A fast hyperspectral change detection algorithm for agricultural crops based on low-rank matrix and morphological feature extraction
FRONTIERS IN SUSTAINABLE FOOD SYSTEMS(2024)
摘要
Crop change detection study is the foundation of agricultural sustainability. The inherent high spectral resolution of hyperspectral images, combined with multi-temporal datasets, facilitates the detection of subtle changes. To enhance the accuracy and applicability of hyperspectral change detection in agricultural scenes, this paper introduces a fast hyperspectral change detection approach for agricultural crops based on low-rank matrix and morphological feature extraction (FLRaMF). The goal is to improve detection precision and computational efficiency of the change detection process. The method initially employs rapid low-rank matrix extraction to separate changing and non-changing pixels in the spectral domain. Subsequently, spatial information is introduced using attribute profiles, restricting spectral anomalies through hyperspectral morphology, which ultimately improves the detection results. This study utilized four hyperspectral change detection datasets in agricultural crop scenarios, optimizing and analyzing parameters. Experimental results and analysis indicate that the FLRaMF method can achieve higher detection accuracy with reduced computation cost in unsupervised, default parameter scenarios when performing agricultural crop change detection tasks.
更多查看译文
关键词
agricultural crops,hyperspectral change detection,morphological feature extraction,low-rank matrix,greedy bilateral smoothing
AI 理解论文
溯源树
样例
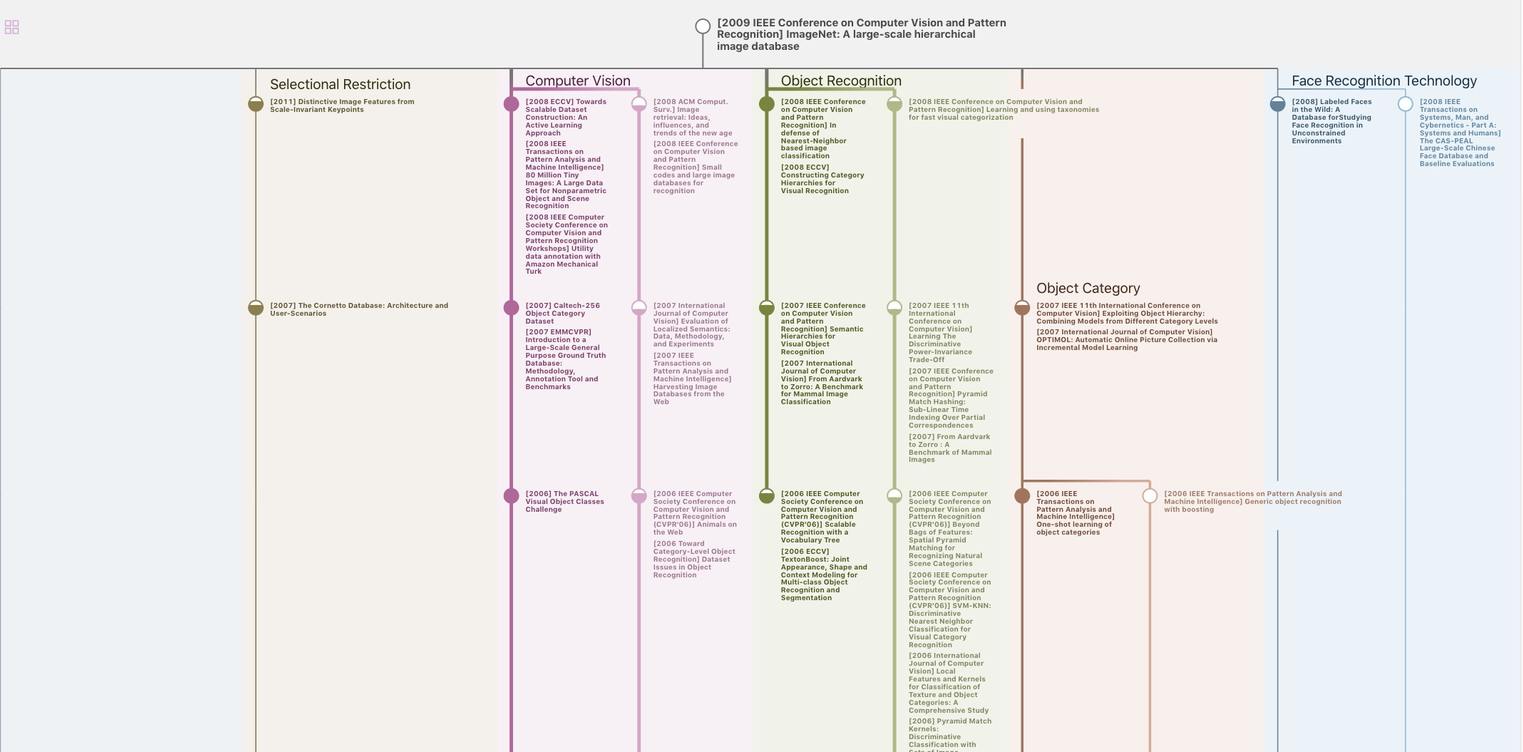
生成溯源树,研究论文发展脉络
Chat Paper
正在生成论文摘要