Automated learning data-driven potential models for spectroscopic characterization of astrophysical interest noble gas-containing NgH2+ molecules
Artificial Intelligence Chemistry(2024)
摘要
The choice of a proper machine learning (ML) algorithm for constructing potential energy surface (PES) models has become a crucial tool in the fields of quantum chemistry and computational modeling. These algorithms offer the ability to make reliable and accurate predictions at a reasonable computational cost, and thus they can be then used in various molecular dynamics and spectroscopic studies. For that, it is not surprising that much of the current research focuses on the development of software that generates machine learning models using precalculated ab initio data points. This study is primarily dedicated to the application and assessment of various automated learning models. These models are trained and tested using datasets derived from CCSD(T)/CBS[56] calculations, aiming to represent intermolecular interactions in small molecules, such as the NgH2+ complexes, where Ng represents helium (He), neon (Ne), and argon (Ar) atoms. These noble gas-containing molecules have gained increasing significance in the field of molecular astrochemistry, due to the recent discovery of HeH+ and ArH+ molecular cations in the interstellar medium (ISM), thereby opening up a wide range of possibilities in this scientific area. Consequently, the ML-generated PESs are employed to compute vibrational bound states for these molecular cations, with the goal of characterizing all their known isotopologues. Furthermore, the results are compared with spectroscopic data, when available, from previous studies in the literature. Our findings have the potential to provide valuable guidance for future ML-PES development and benchmarking studies involving noble gas-containing cations of astrophysical importance.
更多查看译文
关键词
Noble gas hydrides molecules,Theoretical quantum chemistry calculations,Electronic structure calculations,Intermolecular potentials,Machine-learning potential energy surfaces,Molecular spectroscopic constants,Computational vibrational spectroscopy
AI 理解论文
溯源树
样例
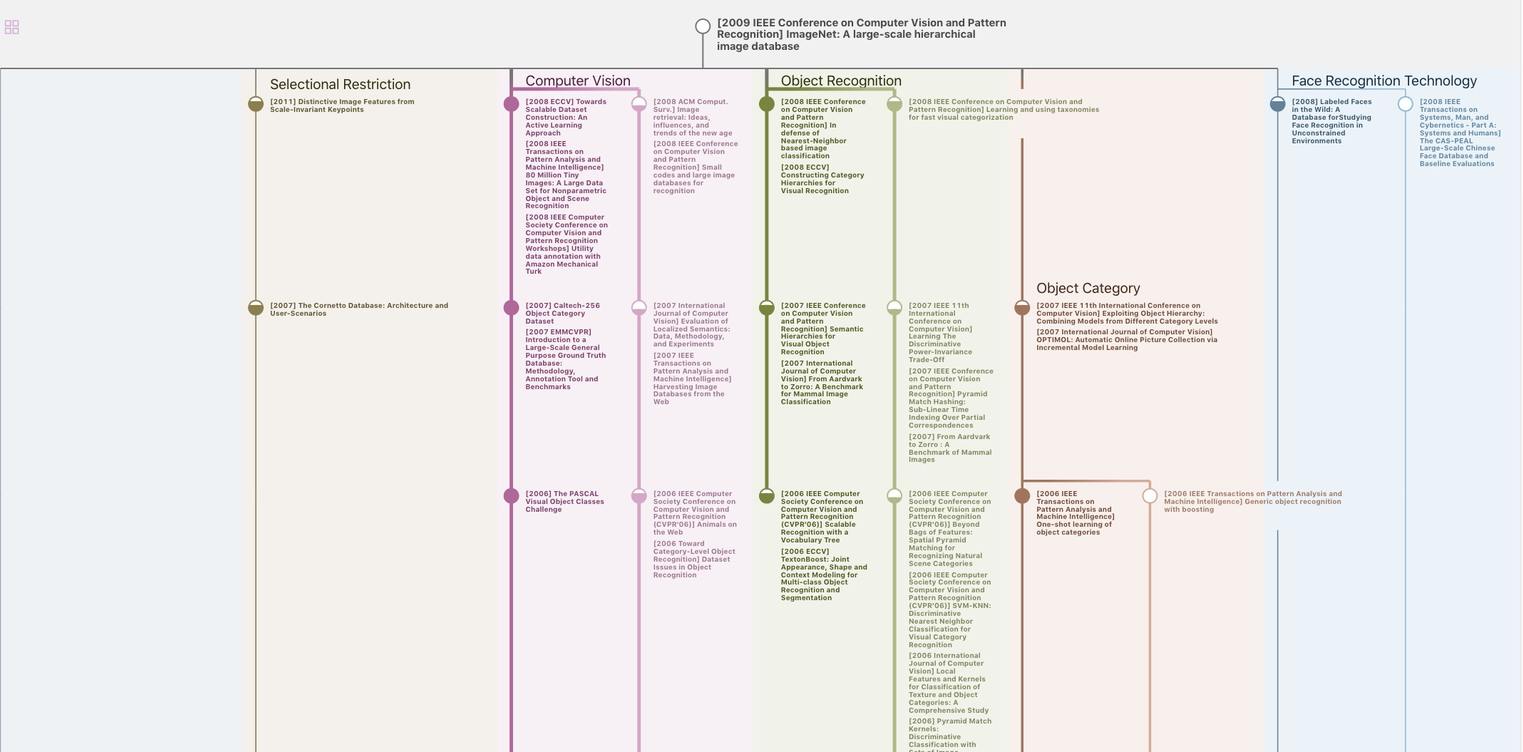
生成溯源树,研究论文发展脉络
Chat Paper
正在生成论文摘要