Learning evolving relations for multivariate time series forecasting
Applied Intelligence(2024)
摘要
Multivariate time series forecasting is essential in various fields, including healthcare and traffic management, but it is a challenging task due to the strong dynamics in both intra-channel relations (temporal patterns within individual variables) and inter-channel relations (the relationships between variables), which can evolve over time with abrupt changes. This paper proposes ERAN (Evolving Relational Attention Network), a framework for multivariate time series forecasting, that is capable to capture such dynamics of these relations. On the one hand, ERAN represents inter-channel relations with a graph which evolves over time, modeled using a recurrent neural network. On the other hand, ERAN represents the intra-channel relations using a temporal attentional convolution, which captures the local temporal dependencies adaptively with the input data. The elvoving graph structure and the temporal attentional convolution are intergrated in a unified model to capture both types of relations. The model is experimented on a large number of real-life datasets including traffic flows, energy consumption, and COVID-19 transmission data. The experimental results show a significant improvement over the state-of-the-art methods in multivariate time series forecasting particularly for non-stationary data.
更多查看译文
关键词
Time series forecasting,Multivariate time series forecasting,Dynamic graph neural networks,Attention mechanism
AI 理解论文
溯源树
样例
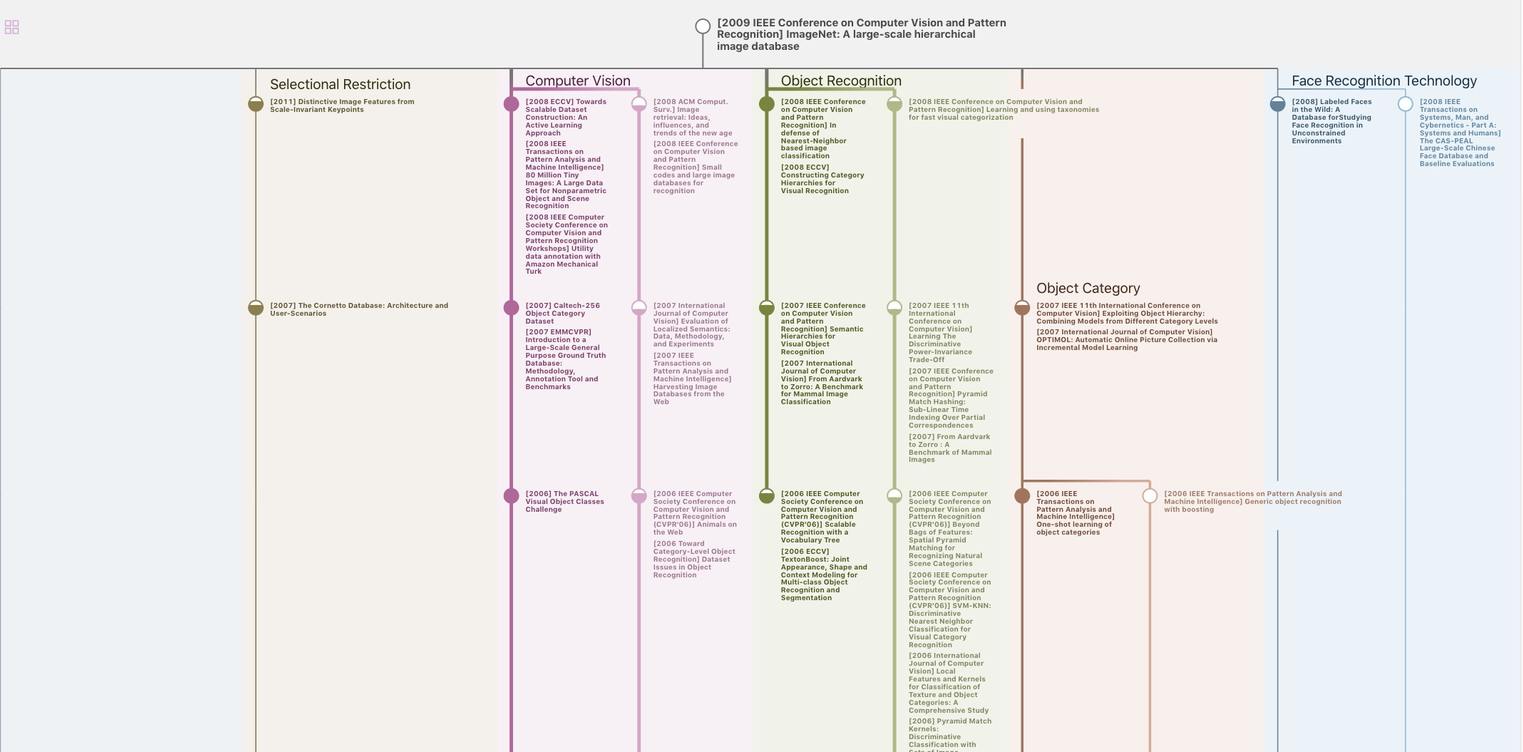
生成溯源树,研究论文发展脉络
Chat Paper
正在生成论文摘要