Reconstructing Computational Spectra Using Deep Learning's Self-Attention Method
crossref(2024)
摘要
Abstract Miniaturized computational spectrometers have become a new research hotspot due to their portability and miniaturization. However, there are several issues, like low precision and poor stability. Because the problem of spectrum reconstruction accuracy is very evident, we suggested a novel approach to raise the reconstruction accuracy. Using the time-domain finite-difference (FDTD) method, a library of transmittance functions was acquired. A cross-correlation algorithm was then used to choose 100 sparse transmittance functions, which were then built as an encoding matrix. Then, based on the encoding matrix, a self-attention mechanism algorithm was used to improve the accuracy of the reconstructed spectrum by improving the autocorrelation characteristics between input signals. The mean square error (MSE) of the reconstructed spectrum is 0.0019, and its similarity coefficient (R2) is 0.9780. This self-attention mechanism spectral reconstruction technique will open up new possibilities for high-accuracy reconstruction for a variety of computational spectrometer types.
更多查看译文
AI 理解论文
溯源树
样例
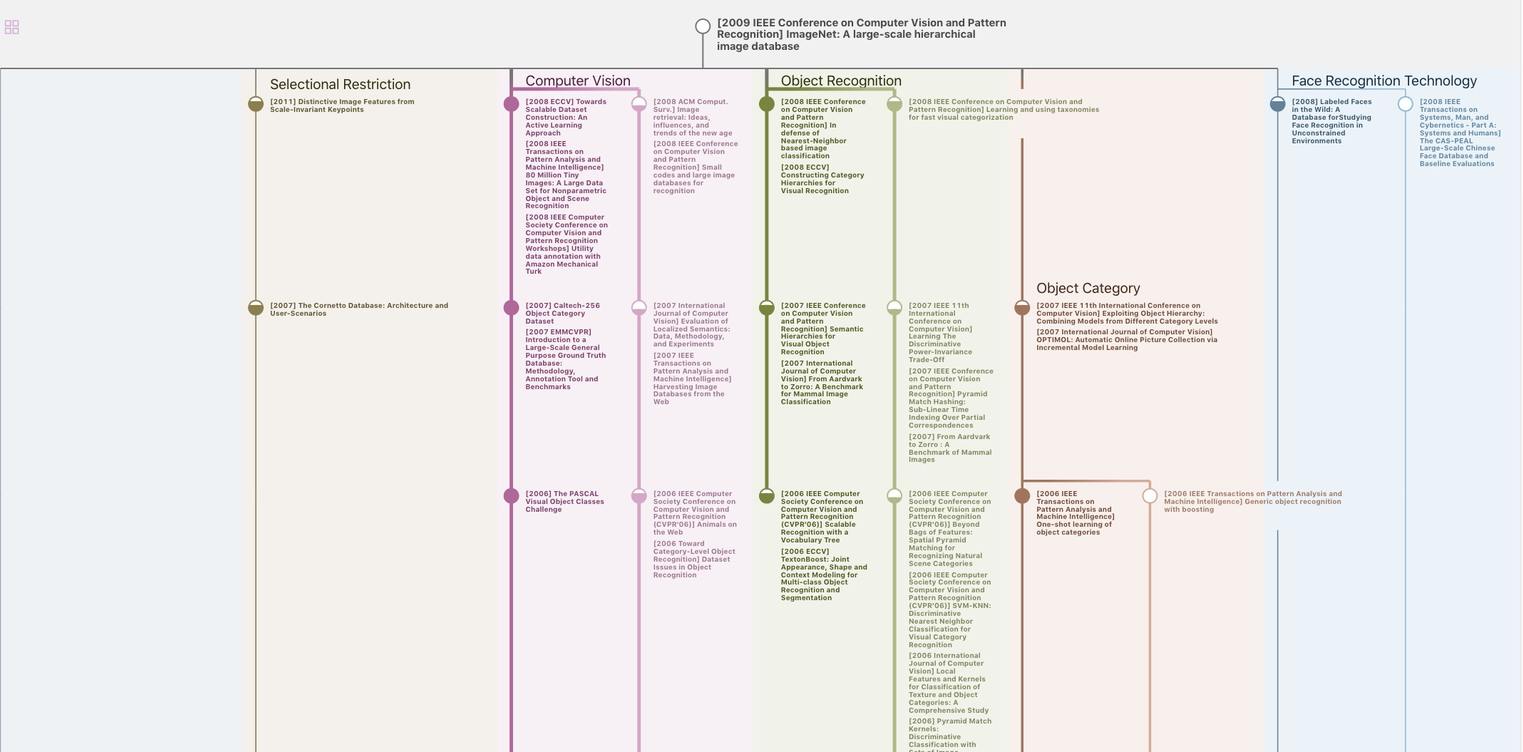
生成溯源树,研究论文发展脉络
Chat Paper
正在生成论文摘要