Online concept evolution detection based on active learning
Data Mining and Knowledge Discovery(2024)
摘要
Concept evolution detection is an important and difficult problem in streaming data mining. When the labeled samples in streaming data insufficient to reflect the training data distribution, it will often further restrict the detection performance. This paper proposed a concept evolution detection method based on active learning (CE_AL). Firstly, the initial classifiers are constructed by a small number of labeled samples. The sample areas are divided into the automatic labeling and the active labeling areas according to the relationship between the classifiers of different categories. Secondly, for online new coming samples, according to their different areas, two strategies based on the automatic learning-based model labeling and active learning-based expert labeling are adopted respectively, which can improve the online learning performance with only a small number of labeled samples. Besides, the strategy of “data enhance” combined with “model enhance” is adopted to accelerate the convergence of the evolution category detection model. The experimental results show that the proposed CE_AL method can enhance the detection performance of concept evolution and realize efficient learning in an unstable environment by labeling a small number of key samples.
更多查看译文
关键词
Concept evolution,Active learning,CE_AL model,Model labeling,Expert labeling,Data enhance,Model enhance
AI 理解论文
溯源树
样例
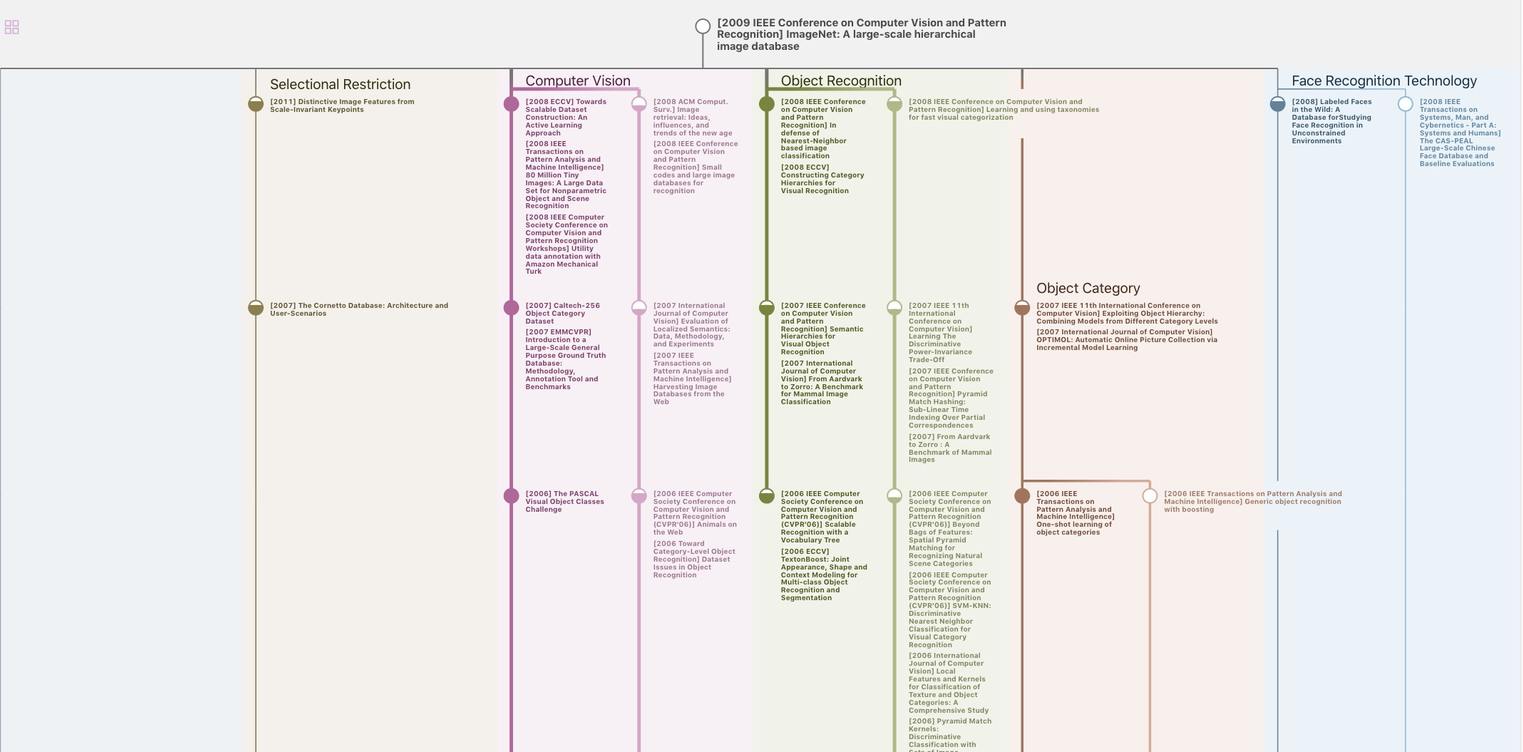
生成溯源树,研究论文发展脉络
Chat Paper
正在生成论文摘要