Zero-shot image classification via Visual–Semantic Feature Decoupling
Multimedia Systems(2024)
摘要
Zero-shot image classification refers to the use of labeled images to train a classification model that can correctly classify images of unseen categories. Traditional zero-shot methods use attribute labels as supervisory information and map the visual information of images to semantic space for classification. However, due to the modal heterogeneity between images and semantics, it is impossible to completely align visual and semantic information, resulting in information loss in visual–semantic mapping. In addition, zero-shot classification also faces domain drift problems caused by non-intersecting training and testing categories. Therefore, this paper proposes a zero-shot image classification method based on decoupling of visual–semantic features, which alleviates modal heterogeneity and domain drift problems by complementing modal information with each other. The proposed method first uses feature extractors with the same structure but different parameters to extract visual and semantic features separately; second, it uses an Attribute Correction Module (ACM) to weight-fuse the semantic features of seen classes and attribute labels to correct semantic supervisory information; then it uses an adaptive data distribution adjustment strategy to balance the discriminability and transferability of the model and alleviate domain drift problems; finally, it discards modality-independent information in visual and semantic features and recombines decoupled features for classification. Experimental results demonstrate the effectiveness and advancement of our method.
更多查看译文
关键词
Zero-Shot Learning,Image classification,Transfer learning,Feature decoupling,Attribute correction
AI 理解论文
溯源树
样例
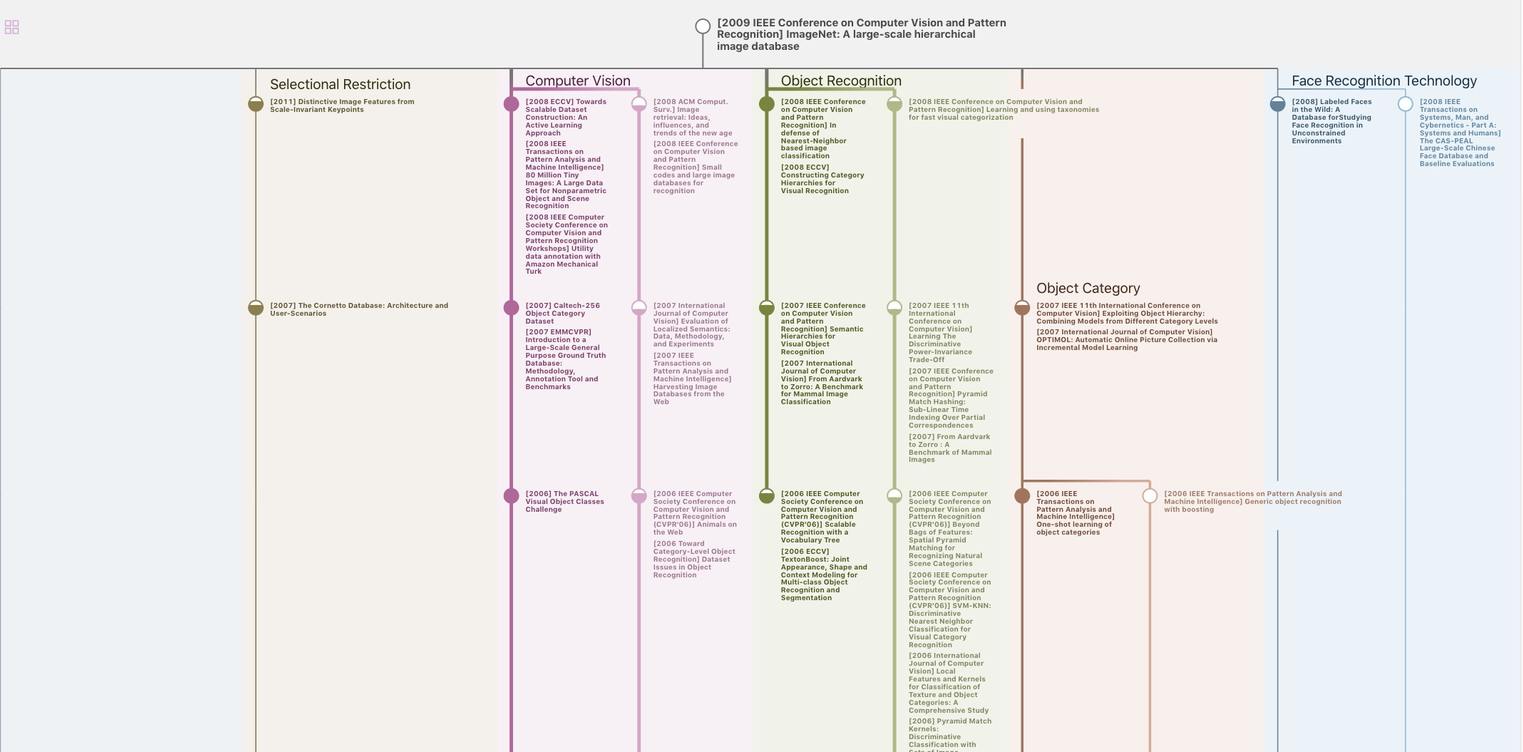
生成溯源树,研究论文发展脉络
Chat Paper
正在生成论文摘要