Person identification using autoencoder-CNN approach with multitask-based EEG biometric
Multimedia Tools and Applications(2024)
摘要
In this research paper, we propose an unsupervised framework for feature learning based on an autoencoder to learn sparse feature representations for EEG-based person identification. Autoencoder and CNN do the person identification task for signal reconstruction and recognition. Electroencephalography (EEG) based biometric system is vesting humans to recognize, identify and communicate with the outer world using brain signals for interactions. EEG-based biometrics are putting forward solutions because of their high-safety capabilities and handy transportable instruments. Motor imagery EEG (MI-EEG) is a maximum broadly centered EEG signal that exhibits a subject’s motion intentions without real actions. The Proposed framework proved to be a practical approach to managing the massive volume of EEG data and identifying the person based on their different task with resting states. The experiments have been conducted on the standard publicly available motor imagery EEG dataset with 109 subjects. The highest recognition rate of 87.60
更多查看译文
关键词
Electroencephalography (EEG),Biometric authentication,Person identification,Autoencoder,Convolutional neural network
AI 理解论文
溯源树
样例
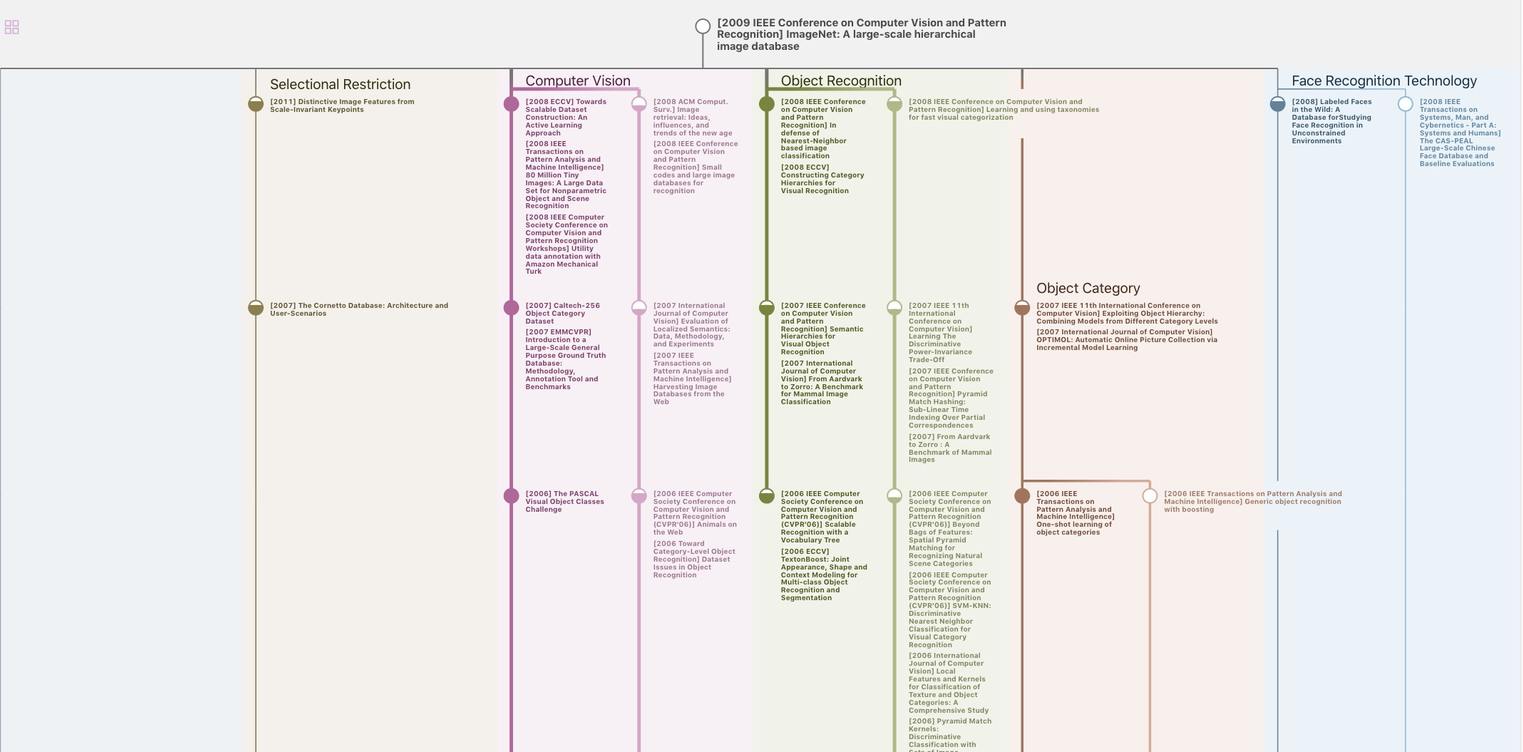
生成溯源树,研究论文发展脉络
Chat Paper
正在生成论文摘要