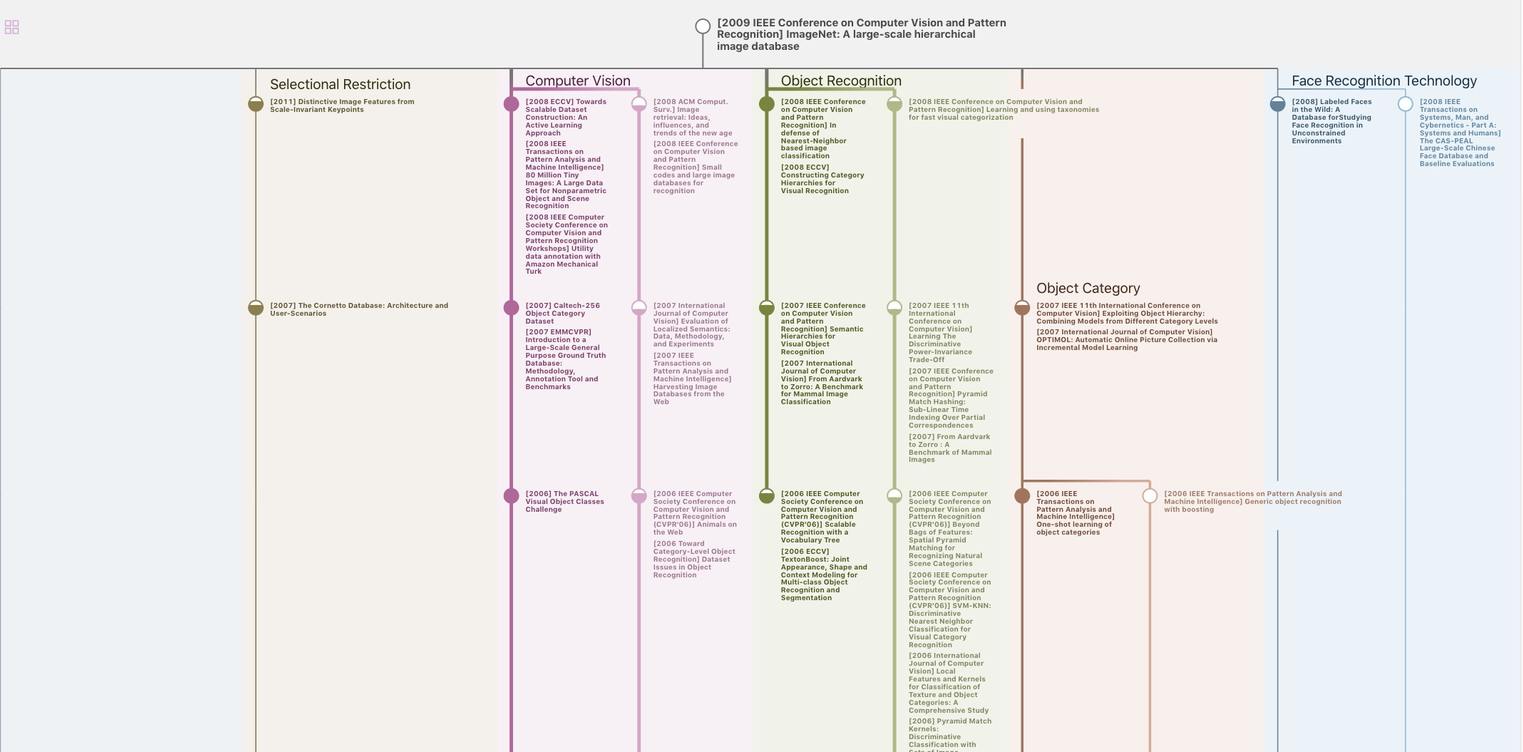
生成溯源树,研究论文发展脉络
Chat Paper
正在生成论文摘要