Time series retrieval of Multi-wavelength Aerosol optical depth by adapting Transformer (TMAT) using Himawari-8 AHI data
Remote Sensing of Environment(2024)
摘要
Accurate aerosol optical depth (AOD) with high temporal resolution is required for dynamic monitoring changes of aerosol properties. The Himawari-8 Advanced Himawari imager (AHI) data with 10-min temporal resolution has been widely used for AOD retrieval but usually involves radiative transfer models and assumptions on surface reflection and aerosol properties that are hard to satisfy. This paper introduces a data-driven algorithm without any priori assumptions on surface or aerosol properties called Time series retrieval of Multi-wavelength AOD by adapting Transformer (TMAT). TMAT directly retrieves AOD at eight-wavelengths every 10-min throughout the day, using AHI top of atmosphere (TOA) reflectances in 6 wavebands and ancillary data. TMAT explores diurnal temporal change patterns of AOD and surface reflection using time series data. TMAT was trained and evaluated using samples derived by matching a year (2019) of AHI full-disk data and AERONET/SONET observations with 12,768 diurnal time series, each of which includes up to 81 10-min 6-band AHI TOA observations and 81 (time) × 8 (wavelength) AOD values per day. There are up to 273,413 (<12,768 × 81) TOA observations in 6 wavebands and up to 1,420,716 (<12,768 × 81 × 8) AOD values in all the time series samples, with filled values (−9999) to represent no data due to cloud contamination and incomplete wavelength configurations of AOD stations. The masking mechanism in Transformer is leveraged to exclude the contribution of the AHI filled values in time series AOD retrieval and exclude the contribution of AOD filled values in training. TMAT was trained with a physical constraint that the retrieved AOD decreases toward longer wavelengths. TMAT AOD was evaluated by separating training and validation samples using site-specific leave-one-station-out validation. TMAT AOD shows extremely high accuracy at all wavelengths, with RMSE = 0.101, R = 0.949 and 81.69% of retrievals within ±0.05+15%∗AODsites for the AOD at 550 nm. We also validated the accuracy of the NASA multiangle implementation of atmospheric correction (MAIAC) AHI AOD over these sites with RMSE = 0.186, R = 0.832 and 52.10% of retrievals within ±0.05+15%∗AODsites. The Ångström exponent (AE) derived from the multi-wavelength TMAT AOD also shows good agreement with AERONET/SONET AE, with a correlation coefficient of 0.620 and an RMSE of 0.291. The TMAT effectiveness to capture diurnal temporal change patterns of surface reflection and AOD was evidenced by the significant reduction of AOD accuracy when using a non-time series deep learning method. The effectiveness of multi-wavelength joint retrieval was evidenced by the significant reduction of AE accuracy when using single wavelength retrieval models. Evidence shows the training and evaluation split methods have a large impact on evaluation accuracy and this study further used ground network independent training and evaluation split to confirm the leave-one-station-out validation accuracy. Despite this, future study will focus on more spatially independent validation and more comprehensive comparison between the MAIAC and TMAT results.
更多查看译文
关键词
Aerosol optical depth (AOD),Multi-wavelength,Himawari-8,Transformer,Time series retrieval
AI 理解论文
溯源树
样例
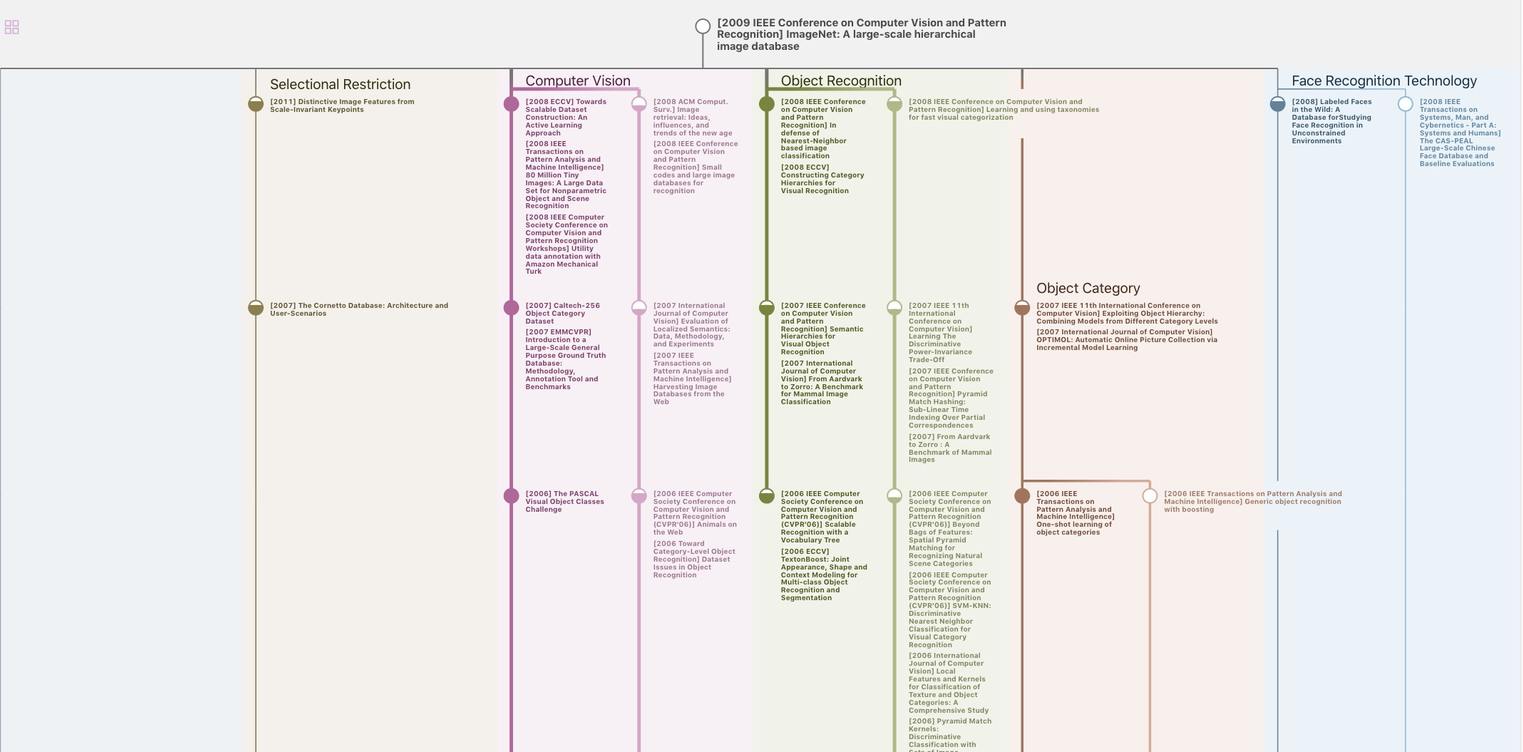
生成溯源树,研究论文发展脉络
Chat Paper
正在生成论文摘要