CTF-former: A novel simplified multi-task learning strategy for simultaneous multivariate chaotic time series prediction
Neural Networks(2024)
摘要
Multivariate chaotic time series prediction is a challenging task, especially when multiple variables are predicted simultaneously. For multiple related prediction tasks typically require multiple models, however, multiple models are difficult to keep synchronization, making immediate communication between predicted values challenging. Although multi-task learning can be applied to this problem, the principles of allocation and layout options between shared and specific representations are ambiguous. To address this issue, a novel simplified multi-task learning method was proposed for the precise implementation of simultaneous multiple chaotic time series prediction tasks. The scheme proposed consists of a cross-convolution operator designed to capture variable correlations and sequence correlations, and an attention module proposed to capture the information embedded in the sequence structure. In the attention module, a non-linear transformation was implemented with convolution, and its local receptive field and the global dependency of the attention mechanism achieve complementarity. In addition, an attention weight calculation was devised that takes into account not only the synergy of time and frequency domain features, but also the fusion of series and channel information. Notably the scheme proposed a purely simplified design principle of multi-task learning by reducing the specific network to single neuron. The precision of the proposed solution and its potential for engineering applications were verified with the Lorenz system and power consumption. The mean absolute error of the proposed method was reduced by an average of 82.9% in the Lorenz system and 19.83% in power consumption compared to the Gated Recurrent Unit.
更多查看译文
关键词
Time series prediction,Chaotic time series,Multivariate prediction,Multi-task learning,Attention mechanism,Power consumption
AI 理解论文
溯源树
样例
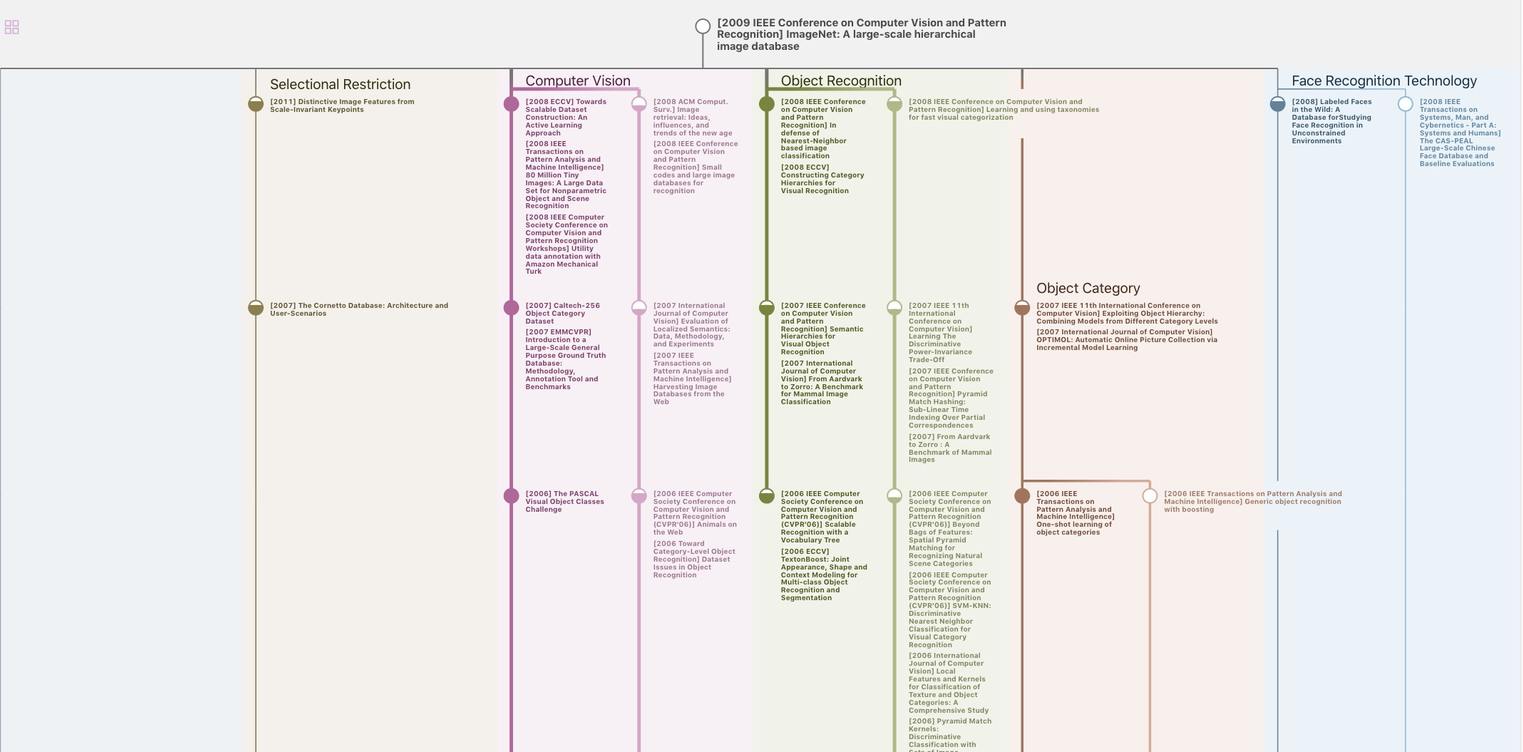
生成溯源树,研究论文发展脉络
Chat Paper
正在生成论文摘要