End-to-end point supervised object detection with low-level instance features
Applied Soft Computing(2024)
摘要
The use of point-level annotations in Point Supervision Object Detection (PSOD) has gained popularity due to its low labeling cost and the provision of specific location information. Previous studies have often relied on Multiple Instance Learning (MIL) to select and refine a low-quality pseudo box, which heavily relies on category information. This method concentrates on identifying the most distinctive objects in each category within an image. However, without the boundary constraints provided by Ground-Truth (GT), the detector may suffer from instance ambiguity problems, which can significantly deteriorate the model’s performance. To tackle these concerns, we propose an end-to-end object detection model called low-level instance features object detector (LIFOD) to mime low-level instance features information to refine the pseudo box. LIFOD utilizes a Low-level Feature Extractor (LFE) module to exploit low-level instance feature information to combine low-level feature information and category information, thus solving the challenge of instance ambiguity. To further alleviate the problem of local minima, we introduce bounding box regression in LIFOD and implement an end-to-end training strategy for point-supervised models to mitigate local minima. We evaluate our proposed LIFOD method on the public datasets COCO 2014 and 2017 and show that it outperforms the existing image-level and point-level supervised detection methods, and significantly reduces the gap in performance between PSOD and box-level object detection.
更多查看译文
关键词
End-to-end,Low-level instance features,Point supervision
AI 理解论文
溯源树
样例
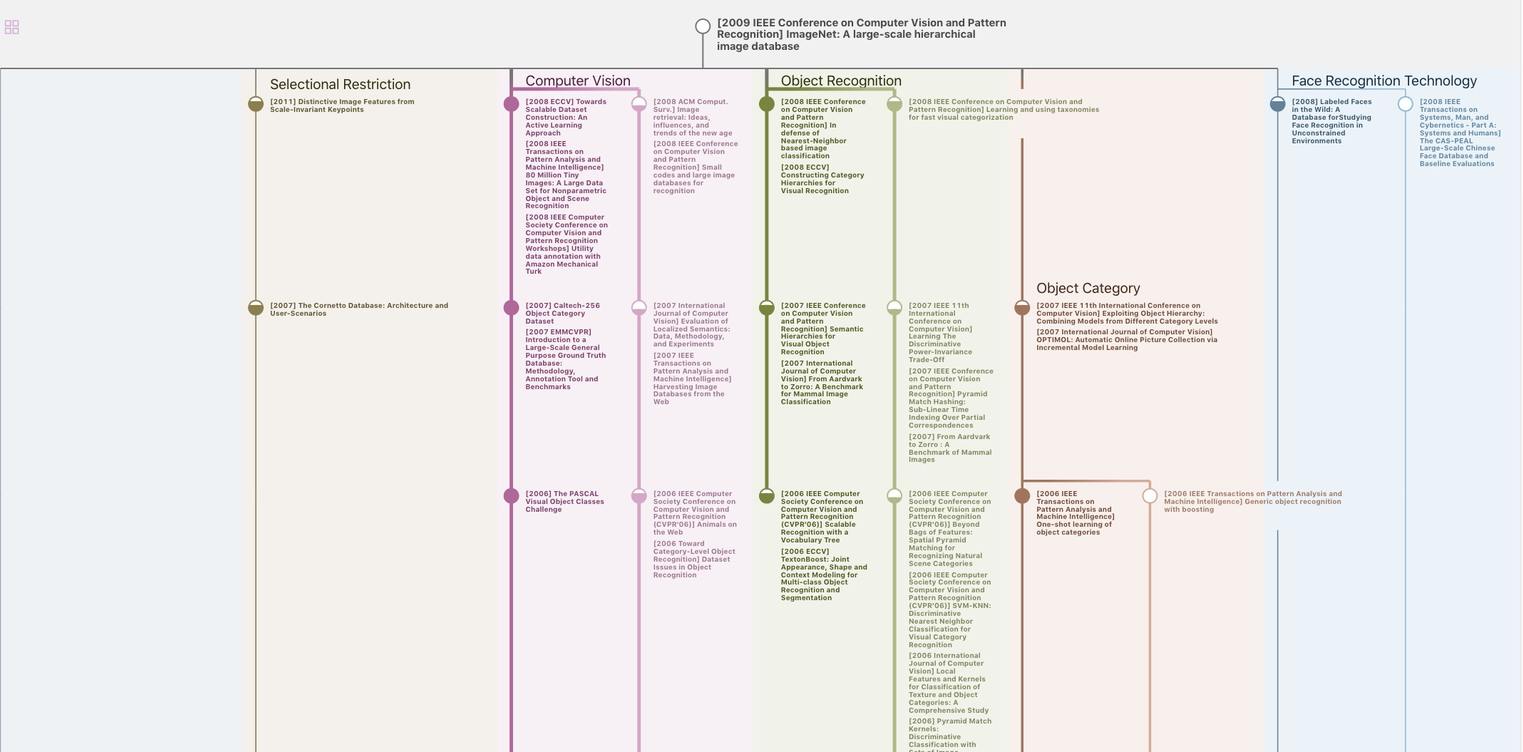
生成溯源树,研究论文发展脉络
Chat Paper
正在生成论文摘要