Switching Self-Attention Text Classification Model with Innovative Reverse Positional Encoding for Right-to-Left Languages: A Focus on Arabic Dialects
MATHEMATICS(2024)
摘要
Transformer models have emerged as frontrunners in the field of natural language processing, primarily due to their adept use of self-attention mechanisms to grasp the semantic linkages between words in sequences. Despite their strengths, these models often face challenges in single-task learning scenarios, particularly when it comes to delivering top-notch performance and crafting strong latent feature representations. This challenge is more pronounced in the context of smaller datasets and is particularly acute for under-resourced languages such as Arabic. In light of these challenges, this study introduces a novel methodology for text classification of Arabic texts. This method harnesses the newly developed Reverse Positional Encoding (RPE) technique. It adopts an inductive-transfer learning (ITL) framework combined with a switching self-attention shared encoder, thereby increasing the model's adaptability and improving its sentence representation accuracy. The integration of Mixture of Experts (MoE) and RPE techniques empowers the model to process longer sequences more effectively. This enhancement is notably beneficial for Arabic text classification, adeptly supporting both the intricate five-point and the simpler ternary classification tasks. The empirical evidence points to its outstanding performance, achieving accuracy rates of 87.20% for the HARD dataset, 72.17% for the BRAD dataset, and 86.89% for the LABR dataset, as evidenced by the assessments conducted on these datasets.
更多查看译文
关键词
switching self-attention,reverse positional encoding (RPE) mothed,text classification (TC),right-to-left text,five-polarity,ITL
AI 理解论文
溯源树
样例
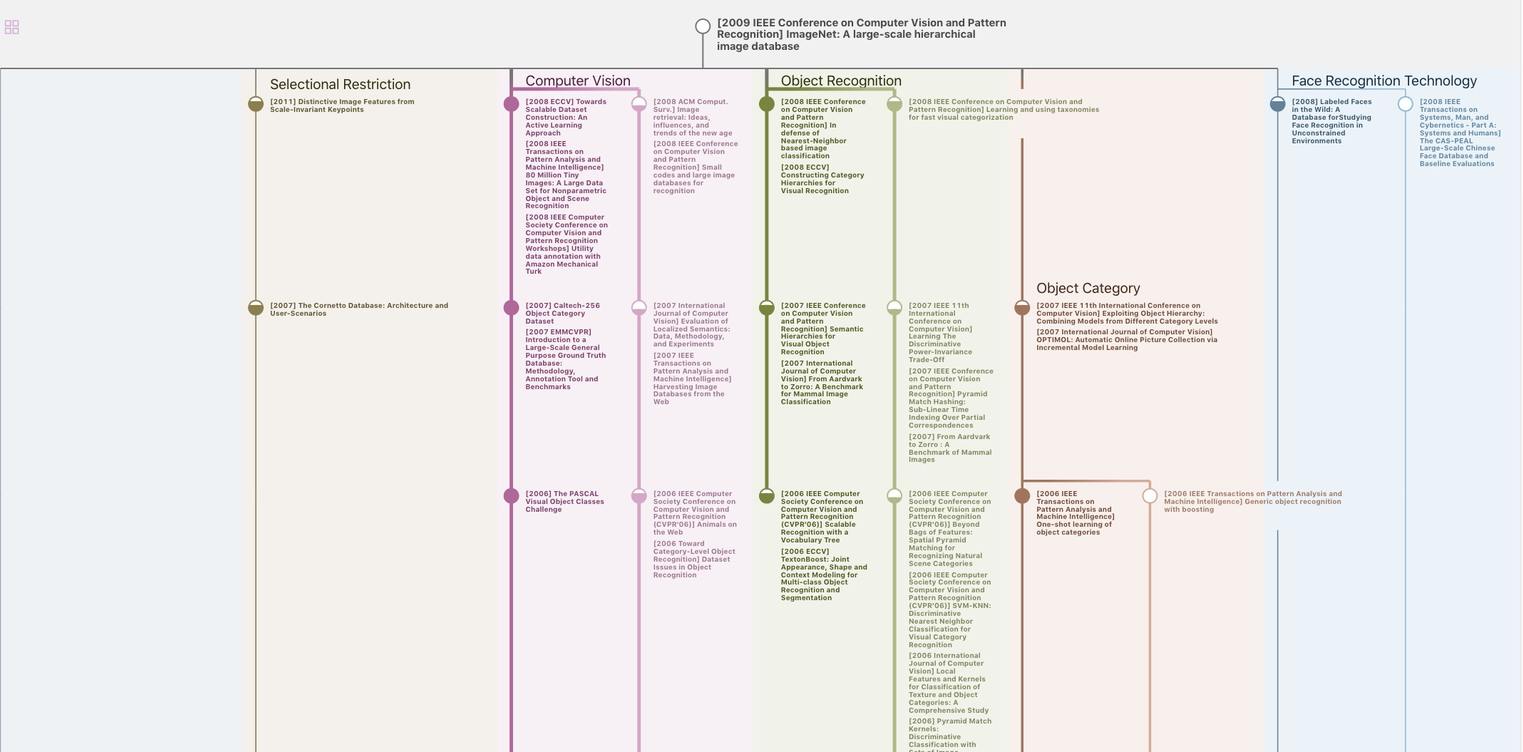
生成溯源树,研究论文发展脉络
Chat Paper
正在生成论文摘要