Automatic Fine-Grained BIM element classification using Multi-Modal deep learning (MMDL)
Advanced Engineering Informatics(2024)
摘要
In Building Information Modeling (BIM)-based domain-specific applications, elements should be classified into fine-grained sub-categories concerning their graphical and/or non-graphical characteristics to support downstream tasks. Traditional rule-based and machine learning methods are either time-consuming or cannot meet fine-grained classification requirements in domain-specific applications. To overcome this challenge, this paper presents a novel framework based on BIM and Multi-Modal Deep Learning (MMDL) for automatic fine-grained BIM element classification. It begins with transforming multi-modal (i.e., graphical and non-graphical) element features from BIM models. A feature selection algorithm is then designed to determine relevant BIM element features automatically. Subsequently, an MMDL model is developed and deployed to fuse the selected multi-modal BIM element features for end-to-end fine-grained classification. The framework is validated with a BIM element classification dataset. The results show that fine-grained elements can be classified with high accuracy (over 98%) in an end-to-end manner, which is unattainable by other BIM element classification methods.
更多查看译文
关键词
Building information modeling,Multi-modal deep learning,Feature selection,BIM data fusion,Classification,Interoperability
AI 理解论文
溯源树
样例
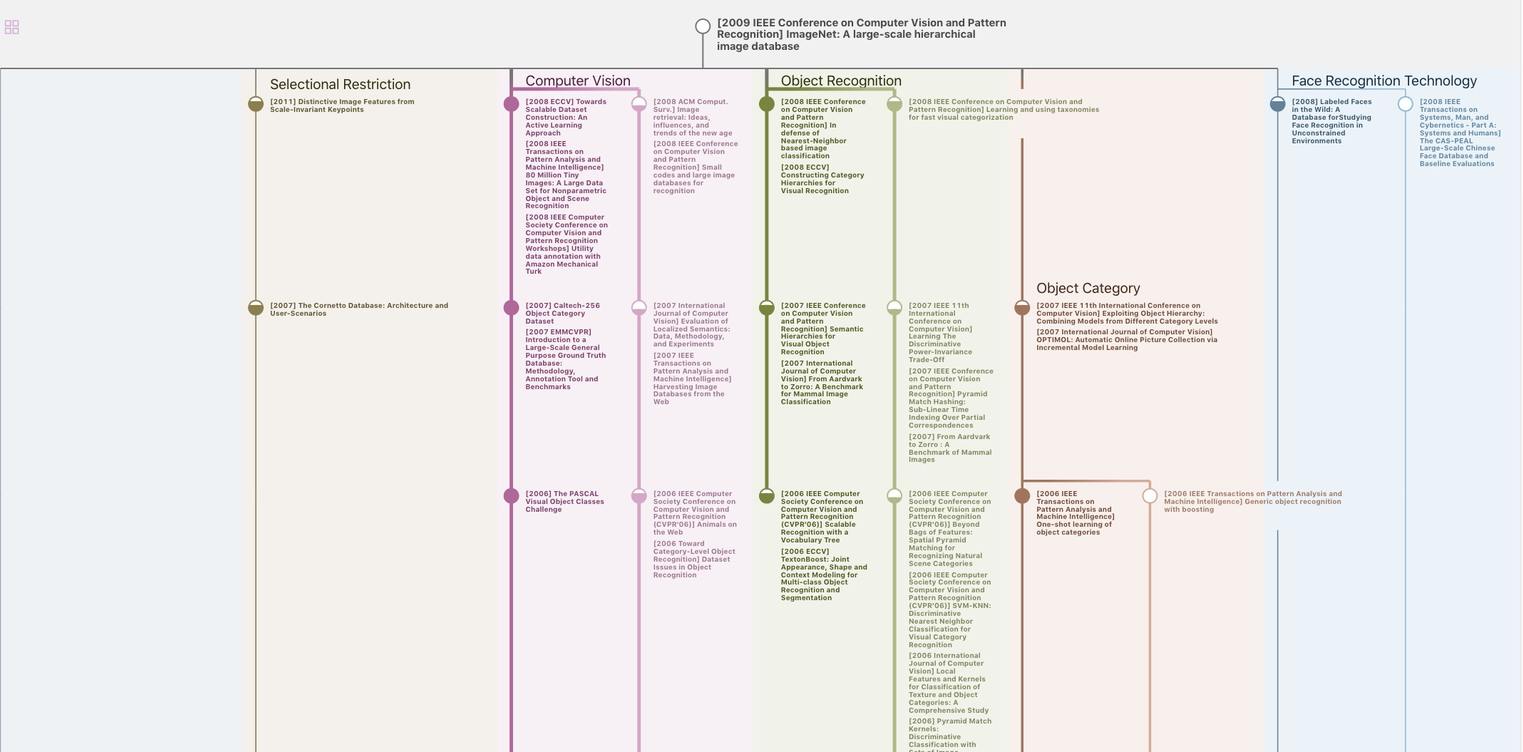
生成溯源树,研究论文发展脉络
Chat Paper
正在生成论文摘要