Towards an adaptive algorithms selection on predicting the update interval for social media feeds
PROCEEDINGS OF THE 2023 IEEE/ACM INTERNATIONAL CONFERENCE ON ADVANCES IN SOCIAL NETWORKS ANALYSIS AND MINING, ASONAM 2023(2023)
摘要
Efficiently synchronizing data with external sources, such as social media feeds, while minimizing well-timed requests is a challenge in various domains. This research investigates prediction algorithms for determining appropriate update intervals for Facebook and Twitter feeds, considering metrics such as the delay (time between a post's publication and retrieval) and requests per post. Due to variations in update intervals, different algorithms yield diverse results. Selecting the most suitable algorithm for each feed is a time-consuming but crucial task for achieving optimal resource usage. We propose three strategies for algorithm selection: baseline (using a single algorithm per feed), optimum (calculating the best algorithm for each feed), and classification (identifying algorithms through classification). Real-world data from Facebook and Twitter are used to evaluate the strategies, comprehensively assessing their strengths and weaknesses. Findings demonstrate that the strategy optimum identifies the best algorithms, while the strategy classification selects fairly good algorithms at significantly reduced computational effort.
更多查看译文
AI 理解论文
溯源树
样例
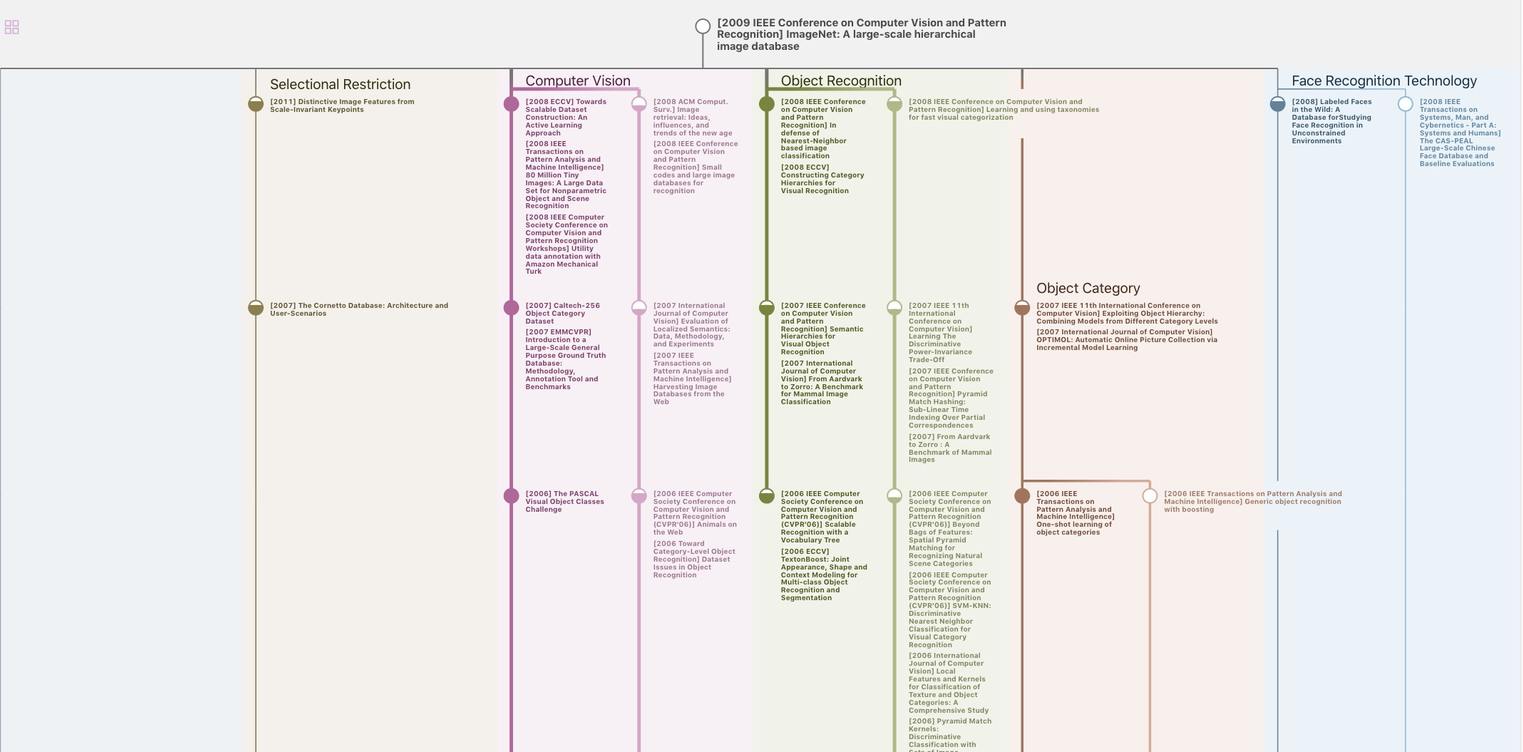
生成溯源树,研究论文发展脉络
Chat Paper
正在生成论文摘要