Quantifying the Transience of Social Web Datasets
PROCEEDINGS OF THE 2023 IEEE/ACM INTERNATIONAL CONFERENCE ON ADVANCES IN SOCIAL NETWORKS ANALYSIS AND MINING, ASONAM 2023(2023)
摘要
The social web presents a modern-day instrument to analyze a wide range of behavioral research questions. Of these platforms, Twitter has played a key role in social science research for more than a decade. This paper looks into an underexplored aspect - transience of Twitter datasets and makes the following three contributions. First, via a comprehensive investigation of more than 40 Twitter datasets, we identify that many of these datasets suffer from severe retrieval loss. Second, we demonstrate that the retrieval loss across labels is often imbalanced with inappropriate labels (e.g., misinformation, hate speech) suffering from more retrieval loss. Finally, we demonstrate that imbalanced retrieval loss may impact machine learning models differently than balanced retrieval loss.
更多查看译文
关键词
Quantifying Dataset Transience,Quantifying Retrieval Imbalance,Social Web
AI 理解论文
溯源树
样例
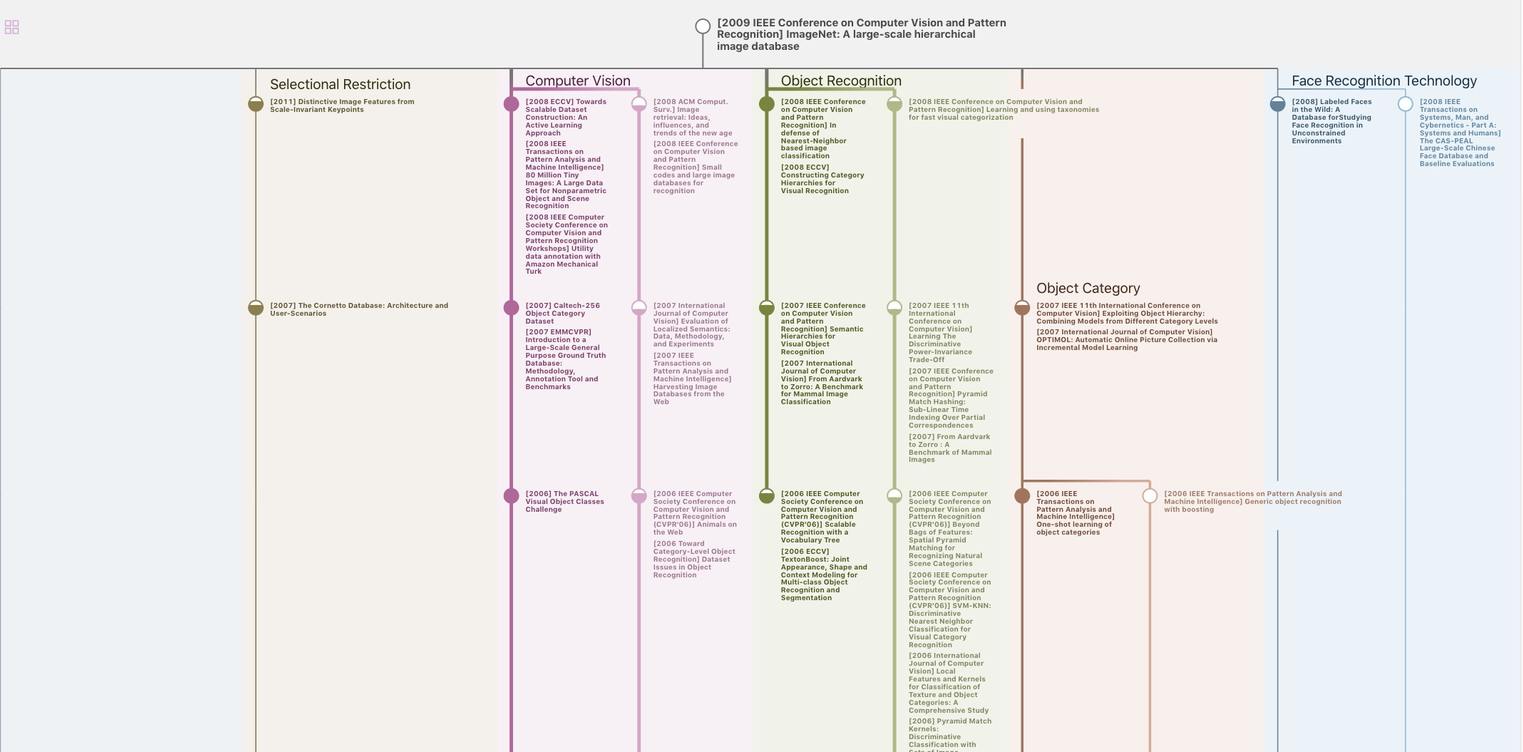
生成溯源树,研究论文发展脉络
Chat Paper
正在生成论文摘要