Machine learning-based gait health monitoring for multi-occupant smart homes
Internet of Things(2024)
摘要
Biomarkers related to gait are indicators for many diseases and overall well-being. It is important to monitor gait and its changes over time. The current standard of practice for gait assessments is performed in clinical settings, which does not accurately reflect gait patterns in daily life. Internet-of-Things technologies enable an opportunity to bridge this disparity in gait measurements by bringing sensors cost effectively into the home environment. A smart home system is presented that uses the vibrations in the floor naturally created by footfalls to extract gait health information from the residents. A signal processing approach is presented to detect and characterize footfalls in two-walker scenarios with a 94% success rate of correctly identifying an individual’s footfall. An adapted Gaussian mixture model was developed to estimate step time asymmetry with a root-mean-square error of 0.019s, or 3.4% of the average step time. A tracking machine learning method was used to estimate footfall locations with a root-mean-square error of 0.66 m. Lastly, a machine learning approach was implemented to estimate ground reaction forces with a root-mean-square error of 0.31g, or 9.1% of the average ground truth force. As an ambient continuous gait monitoring system, the proposed floor sensing system may be used to enable early detection and early intervention of gait abnormalities.
更多查看译文
关键词
Gait monitoring,Smart home,Signal processing,Machine Learning,Localization,Ground reaction force,Early intervention,Parkinson’s
AI 理解论文
溯源树
样例
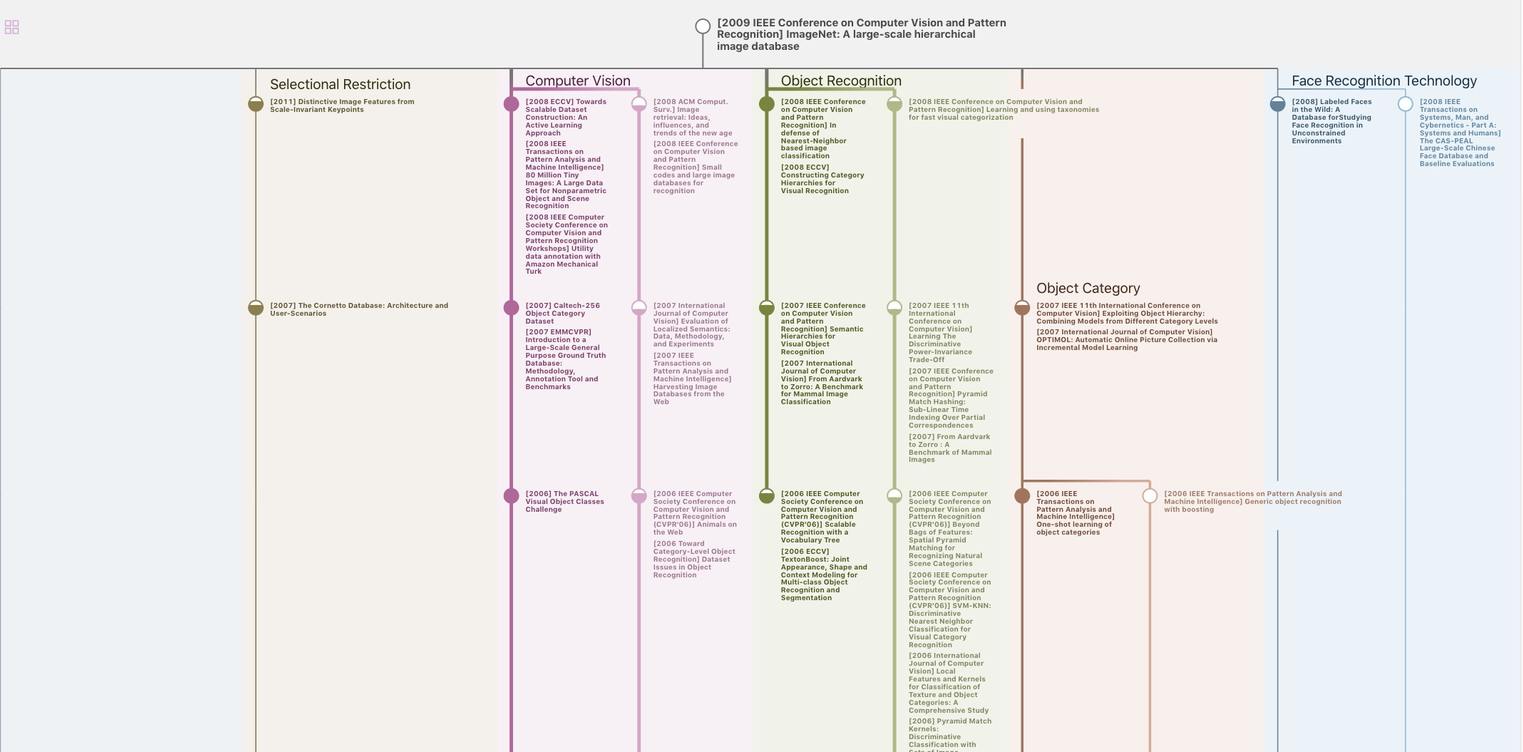
生成溯源树,研究论文发展脉络
Chat Paper
正在生成论文摘要