Concrete forensic analysis using deep learning-based coarse aggregate segmentation
AUTOMATION IN CONSTRUCTION(2024)
摘要
This study proposes an innovative and field-friendly concrete forensic analysis technique. State -of -the -art encoder-decoder models are developed, trained and evaluated on a self-curated standard image dataset for segmenting gray-colored coarse aggregates within the same-colored concrete components. Contrary to existing practices, no color treatment or microscopy images are used to facilitate segmentation. A vision transformerbased (SwinTV2 + Segformer) model achieves the highest mIoU of 94.7%, surpassing the best-performing convolutional neural network-based (EfficientNetV2 + DeepLabV3+) model. Using segmented aggregate information, aggregate properties, i.e., area, size, and distance, are quantified using image processing methods. These properties are used to study the impact of varying supplementary cementitious materials and water-cement ratios on concrete segregation. Lastly, a forensic analysis technique is proposed for comparing and assessing the structural health of different samples within a specific member based on aggregate proportion in the mixture. The proposed method is validated by rigorous statistical analysis and promises broader applications in the real world.
更多查看译文
关键词
Concrete aggregate,Forensic analysis,Deep learning,Semantic segmentation,Structural health monitoring,Concrete inspection
AI 理解论文
溯源树
样例
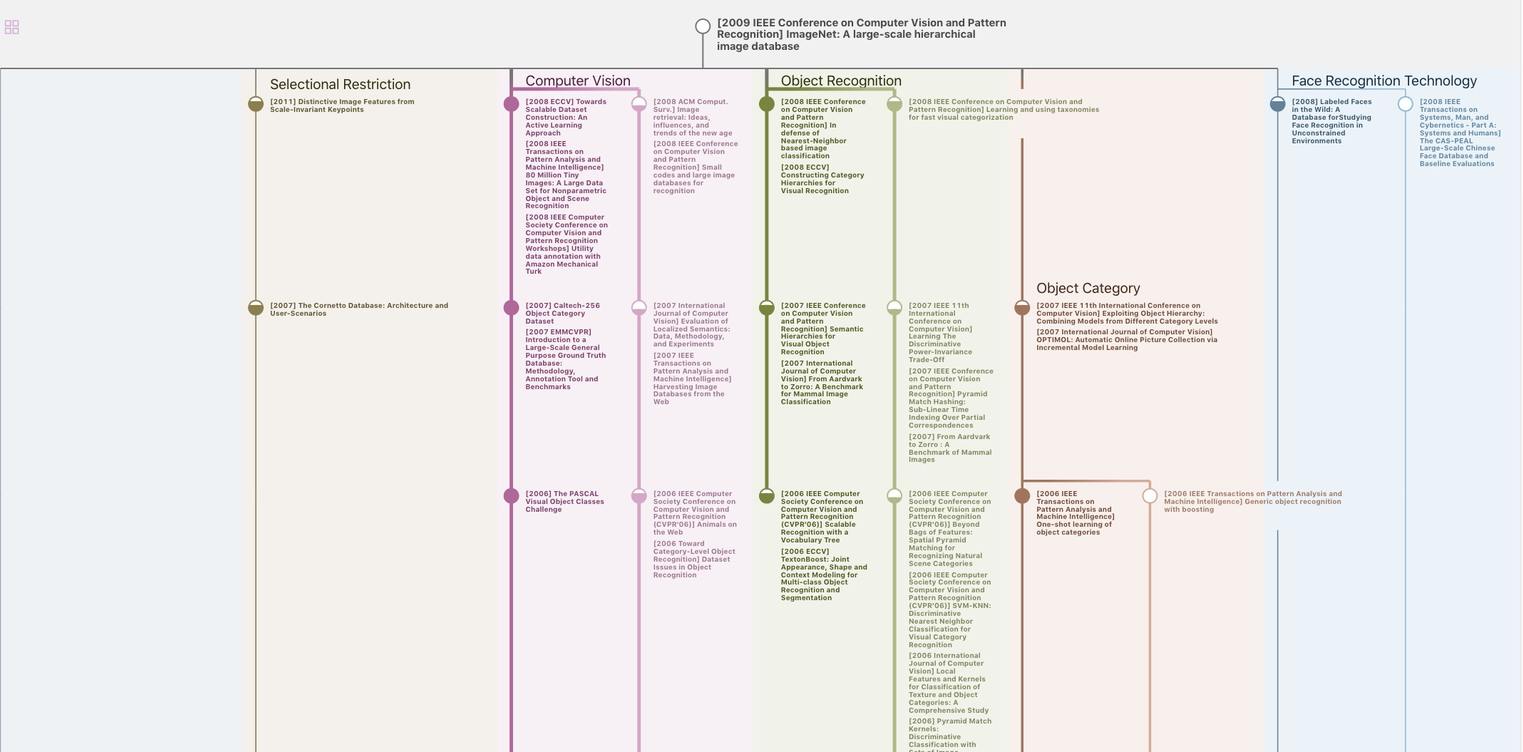
生成溯源树,研究论文发展脉络
Chat Paper
正在生成论文摘要