396 Creating a High-Precision, Dynamic Machine Learning Model Using Time Series Data to Predict Cerebral Vasospsm in Aneurysmal Subarachnoid Hemorrhage
Neurosurgery(2024)
摘要
INTRODUCTION: Cerebral vasospasm (CV) occurs in up to one-third of patients who suffer from aneurysmal subarachnoid hemorrhage (aSAH) and can result in significant morbidity. Prior attempts to predict CV after aSAH have failed because they use static data collected on the day of presentation. We hypothesize that trending clinical and radiographic data including transcranial dopplers (TCDs) throughout 14 intensive care unit (ICU) days can be used to create a highly predictive, dynamic machine learning model for CV. METHODS: We conducted an IRB-approved retrospective study including 428 patients with aSAH hemorrhage treated at our institution between June 2014 and December 2018. For each patient, 74 features were trended including vital signs, lab values and ventriculostomy output. TCD measurements included anterior and posterior circulation velocities, Lindegaard Ratios and pulsatility indices. We employed a generative transformer-based model that uses patient representations from aggregate prior time points to predict the likelihood of CV. RESULTS: Of the 428 aSAH patients, 85 had angiographic-proven CV. There was no difference in demographic features including age, gender distribution and BMI between the CV and no-CV groups. Throughout 14 ICU days, the CV patients consistently displayed elevated mean arterial pressure and cerebral perfusion pressure. All TCD measurements were higher for the CV group. Initial logistic regression models based on static data from post-ictus days 1 through 4 showed steadily increasing F1 scores from 0.67 to 0.76. Feature analysis identified Lindegaard Ratios as the most heavily weighted features. CONCLUSIONS: Daily TCDs paired with additional longitudinal clinical data can be used to create a high-precision dynamic machine learning model for predicting CV after aSAH. These methods could also permit identification of patients at low risk of CV for reduced ICU length of stay.
更多查看译文
AI 理解论文
溯源树
样例
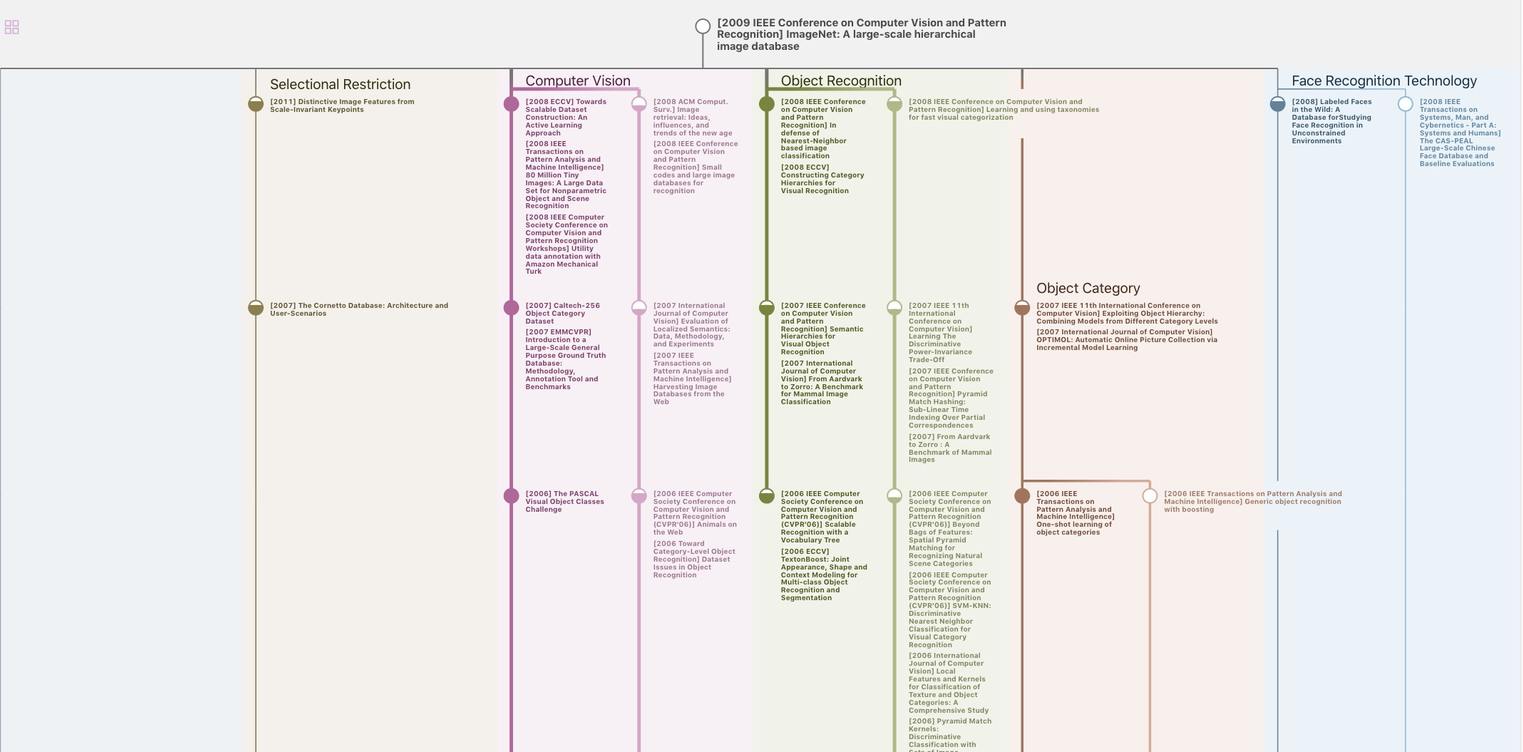
生成溯源树,研究论文发展脉络
Chat Paper
正在生成论文摘要