Prediction of adsorption of metal cations by clay minerals using machine learning
Science of The Total Environment(2024)
摘要
Adsorption of heavy metals by clay minerals occurs widely at the solid-liquid interface in natural environments, and in this paper, the phenomenon of adsorption of Cd2+, Cu2+, Pb2+, Zn2+, Ni2+ and Co2+ by montmorillonite, kaolinite and illite was simulated using machine learning. We firstly used six machine learning models including Random Forest(R), Extremely Forest(E), Gradient Boosting Decision Tree(G), Extreme Gradient Boosting(X), Light Gradient Boosting(LGB) and Category Boosting(CAT) to feature engineer the metal cations and the parameters of the minerals, and based on the feature engineering results, we determined the first order hydrolysis constant(log K), solubility product constant(SPC), and higher hydrolysis constant (HHC) as the descriptors of the metal cations, and site density(SD) and cation exchange capacity(CEC) as the descriptors of the clay minerals. After comparing the predictive effects of different data cleaning methods (pH50 method, Box method and pH50-Box method) and six model combinations, it was finally concluded that the best simulation results could be achieved by using the pH 50-Box method for data cleaning and Extreme Gradient Boosting for modelling (RMSE = 4.158 %, R2 = 0.977). Finally, model interpretation was carried out using Shapley explanation plot (SHAP) and partial dependence plot(PDP) to analyse the potential connection between each input variable and the output results. This study combines machine learning with geochemical analysis of the mechanism of heavy metal adsorption by clay minerals, which provides a different research perspective from the traditional surface complexation model.
更多查看译文
关键词
Clay minerals,Heavy metals,Feature engineering,Machine learning
AI 理解论文
溯源树
样例
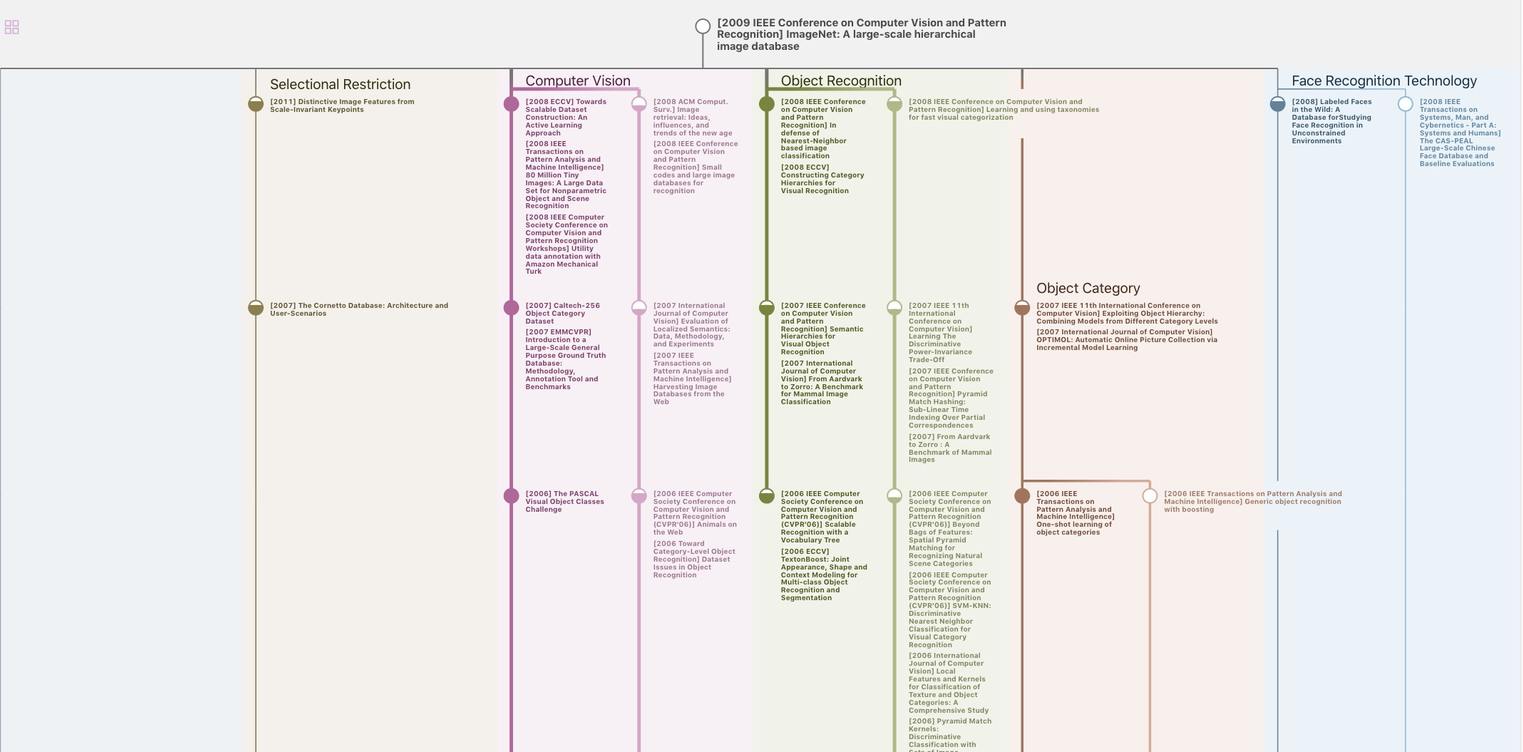
生成溯源树,研究论文发展脉络
Chat Paper
正在生成论文摘要