Federated learning survival model and potential radiotherapy decision support impact assessment for non-small cell lung cancer using real-world data
Clinical Oncology(2024)
摘要
Background
The objective of this study was to develop a two-year overall survival model for inoperable stage I-III non-small cell lung cancer (NSCLC) patients using routine radiation oncology data over a federated (distributed) learning network and evaluate the potential of decision support for curative versus palliative radiotherapy.
Methods
A federated infrastructure of data extraction, de-identification, standardization, image analysis, and modelling was installed for seven clinics to obtain clinical and imaging features and survival information for patients treated 2011-2019. A logistic regression model was trained for the 2011-2016 curative patient cohort and validated for the 2017-2019 cohort. Features were selected with univariate and model-based analysis and optimised using bootstrapping. System performance was assessed by the receiver operating characteristic (ROC) and corresponding area under curve (AUC), C-index, calibration metrics and Kaplan Meier survival curves with risk groups defined by model probability quartiles. Decision support was evaluated on a case-control analysis using propensity matching between treatment groups.
Results
1655 patient datasets were included. Overall model AUC was 0.68. Fifty-eight percent of patients treated with palliative radiotherapy had a low-to-moderate risk prediction according to the model, with survival times not significantly different (p=0.87 and 0.061) to patients treated with curative radiotherapy classified as high-risk by the model. When survival was simulated by risk group and model-indicated treatment there was an estimated 11% increase in survival rate at two years (p<0.01).
Conclusion
Federated learning over multiple institution data can be used to develop and validate decision support systems in lung cancer while quantifying potential impact of use in practice. This paves the way for personalized medicine where decisions can be based more closely on individual patient details from routine care.
更多查看译文
关键词
lung cancer,federated learning,machine learning,decision support,radiation oncology
AI 理解论文
溯源树
样例
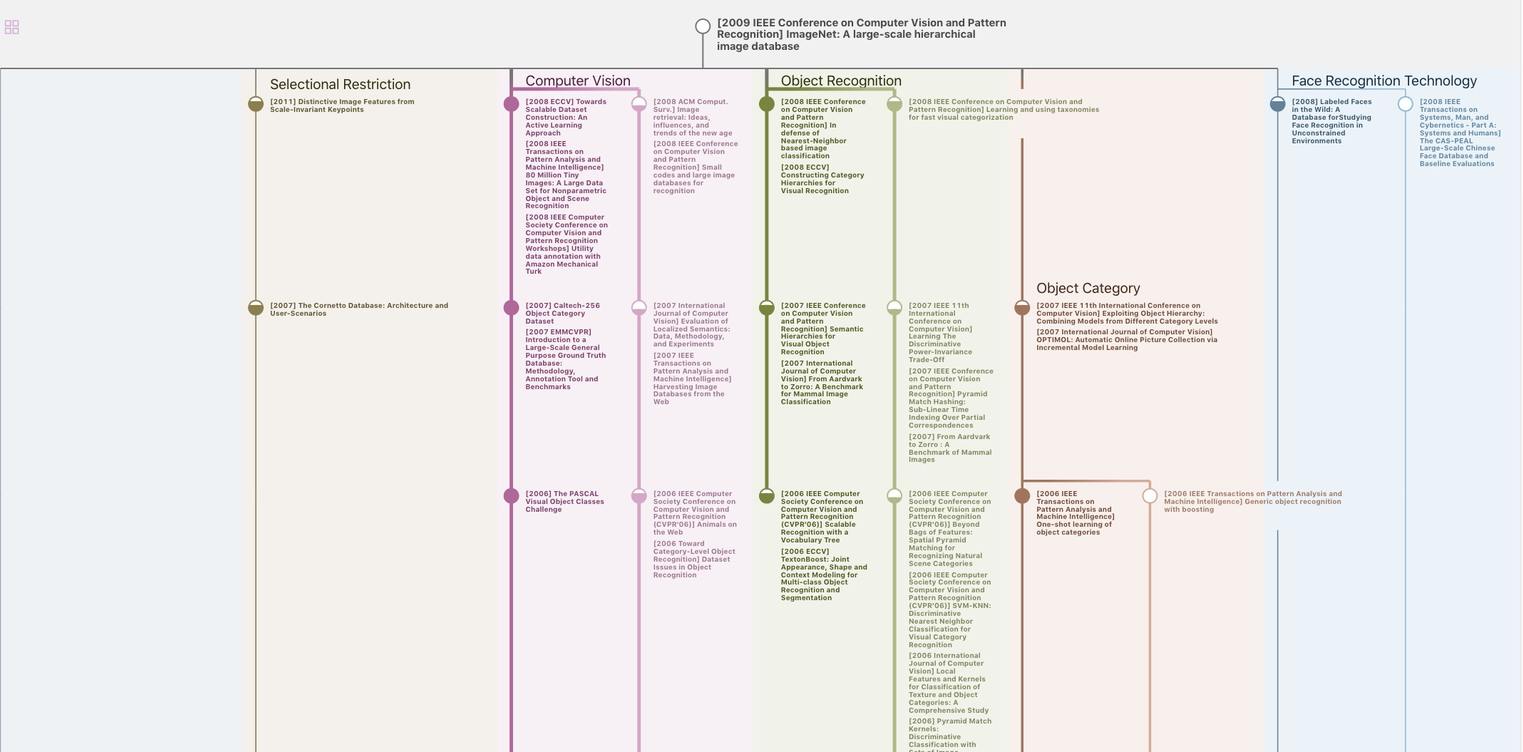
生成溯源树,研究论文发展脉络
Chat Paper
正在生成论文摘要